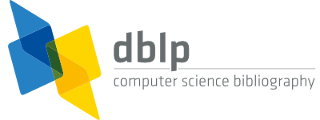


default search action
Advances in Data Analysis and Classification, Volume 10
Volume 10, Number 1, March 2016
- Editorial for issue 1/2016. 1-3
- Vincent Audigier, François Husson, Julie Josse
:
A principal component method to impute missing values for mixed data. 5-26 - Che Ngufor, Janusz Wojtusiak:
Extreme logistic regression. 27-52 - Michela Gnaldi, Silvia Bacci
, Francesco Bartolucci
:
A multilevel finite mixture item response model to cluster examinees and schools. 53-70 - Matthijs J. Warrens:
A comparison of reliability coefficients for psychometric tests that consist of two parts. 71-84 - Mingkun Chen, Evelyne Vigneau:
Supervised clustering of variables. 85-101 - Guillermo Vinué
, Amelia Simó
, Sandra Alemany
:
The k-means algorithm for 3D shapes with an application to apparel design. 103-132
Volume 10, Number 2, June 2016
- Angela Montanari, Maurizio Vichi:
Special issue on Advances in latent variables: methods, models and applications. 133-137 - Margot Bennink, Marcel A. Croon, Brigitte Kroon, Jeroen K. Vermunt
:
Micro-macro multilevel latent class models with multiple discrete individual-level variables. 139-154 - Damien McParland, Isobel Claire Gormley
:
Model based clustering for mixed data: clustMD. 155-169 - Daniel L. Oberski
:
Beyond the number of classes: separating substantive from non-substantive dependence in latent class analysis. 171-182 - Matthieu Marbac, Christophe Biernacki
, Vincent Vandewalle:
Latent class model with conditional dependency per modes to cluster categorical data. 183-207 - Fetene B. Tekle
, Dereje W. Gudicha, Jeroen K. Vermunt
:
Power analysis for the bootstrap likelihood ratio test for the number of classes in latent class models. 209-224 - Anna Gottard, Maria Iannario
, Domenico Piccolo:
Varying uncertainty in CUB models. 225-244 - Francesco Bartolucci
, Giorgio Eduardo Montanari
, Silvia Pandolfi:
Item selection by latent class-based methods: an application to nursing home evaluation. 245-262 - Francesca Bassi
:
Dynamic segmentation with growth mixture models. 263-279
Volume 10, Number 3, September 2016
- Editorial ADAC issue 3, volume 10 (2016). 281-283
- Vincenzo Spinelli:
Pruning boxes in a box-based classification method. 285-304 - Henrik J. Nyman, Jie Xiong, Johan Pensar
, Jukka Corander:
Marginal and simultaneous predictive classification using stratified graphical models. 305-326 - Volodymyr Melnykov, Igor Melnykov
, Semhar Michael
:
Semi-supervised model-based clustering with positive and negative constraints. 327-349 - Tiziano Bellini:
The forward search interactive outlier detection in cointegrated VAR analysis. 351-373 - Hussein Hashem
, Veronica Vinciotti
, Rahim Alhamzawi
, Keming Yu
:
Quantile regression with group lasso for classification. 375-390 - Borja R. Lafuente-Rego
, José Antonio Vilar:
Clustering of time series using quantile autocovariances. 391-415
Volume 10, Number 4, December 2016
- Angela Montanari, Maurizio Vichi:
Second special issue on "Advances in latent variables: methods, models and applications". 417-421 - Cristina Tortora
, Paul D. McNicholas
, Ryan P. Browne
:
A mixture of generalized hyperbolic factor analyzers. 423-440 - Cristina Tortora
, Mireille Gettler-Summa, Marina Marino, Francesco Palumbo
:
Factor probabilistic distance clustering (FPDC): a new clustering method. 441-464 - Pietro Giorgio Lovaglio
, Gianmarco Vacca, Stefano Verzillo
:
Human capital estimation in higher education. 465-489 - Cristina Davino, Vincenzo Esposito Vinzi:
Quantile composite-based path modeling. 491-520 - Arthur J. White
, Thomas Brendan Murphy:
Exponential family mixed membership models for soft clustering of multivariate data. 521-540 - Reyhaneh Rikhtehgaran, Iraj Kazemi:
The determination of uncertainty levels in robust clustering of subjects with longitudinal observations using the Dirichlet process mixture. 541-562 - Semhar Michael
, Volodymyr Melnykov:
An effective strategy for initializing the EM algorithm in finite mixture models. 563-583

manage site settings
To protect your privacy, all features that rely on external API calls from your browser are turned off by default. You need to opt-in for them to become active. All settings here will be stored as cookies with your web browser. For more information see our F.A.Q.