Deleted articles cannot be recovered. Draft of this article would be also deleted. Are you sure you want to delete this article?
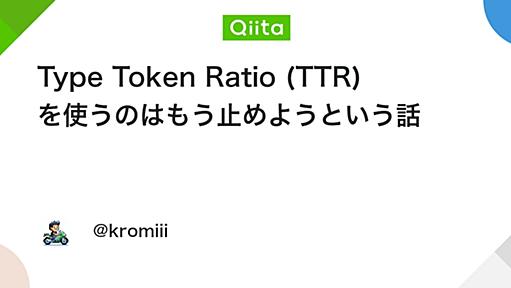
この記事は検証可能な参考文献や出典が全く示されていないか、不十分です。 出典を追加して記事の信頼性向上にご協力ください。(このテンプレートの使い方) 出典検索?: "壺問題" – ニュース · 書籍 · スカラー · CiNii · J-STAGE · NDL · dlib.jp · ジャパンサーチ · TWL (2011年6月) この記事には参考文献や外部リンクの一覧が含まれていますが、脚注による参照が不十分であるため、情報源が依然不明確です。 適切な位置に脚注を追加して、記事の信頼性向上にご協力ください。(2011年6月) 確率論や統計学において、壺問題(つぼもんだい、英語: urn problem)は理想化された思考実験の一つである。実際の関心のある対象(原子、人、車など)を、壺などの容器の中に入れた色付きの球として表現し、壺から1つまたはそれ以上の球を取り出す。思考実験の目的は、あ
アリストテレス以来の文脈では、これは近代以降の論理学の指示、複数性、時制、モダリティと同じ問題を説明するためのものである。この理論を発展させたのは、主に、ジャン・ビュリダン、オッカムのウィリアム、シャーウッドのウィリアム、ウォルター・バーレイ、ペトルス・ヒスパヌスといった哲学者達である。この理論は14世紀までに少なくとも二つの明らかに異なる理論、「拡張(英語版)」を含んでいて、指示の理論により近い「本来的代示」と、意図した目的が不明確な「代示の諸様態」に分かれてしまった。 代示は、項辞(terminus)と項辞を使って話されているところのものとの意味論的な関係である。だから、例えば、「もう一杯飲みなさい」というときの「杯」という項辞は杯に入ったワインを代示している。 論理学的な意味での項辞の「代示物(suppositum)」とは項辞が指示する対象を指す(文法学において「suppositum
Probabilistic latent semantic analysis (PLSA), also known as probabilistic latent semantic indexing (PLSI, especially in information retrieval circles) is a statistical technique for the analysis of two-mode and co-occurrence data. In effect, one can derive a low-dimensional representation of the observed variables in terms of their affinity to certain hidden variables, just as in latent semantic
This article relies excessively on references to primary sources. Please improve this article by adding secondary or tertiary sources. Find sources: "Maximal information coefficient" – news · newspapers · books · scholar · JSTOR (May 2012) (Learn how and when to remove this message) In statistics, the maximal information coefficient (MIC) is a measure of the strength of the linear or non-linear as
The Canberra distance is a numerical measure of the distance between pairs of points in a vector space, introduced in 1966[1] and refined in 1967[2] by Godfrey N. Lance and William T. Williams. It is a weighted version of L₁ (Manhattan) distance.[3] The Canberra distance has been used as a metric for comparing ranked lists[3] and for intrusion detection in computer security.[4] It has also been us
――最初に伝えるべきは、影響を被った「個人株主に対する配慮」だったのではないだろうか? 2024年12月26日、NewsPicksから1本の動画が公開された。 落合陽一氏が率いるピクシーダストテクノロジーズの上場廃止に迫るものであった。 NewsPicksは同氏と懇意にしており、報じないだろうと予見されていた。 その内容は瞬く間に拡散され、当編集部もその取材の緻密さ、ジャーナリズムには身震いさせられた。 【続報】NewsPicks、ついに落合陽一氏の「ピクシーダスト」上場廃止に迫る動画を公開。 正直、震えました。NPレポートシリーズの年度締めに強烈な一本。「ナスダック上場廃止ブーム」関連の2本だけでも、課金して見る価値ありです。 – ■「ピクシーダスト上場廃止」動画のトピックス… https://t.co/WLXKnhFt7R pic.twitter.com/UHnxlS7j99 — SU
The Itakura–Saito distance (or Itakura–Saito divergence) is a measure of the difference between an original spectrum and an approximation of that spectrum. Although it is not a perceptual measure, it is intended to reflect perceptual (dis)similarity. It was proposed by Fumitada Itakura and Shuzo Saito in the 1960s while they were with NTT.[1] The distance is defined as:[2] The Itakura–Saito distan
In computer science, learning vector quantization (LVQ) is a prototype-based supervised classification algorithm. LVQ is the supervised counterpart of vector quantization systems. LVQ can be understood as a special case of an artificial neural network, more precisely, it applies a winner-take-all Hebbian learning-based approach. It is a precursor to self-organizing maps (SOM) and related to neural
ベクトル量子化(ベクトルりょうしか、英: Vector Quantization, VQ)は連続空間に存在するベクトルを有限個の代表ベクトルへ離散化する操作である。すなわちベクトルを入力とする量子化である。 通常の(スカラー)量子化は連続値を有限個の代表値へと集約する。例えば標本化したアナログ音声信号の各サンプルを、最も近いビット/デジタル符号に置き換える。サンプルと代表値はともに1次元/スカラーである。 これに対してベクトル量子化はN次元空間内のベクトルを対象として量子化をおこなう。例えばステレオ2chの信号を各チャンネルごとでなく左右セット (=2次元ベクトル) で扱い、このベクトルをまとめて有限個の代表値へ符号化する。ベクトル単位での量子化であることからベクトル量子化と呼ばれる。
Association rule learning is a rule-based machine learning method for discovering interesting relations between variables in large databases. It is intended to identify strong rules discovered in databases using some measures of interestingness.[1] In any given transaction with a variety of items, association rules are meant to discover the rules that determine how or why certain items are connect
Explosion builds developer tools for AI, Machine Learning and Natural Language Processing. → Consulting ProjectspaCyProdigyThincConsultingCase StudyTopicsLLMsNLP StrategyAnnotationBiomedicalFinanceMediaLegalHumanitiesComputer VisionCategoryBlogReleaseUniverseTalkInterviewVideoPaperTasksAuthorsNewsletterJoin our mailing list to receive updates about new blog posts and projects!
Get things done spaCy is designed to help you do real work — to build real products, or gather real insights. The library respects your time, and tries to avoid wasting it. It's easy to install, and its API is simple and productive. Blazing fast spaCy excels at large-scale information extraction tasks. It's written from the ground up in carefully memory-managed Cython. If your application needs to
リリース、障害情報などのサービスのお知らせ
最新の人気エントリーの配信
処理を実行中です
j次のブックマーク
k前のブックマーク
lあとで読む
eコメント一覧を開く
oページを開く