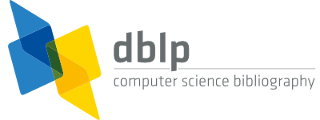


default search action
Kai Ming Ting
Person information
- affiliation: Nanjing University, National Key Laboratory for Novel Software Technology, Nanjing, China
Refine list

refinements active!
zoomed in on ?? of ?? records
view refined list in
export refined list as
2020 – today
- 2025
- [j57]Yang Cao, Yixiao Ma, Ye Zhu, Kai Ming Ting:
Revisiting streaming anomaly detection: benchmark and evaluation. Artif. Intell. Rev. 58(1): 8 (2025) - 2024
- [j56]Kai Ming Ting, Takashi Washio, Ye Zhu
, Yang Xu, Kaifeng Zhang:
Is it possible to find the single nearest neighbor of a query in high dimensions? Artif. Intell. 336: 104206 (2024) - [j55]Yang Cao
, Ye Zhu, Kai Ming Ting, Flora D. Salim, Hong Xian Li, Luxing Yang, Gang Li:
Detecting Change Intervals with Isolation Distributional Kernel. J. Artif. Intell. Res. 79: 273-306 (2024) - [j54]Yufan Wang, Zijing Wang, Kai Ming Ting, Yuanyi Shang:
A Principled Distributional Approach to Trajectory Similarity Measurement and its Application to Anomaly Detection. J. Artif. Intell. Res. 79: 865-893 (2024) - [j53]Kai Ming Ting
, Zongyou Liu, Lei Gong
, Hang Zhang, Ye Zhu
:
A new distributional treatment for time series anomaly detection. VLDB J. 33(3): 753-780 (2024) - [c80]Yang Cao, Ye Zhu, Kai Ming Ting, Flora D. Salim, Hong Xian Li, Luxing Yang, Gang Li:
Detecting Change Intervalswith Isolation Distributional Kernel (Abstract Reprint). IJCAI 2024: 8476 - [c79]Lei Gong, Hang Zhang, Zongyou Liu, Kai Ming Ting, Yang Cao, Ye Zhu:
Local Subsequence-Based Distribution for Time Series Clustering. PAKDD (1) 2024: 259-270 - [c78]Yuanyi Shang, Kai Ming Ting, Zijing Wang, Yufan Wang:
Distributional Kernel: An Effective and Efficient Means for Trajectory Retrieval. PAKDD (5) 2024: 271-283 - [i22]Yang Cao, Haolong Xiang, Hang Zhang, Ye Zhu, Kai Ming Ting:
Anomaly Detection Based on Isolation Mechanisms: A Survey. CoRR abs/2403.10802 (2024) - [i21]Hang Zhang, Yang Xu, Lei Gong, Ye Zhu, Kai Ming Ting:
Distributed Clustering based on Distributional Kernel. CoRR abs/2409.09418 (2024) - [i20]Kaichen Zhou, Yang Cao, Taewhan Kim, Hao Zhao, Hao Dong, Kai Ming Ting, Ye Zhu:
RAD: A Dataset and Benchmark for Real-Life Anomaly Detection with Robotic Observations. CoRR abs/2410.00713 (2024) - [i19]Xinpeng Li, Zile Jiang, Kai Ming Ting, Ye Zhu:
An Online Automatic Modulation Classification Scheme Based on Isolation Distributional Kernel. CoRR abs/2410.02750 (2024) - 2023
- [j52]Ye Zhu
, Kai Ming Ting:
Kernel-based clustering via Isolation Distributional Kernel. Inf. Syst. 117: 102212 (2023) - [j51]Kai Ming Ting, Takashi Washio, Jonathan R. Wells, Hang Zhang, Ye Zhu
:
Isolation Kernel Estimators. Knowl. Inf. Syst. 65(2): 759-787 (2023) - [j50]Xin Han, Ye Zhu
, Kai Ming Ting, Gang Li:
The impact of isolation kernel on agglomerative hierarchical clustering algorithms. Pattern Recognit. 139: 109517 (2023) - [j49]Kai Ming Ting, Bi-Cun Xu
, Takashi Washio
, Zhi-Hua Zhou:
Isolation Distributional Kernel: A New Tool for Point and Group Anomaly Detections. IEEE Trans. Knowl. Data Eng. 35(3): 2697-2710 (2023) - [j48]Kai Ming Ting
, Jonathan R. Wells
, Ye Zhu
:
Point-Set Kernel Clustering. IEEE Trans. Knowl. Data Eng. 35(5): 5147-5158 (2023) - [c77]Zijing Wang, Ye Zhu, Kai Ming Ting:
Distribution-Based Trajectory Clustering. ICDM 2023: 1379-1384 - [c76]Hang Zhang, Kaifeng Zhang, Kai Ming Ting, Ye Zhu:
Towards a Persistence Diagram that is Robust to Noise and Varied Densities. ICML 2023: 41952-41972 - [c75]Zhong Zhuang, Kai Ming Ting, Guansong Pang, Shuaibin Song:
Subgraph Centralization: A Necessary Step for Graph Anomaly Detection. SDM 2023: 703-711 - [i18]Yufan Wang, Kai Ming Ting, Yuanyi Shang:
A principled distributional approach to trajectory similarity measurement. CoRR abs/2301.00393 (2023) - [i17]Zhong Zhuang, Kai Ming Ting, Guansong Pang
, Shuaibin Song:
Subgraph Centralization: A Necessary Step for Graph Anomaly Detection. CoRR abs/2301.06794 (2023) - [i16]Zijing Wang, Ye Zhu, Kai Ming Ting:
Distribution-Based Trajectory Clustering. CoRR abs/2310.05123 (2023) - 2022
- [j47]Ye Zhu
, Kai Ming Ting, Yuan Jin, Maia Angelova
:
Hierarchical clustering that takes advantage of both density-peak and density-connectivity. Inf. Syst. 103: 101871 (2022) - [j46]Kai Ming Ting, Zongyou Liu, Hang Zhang, Ye Zhu:
A New Distributional Treatment for Time Series and An Anomaly Detection Investigation. Proc. VLDB Endow. 15(11): 2321-2333 (2022) - [j45]Xiangyu Song
, Sunil Aryal
, Kai Ming Ting, Zhen Liu
, Bin He:
Spectral-Spatial Anomaly Detection of Hyperspectral Data Based on Improved Isolation Forest. IEEE Trans. Geosci. Remote. Sens. 60: 1-16 (2022) - [j44]Ming Pang
, Kai Ming Ting, Peng Zhao
, Zhi-Hua Zhou
:
Improving Deep Forest by Screening. IEEE Trans. Knowl. Data Eng. 34(9): 4298-4312 (2022) - [c74]Ye Zhu, Kai Ming Ting:
Improving the Effectiveness and Efficiency of Stochastic Neighbour Embedding with Isolation Kernel (Extended Abstract). IJCAI 2022: 5792-5796 - [c73]Xin Han
, Ye Zhu, Kai Ming Ting, De-Chuan Zhan, Gang Li:
Streaming Hierarchical Clustering Based on Point-Set Kernel. KDD 2022: 525-533 - [i15]Yang Cao, Ye Zhu, Kai Ming Ting, Flora D. Salim, Hong Xian Li
, Gang Li:
Detecting Change Intervals with Isolation Distributional Kernel. CoRR abs/2212.14630 (2022) - 2021
- [j43]Kai Ming Ting
, Jonathan R. Wells, Takashi Washio:
Isolation kernel: the X factor in efficient and effective large scale online kernel learning. Data Min. Knowl. Discov. 35(6): 2282-2312 (2021) - [j42]Ye Zhu, Kai Ming Ting:
Improving the Effectiveness and Efficiency of Stochastic Neighbour Embedding with Isolation Kernel. J. Artif. Intell. Res. 71: 667-695 (2021) - [j41]Ye Zhu
, Kai Ming Ting, Mark J. Carman
, Maia Angelova
:
CDF Transform-and-Shift: An effective way to deal with datasets of inhomogeneous cluster densities. Pattern Recognit. 117: 107977 (2021) - [c72]Bi-Cun Xu, Kai Ming Ting, Yuan Jiang:
Isolation Graph Kernel. AAAI 2021: 10487-10495 - [c71]Kai Ming Ting, Takashi Washio, Jonathan R. Wells, Hang Zhang:
Isolation Kernel Density Estimation. ICDM 2021: 619-628 - [c70]Yi-Xuan Xu, Ming Pang, Ji Feng, Kai Ming Ting, Yuan Jiang, Zhi-Hua Zhou:
Reconstruction-based Anomaly Detection with Completely Random Forest. SDM 2021: 127-135 - [i14]Kai Ming Ting, Takashi Washio, Ye Zhu, Yang Xu:
Breaking the curse of dimensionality with Isolation Kernel. CoRR abs/2109.14198 (2021) - 2020
- [j40]Sunil Aryal
, Kai Ming Ting, Takashi Washio, Gholamreza Haffari:
A comparative study of data-dependent approaches without learning in measuring similarities of data objects. Data Min. Knowl. Discov. 34(1): 124-162 (2020) - [j39]Jonathan R. Wells, Sunil Aryal, Kai Ming Ting:
Simple supervised dissimilarity measure: Bolstering iForest-induced similarity with class information without learning. Knowl. Inf. Syst. 62(8): 3203-3216 (2020) - [c69]Kai Ming Ting, Bi-Cun Xu, Takashi Washio, Zhi-Hua Zhou:
Isolation Distributional Kernel: A New Tool for Kernel based Anomaly Detection. KDD 2020: 198-206 - [c68]Bo Chen
, Kai Ming Ting, Tat-Jun Chin:
Anomaly Detection via Neighbourhood Contrast. PAKDD (2) 2020: 647-659 - [c67]Durgesh Samariya
, Sunil Aryal, Kai Ming Ting, Jiangang Ma:
A New Effective and Efficient Measure for Outlying Aspect Mining. WISE (2) 2020: 463-474 - [i13]Kai Ming Ting, Jonathan R. Wells, Ye Zhu:
Clustering based on Point-Set Kernel. CoRR abs/2002.05815 (2020) - [i12]Kai Ming Ting, Bi-Cun Xu, Takashi Washio, Zhi-Hua Zhou:
Isolation Distributional Kernel: A New Tool for Point & Group Anomaly Detection. CoRR abs/2009.12196 (2020) - [i11]Xin Han, Ye Zhu, Kai Ming Ting, Gang Li:
The Impact of Isolation Kernel on Agglomerative Hierarchical Clustering Algorithms. CoRR abs/2010.05473 (2020)
2010 – 2019
- 2019
- [j38]Kai Ming Ting, Ye Zhu
, Mark J. Carman
, Yue Zhu, Takashi Washio, Zhi-Hua Zhou:
Lowest probability mass neighbour algorithms: relaxing the metric constraint in distance-based neighbourhood algorithms. Mach. Learn. 108(2): 331-376 (2019) - [j37]Jonathan R. Wells, Kai Ming Ting:
A new simple and efficient density estimator that enables fast systematic search. Pattern Recognit. Lett. 122: 92-98 (2019) - [c66]Xiaoyu Qin, Kai Ming Ting, Ye Zhu, Vincent C. S. Lee:
Nearest-Neighbour-Induced Isolation Similarity and Its Impact on Density-Based Clustering. AAAI 2019: 4755-4762 - [c65]Xin-Qiang Cai, Peng Zhao, Kai-Ming Ting, Xin Mu, Yuan Jiang:
Nearest Neighbor Ensembles: An Effective Method for Difficult Problems in Streaming Classification with Emerging New Classes. ICDM 2019: 970-975 - [c64]Bi-Cun Xu, Kai Ming Ting, Zhi-Hua Zhou:
Isolation Set-Kernel and Its Application to Multi-Instance Learning. KDD 2019: 941-949 - [i10]Sunil Aryal, Kai Ming Ting, Takashi Washio, Gholamreza Haffari:
A new simple and effective measure for bag-of-word inter-document similarity measurement. CoRR abs/1902.03402 (2019) - [i9]Ye Zhu, Kai Ming Ting:
Improving Stochastic Neighbour Embedding fundamentally with a well-defined data-dependent kernel. CoRR abs/1906.09744 (2019) - [i8]Xiaoyu Qin, Kai Ming Ting, Ye Zhu, Vincent C. S. Lee:
Nearest-Neighbour-Induced Isolation Similarity and its Impact on Density-Based Clustering. CoRR abs/1907.00378 (2019) - [i7]Kai Ming Ting, Jonathan R. Wells, Takashi Washio:
Isolation Kernel: The X Factor in Efficient and Effective Large Scale Online Kernel Learning. CoRR abs/1907.01104 (2019) - 2018
- [j36]Tharindu R. Bandaragoda
, Kai Ming Ting, David W. Albrecht
, Fei Tony Liu, Ye Zhu
, Jonathan R. Wells:
Isolation-based anomaly detection using nearest-neighbor ensembles. Comput. Intell. 34(4): 968-998 (2018) - [j35]Bo Chen
, Kai Ming Ting, Takashi Washio, Ye Zhu
:
Local contrast as an effective means to robust clustering against varying densities. Mach. Learn. 107(8-10): 1621-1645 (2018) - [j34]Ye Zhu
, Kai Ming Ting, Mark J. Carman
:
Grouping points by shared subspaces for effective subspace clustering. Pattern Recognit. 83: 230-244 (2018) - [j33]Yue Zhu
, Kai Ming Ting, Zhi-Hua Zhou
:
Multi-Label Learning with Emerging New Labels. IEEE Trans. Knowl. Data Eng. 30(10): 1901-1914 (2018) - [c63]Kai Ming Ting, Sunil Aryal
, Takashi Washio:
Which Outlier Detector Should I use? ICDM 2018: 8 - [c62]Ming Pang, Kai-Ming Ting, Peng Zhao, Zhi-Hua Zhou:
Improving Deep Forest by Confidence Screening. ICDM 2018: 1194-1199 - [c61]Kai Ming Ting, Yue Zhu, Zhi-Hua Zhou:
Isolation Kernel and Its Effect on SVM. KDD 2018: 2329-2337 - [c60]Ye Zhu
, Kai Ming Ting, Maia Angelova
:
A Distance Scaling Method to Improve Density-Based Clustering. PAKDD (3) 2018: 389-400 - [c59]Bo Chen
, Kai Ming Ting:
Neighbourhood Contrast: A Better Means to Detect Clusters Than Density. PAKDD (3) 2018: 401-412 - [i6]Ye Zhu, Kai Ming Ting, Mark J. Carman, Maia Angelova
:
CDF Transform-Shift: An effective way to deal with inhomogeneous density datasets. CoRR abs/1810.02897 (2018) - [i5]Ye Zhu, Kai Ming Ting, Yuan Jin, Maia Angelova
:
Hierarchical clustering that takes advantage of both density-peak and density-connectivity. CoRR abs/1810.03393 (2018) - [i4]Kai Ming Ting, Takashi Washio, Ata Kabán:
Data Dependent Dissimilarity Measures (NII Shonan Meeting 2018-13). NII Shonan Meet. Rep. 2018 (2018) - 2017
- [j32]Sunil Aryal
, Kai Ming Ting, Takashi Washio, Gholamreza Haffari:
Data-dependent dissimilarity measure: an effective alternative to geometric distance measures. Knowl. Inf. Syst. 53(2): 479-506 (2017) - [j31]Kai Ming Ting, Takashi Washio, Jonathan R. Wells, Sunil Aryal
:
Defying the gravity of learning curve: a characteristic of nearest neighbour anomaly detectors. Mach. Learn. 106(1): 55-91 (2017) - [j30]Xin Mu, Kai Ming Ting, Zhi-Hua Zhou:
Classification Under Streaming Emerging New Classes: A Solution Using Completely-Random Trees. IEEE Trans. Knowl. Data Eng. 29(8): 1605-1618 (2017) - [c58]Yue Zhu, Kai Ming Ting, Zhi-Hua Zhou:
Discover Multiple Novel Labels in Multi-Instance Multi-Label Learning. AAAI 2017: 2977-2984 - [c57]Yue Zhu, Kai Ming Ting, Zhi-Hua Zhou:
New Class Adaptation Via Instance Generation in One-Pass Class Incremental Learning. ICDM 2017: 1207-1212 - [r10]Kai Ming Ting:
Confusion Matrix. Encyclopedia of Machine Learning and Data Mining 2017: 260 - [r9]Kai Ming Ting:
Error Rate. Encyclopedia of Machine Learning and Data Mining 2017: 414 - [r8]Kai Ming Ting:
Precision. Encyclopedia of Machine Learning and Data Mining 2017: 990 - [r7]Kai Ming Ting:
Precision and Recall. Encyclopedia of Machine Learning and Data Mining 2017: 990-991 - [r6]Kai Ming Ting:
Sensitivity and Specificity. Encyclopedia of Machine Learning and Data Mining 2017: 1152 - [i3]Jonathan R. Wells, Kai Ming Ting:
A simple efficient density estimator that enables fast systematic search. CoRR abs/1707.00783 (2017) - 2016
- [j29]Sunil Aryal
, Kai Ming Ting:
A Generic Ensemble Approach to Estimate Multidimensional Likelihood in Bayesian Classifier Learning. Comput. Intell. 32(3): 458-479 (2016) - [j28]Guansong Pang, Kai Ming Ting, David W. Albrecht, Huidong Jin:
ZERO++: Harnessing the Power of Zero Appearances to Detect Anomalies in Large-Scale Data Sets. J. Artif. Intell. Res. 57: 593-620 (2016) - [j27]Ye Zhu
, Kai Ming Ting:
Commentary: a decomposition of the outlier detection problem into a set of supervised learning problems. Mach. Learn. 105(2): 301-304 (2016) - [j26]Ye Zhu
, Kai Ming Ting, Mark James Carman
:
Density-ratio based clustering for discovering clusters with varying densities. Pattern Recognit. 60: 983-997 (2016) - [c56]Yue Zhu, Kai Ming Ting
, Zhi-Hua Zhou:
Multi-label Learning with Emerging New Labels. ICDM 2016: 1371-1376 - [c55]Kai Ming Ting, Ye Zhu
, Mark James Carman
, Yue Zhu, Zhi-Hua Zhou:
Overcoming Key Weaknesses of Distance-based Neighbourhood Methods using a Data Dependent Dissimilarity Measure. KDD 2016: 1205-1214 - [c54]Sunil Aryal
, Kai Ming Ting, Gholamreza Haffari:
Revisiting Attribute Independence Assumption in Probabilistic Unsupervised Anomaly Detection. PAISI 2016: 73-86 - [i2]Xin Mu, Kai Ming Ting, Zhi-Hua Zhou:
Classification under Streaming Emerging New Classes: A Solution using Completely Random Trees. CoRR abs/1605.09131 (2016) - 2015
- [j25]Bo Chen
, Kai Ming Ting, Takashi Washio, Gholamreza Haffari:
Half-space mass: a maximally robust and efficient data depth method. Mach. Learn. 100(2-3): 677-699 (2015) - [c53]Sunil Aryal
, Kai Ming Ting, Gholamreza Haffari, Takashi Washio:
Beyond tf-idf and Cosine Distance in Documents Dissimilarity Measure. AIRS 2015: 400-406 - [c52]Guansong Pang
, Kai Ming Ting, David W. Albrecht
:
LeSiNN: Detecting Anomalies by Identifying Least Similar Nearest Neighbours. ICDM Workshops 2015: 623-630 - 2014
- [j24]Jonathan R. Wells, Kai Ming Ting, Takashi Washio:
LiNearN: A new approach to nearest neighbour density estimator. Pattern Recognit. 47(8): 2702-2720 (2014) - [c51]Tharindu R. Bandaragoda
, Kai Ming Ting, David W. Albrecht
, Fei Tony Liu, Jonathan R. Wells:
Efficient Anomaly Detection by Isolation Using Nearest Neighbour Ensemble. ICDM Workshops 2014: 698-705 - [c50]Sunil Aryal
, Kai Ming Ting, Gholamreza Haffari, Takashi Washio:
Mp-Dissimilarity: A Data Dependent Dissimilarity Measure. ICDM 2014: 707-712 - [c49]Sunil Aryal
, Kai Ming Ting, Jonathan R. Wells, Takashi Washio:
Improving iForest with Relative Mass. PAKDD (2) 2014: 510-521 - 2013
- [j23]Kai Ming Ting, Lian Zhu, Jonathan R. Wells:
Local Models - the Key to Boosting Stable Learners Successfully. Comput. Intell. 29(2): 331-356 (2013) - [j22]Kai Ming Ting, Takashi Washio, Jonathan R. Wells, Fei Tony Liu, Sunil Aryal
:
DEMass: a new density estimator for big data. Knowl. Inf. Syst. 35(3): 493-524 (2013) - [j21]Kai Ming Ting, Guang-Tong Zhou, Fei Tony Liu, Swee Chuan Tan:
Mass estimation. Mach. Learn. 90(1): 127-160 (2013) - [j20]Zhouyu Fu, Guojun Lu
, Kai Ming Ting, Dengsheng Zhang
:
Efficient nonlinear classification via low-rank regularised least squares. Neural Comput. Appl. 22(7-8): 1279-1289 (2013) - [j19]Zhouyu Fu, Guojun Lu
, Kai Ming Ting, Dengsheng Zhang
:
Learning Sparse Kernel Classifiers for Multi-Instance Classification. IEEE Trans. Neural Networks Learn. Syst. 24(9): 1377-1389 (2013) - [c48]Zhouyu Fu, Guojun Lu, Kai Ming Ting, Dengsheng Zhang:
Optimizing Cepstral Features for Audio Classification. IJCAI 2013: 1330-1336 - [c47]Sunil Aryal
, Kai Ming Ting:
MassBayes: A New Generative Classifier with Multi-dimensional Likelihood Estimation. PAKDD (1) 2013: 136-148 - 2012
- [j18]Geoffrey I. Webb
, Janice R. Boughton, Fei Zheng, Kai Ming Ting, Houssam Salem:
Learning by extrapolation from marginal to full-multivariate probability distributions: decreasingly naive Bayesian classification. Mach. Learn. 86(2): 233-272 (2012) - [j17]Guang-Tong Zhou, Kai Ming Ting, Fei Tony Liu, Yilong Yin:
Relevance feature mapping for content-based multimedia information retrieval. Pattern Recognit. 45(4): 1707-1720 (2012) - [j16]Fei Tony Liu, Kai Ming Ting, Zhi-Hua Zhou:
Isolation-Based Anomaly Detection. ACM Trans. Knowl. Discov. Data 6(1): 3:1-3:39 (2012) - [c46]Jonathan R. Wells, Kai Ming Ting, Naiwala P. Chandrasiri:
A non-time series approach to vehicle related time series problems. AusDM 2012: 61-70 - [c45]Zhouyu Fu, Guojun Lu
, Kai Ming Ting, Dengsheng Zhang
:
Learning Sparse Kernel Classifiers in the Primal. SSPR/SPR 2012: 60-69 - 2011
- [j15]Kai Ming Ting, Jonathan R. Wells, Swee Chuan Tan, Shyh Wei Teng
, Geoffrey I. Webb
:
Feature-subspace aggregating: ensembles for stable and unstable learners. Mach. Learn. 82(3): 375-397 (2011) - [j14]Swee Chuan Tan, Kai Ming Ting, Shyh Wei Teng
:
A general stochastic clustering method for automatic cluster discovery. Pattern Recognit. 44(10-11): 2786-2799 (2011) - [j13]Zhouyu Fu, Guojun Lu
, Kai Ming Ting, Dengsheng Zhang
:
Music classification via the bag-of-features approach. Pattern Recognit. Lett. 32(14): 1768-1777 (2011) - [j12]Zhouyu Fu, Guojun Lu
, Kai Ming Ting, Dengsheng Zhang
:
A Survey of Audio-Based Music Classification and Annotation. IEEE Trans. Multim. 13(2): 303-319 (2011) - [c44]Kai Ming Ting, Takashi Washio, Jonathan R. Wells, Fei Tony Liu:
Density Estimation Based on Mass. ICDM 2011: 715-724 - [c43]Zhouyu Fu, Guojun Lu
, Kai Ming Ting, Dengsheng Zhang
:
On Low-Rank Regularized Least Squares for Scalable Nonlinear Classification. ICONIP (2) 2011: 490-499 - [c42]Swee Chuan Tan, Kai Ming Ting, Fei Tony Liu:
Fast Anomaly Detection for Streaming Data. IJCAI 2011: 1511-1516 - [c41]Zhouyu Fu, Guojun Lu
, Kai Ming Ting, Dengsheng Zhang
:
Building Sparse Support Vector Machines for Multi-Instance Classification. ECML/PKDD (1) 2011: 471-486 - [c40]Swee Chuan Tan, Kai Ming Ting, Shyh Wei Teng
:
Simplifying and improving ant-based clustering. ICCS 2011: 46-55 - [i1]Kai Ming Ting, Ian H. Witten:
Issues in Stacked Generalization. CoRR abs/1105.5466 (2011) - 2010
- [j11]Takashi Washio, Einoshin Suzuki, Kai Ming Ting:
Best papers from the 12th Pacific-Asia conference on knowledge discovery and data mining (PAKDD2008). Knowl. Inf. Syst. 25(2): 209-210 (2010) - [c39]Swee Chuan Tan, Kai Ming Ting, Shyh Wei Teng
:
A Comparative Study of a Practical Stochastic Clustering Method with Traditional Methods. Australasian Conference on Artificial Intelligence 2010: 112-121 - [c38]Kai Ming Ting, Jonathan R. Wells:
Multi-dimensional Mass Estimation and Mass-based Clustering. ICDM 2010: 511-520 - [c37]Zhouyu Fu, Guojun Lu
, Kai Ming Ting, Dengsheng Zhang
:
Learning Naive Bayes Classifiers for Music Classification and Retrieval. ICPR 2010: 4589-4592 - [c36]Kai Ming Ting, Guang-Tong Zhou, Fei Tony Liu, James Swee Chuan Tan:
Mass estimation and its applications. KDD 2010: 989-998 - [c35]Fei Tony Liu, Kai Ming Ting, Zhi-Hua Zhou:
On Detecting Clustered Anomalies Using SCiForest. ECML/PKDD (2) 2010: 274-290 - [c34]Zhouyu Fu, Guojun Lu
, Kai Ming Ting, Dengsheng Zhang
:
On Feature Combination for Music Classification. SSPR/SPR 2010: 453-462 - [r5]Kai Ming Ting:
Confusion Matrix. Encyclopedia of Machine Learning 2010: 209 - [r4]Kai Ming Ting:
Error Rate. Encyclopedia of Machine Learning 2010: 331 - [r3]Kai Ming Ting:
Precision. Encyclopedia of Machine Learning 2010: 780 - [r2]Kai Ming Ting:
Precision and Recall. Encyclopedia of Machine Learning 2010: 781 - [r1]Kai Ming Ting:
Sensitivity and Specificity. Encyclopedia of Machine Learning 2010: 901-902
2000 – 2009
- 2009
- [c33]Kai Ming Ting, Jonathan R. Wells, Swee Chuan Tan, Shyh Wei Teng
, Geoffrey I. Webb
:
FaSS: Ensembles for Stable Learners. MCS 2009: 364-374 - [c32]Kai Ming Ting, Lian Zhu:
Boosting Support Vector Machines Successfully. MCS 2009: 509-518 - 2008
- [j10]Fei Tony Liu, Kai Ming Ting, Yang Yu, Zhi-Hua Zhou:
Spectrum of Variable-Random Trees. J. Artif. Intell. Res. 32: 355-384 (2008) - [c31]Swee Chuan Tan, Kai Ming Ting, Shyh Wei Teng
:
Issues of grid-cluster retrievals in swarm-based clustering. IEEE Congress on Evolutionary Computation 2008: 511-518 - [c30]Fei Tony Liu, Kai Ming Ting, Zhi-Hua Zhou:
Isolation Forest. ICDM 2008: 413-422 - [e1]Takashi Washio, Einoshin Suzuki, Kai Ming Ting, Akihiro Inokuchi:
Advances in Knowledge Discovery and Data Mining, 12th Pacific-Asia Conference, PAKDD 2008, Osaka, Japan, May 20-23, 2008 Proceedings. Lecture Notes in Computer Science 5012, Springer 2008, ISBN 978-3-540-68124-3 [contents] - 2007
- [j9]Ying Yang, Geoffrey I. Webb
, Kevin B. Korb, Kai Ming Ting:
Classifying under computational resource constraints: anytime classification using probabilistic estimators. Mach. Learn. 69(1): 35-53 (2007) - [j8]Ying Yang, Geoffrey I. Webb
, Jesús Cerquides
, Kevin B. Korb, Janice R. Boughton, Kai Ming Ting:
To Select or To Weigh: A Comparative Study of Linear Combination Schemes for SuperParent-One-Dependence Estimators. IEEE Trans. Knowl. Data Eng. 19(12): 1652-1665 (2007) - [c29]Swee Chuan Tan, Kai Ming Ting, Shyh Wei Teng:
Examining Dissimilarity Scaling in Ant Colony Approaches to Data Clustering. ACAL 2007: 269-280 - [c28]Yang Yu, Zhi-Hua Zhou, Kai Ming Ting:
Cocktail Ensemble for Regression. ICDM 2007: 721-726 - 2006
- [c27]Shyh Wei Teng, Kai Ming Ting:
Ehipasiko: A Content-based Image Indexing and Retrieval System. AMT 2006: 436-437 - [c26]Tasadduq Imam, Kai Ming Ting, Joarder Kamruzzaman:
z-SVM: An SVM for Improved Classification of Imbalanced Data. Australian Conference on Artificial Intelligence 2006: 264-273 - [c25]Swee Chuan Tan, Kai Ming Ting, Shyh Wei Teng:
Reproducing the Results of Ant-based Clustering Without Using Ants. IEEE Congress on Evolutionary Computation 2006: 1760-1767 - [c24]Ying Yang, Geoffrey I. Webb
, Jesús Cerquides, Kevin B. Korb, Janice R. Boughton, Kai Ming Ting:
To Select or To Weigh: A Comparative Study of Model Selection and Model Weighing for SPODE Ensembles. ECML 2006: 533-544 - [c23]Fei Tony Liu, Kai Ming Ting:
Variable Randomness in Decision Tree Ensembles. PAKDD 2006: 81-90 - 2005
- [j7]Geoffrey I. Webb
, Kai Ming Ting:
On the Application of ROC Analysis to Predict Classification Performance Under Varying Class Distributions. Mach. Learn. 58(1): 25-32 (2005) - [c22]Ying Yang, Kevin B. Korb, Kai Ming Ting, Geoffrey I. Webb
:
Ensemble Selection for SuperParent-One-Dependence Estimators. Australian Conference on Artificial Intelligence 2005: 102-112 - [c21]Fei Tony Liu, Kai Ming Ting, Wei Fan:
Maximizing Tree Diversity by Building Complete-Random Decision Trees. PAKDD 2005: 605-610 - 2004
- [c20]Kwok Pan Pang, Kai Ming Ting:
Improving the Centered CUSUMS Statistic for Structural Break Detection in Time Series. Australian Conference on Artificial Intelligence 2004: 402-413 - [c19]Kai Ming Ting:
Matching Model Versus Single Model: A Study of the Requirement to Match Class Distribution Using Decision Trees. ECML 2004: 429-440 - 2003
- [j6]Kai Ming Ting, Zijian Zheng:
A Study of AdaBoost with Naive Bayesian Classifiers: Weakness and Improvement. Comput. Intell. 19(2): 186-200 (2003) - [c18]Kai Ming Ting, Regina Jing Ying Quek:
Model Stability: A key factor in determining whether an algorithm produces an optimal model from a matching distribution. ICDM 2003: 653-656 - 2002
- [j5]Kai Ming Ting:
An Instance-Weighting Method to Induce Cost-Sensitive Trees. IEEE Trans. Knowl. Data Eng. 14(3): 659-665 (2002) - [c17]Kai Ming Ting:
A Study on the Effect of Class Distribution Using Cost-Sensitive Learning. Discovery Science 2002: 98-112 - [c16]Kai Ming Ting:
Issues in Classifier Evaluation using Optimal Cost Curves. ICML 2002: 642-649 - 2000
- [c15]Kai Ming Ting:
An Empirical Study of MetaCost Using Boosting Algorithms. ECML 2000: 413-425 - [c14]Kai Ming Ting:
A Comparative Study of Cost-Sensitive Boosting Algorithms. ICML 2000: 983-990
1990 – 1999
- 1999
- [j4]Kai Ming Ting, Ian H. Witten:
Issues in Stacked Generalization. J. Artif. Intell. Res. 10: 271-289 (1999) - [j3]Kai Ming Ting, Boon Toh Low, Ian H. Witten:
Learning from Batched Data: Model Combination Versus Data Combination. Knowl. Inf. Syst. 1(1): 83-106 (1999) - [c13]Zijian Zheng, Geoffrey I. Webb, Kai Ming Ting:
Lazy Bayesian Rules: A Lazy Semi-Naive Bayesian Learning Technique Competitive to Boosting Decision Trees. ICML 1999: 493-502 - [c12]Yakov Frayman, Kai Ming Ting, Lipo Wang:
A fuzzy neural network for data mining: dealing with the problem of small disjuncts. IJCNN 1999: 2490-2493 - [c11]Kai Ming Ting, Zijian Zheng:
Improving the Performance of Boosting for Naive Bayesian Classification. PAKDD 1999: 296-305 - 1998
- [c10]Kai Ming Ting, Zijian Zheng:
Boosting Cost-Sensitive Trees. Discovery Science 1998: 244-255 - [c9]Kai Ming Ting, Zijian Zheng:
Boosting Trees for Cost-Sensitive Classifications. ECML 1998: 190-195 - [c8]Zijian Zheng, Geoffrey I. Webb, Kai Ming Ting:
Integrating boosting and stochastic attribute selection committees for further improving the performance of decision tree learning. ICTAI 1998: 216-223 - [c7]Kai Ming Ting:
Inducing Cost-Sensitive Trees via Instance Weighting. PKDD 1998: 139-147 - 1997
- [j2]Kai Ming Ting:
Discretisation in Lazy Learning Algorithms. Artif. Intell. Rev. 11(1-5): 157-174 (1997) - [j1]Kai Ming Ting:
Decision Combination Based on the Characterisation of Predictive Accuracy. Intell. Data Anal. 1(1-4): 181-205 (1997) - [c6]Kai Ming Ting, Boon Toh Low:
Model Combination in the Multiple-Data-Batches Scenario. ECML 1997: 250-265 - [c5]Kai Ming Ting, Ian H. Witten:
Stacking Bagged and Dagged Models. ICML 1997: 367-375 - [c4]Kai Ming Ting, Ian H. Witten:
Stacked Generalizations: When Does It Work? IJCAI (2) 1997: 866-873 - 1996
- [c3]Kai Ming Ting:
The Characterisation of Predictive Accuracy and Decision Combination. ICML 1996: 498-506 - 1995
- [c2]Kai Ming Ting:
Towards using a Single Uniform Metric in Instance-Based Learning. ICCBR 1995: 559-568 - 1994
- [c1]Kai Ming Ting:
An M-of-N Rule Induction Algorithm and its Application to DNA Domain. HICSS (5) 1994: 133-140
Coauthor Index

manage site settings
To protect your privacy, all features that rely on external API calls from your browser are turned off by default. You need to opt-in for them to become active. All settings here will be stored as cookies with your web browser. For more information see our F.A.Q.
Unpaywalled article links
Add open access links from to the list of external document links (if available).
Privacy notice: By enabling the option above, your browser will contact the API of unpaywall.org to load hyperlinks to open access articles. Although we do not have any reason to believe that your call will be tracked, we do not have any control over how the remote server uses your data. So please proceed with care and consider checking the Unpaywall privacy policy.
Archived links via Wayback Machine
For web page which are no longer available, try to retrieve content from the of the Internet Archive (if available).
Privacy notice: By enabling the option above, your browser will contact the API of archive.org to check for archived content of web pages that are no longer available. Although we do not have any reason to believe that your call will be tracked, we do not have any control over how the remote server uses your data. So please proceed with care and consider checking the Internet Archive privacy policy.
Reference lists
Add a list of references from ,
, and
to record detail pages.
load references from crossref.org and opencitations.net
Privacy notice: By enabling the option above, your browser will contact the APIs of crossref.org, opencitations.net, and semanticscholar.org to load article reference information. Although we do not have any reason to believe that your call will be tracked, we do not have any control over how the remote server uses your data. So please proceed with care and consider checking the Crossref privacy policy and the OpenCitations privacy policy, as well as the AI2 Privacy Policy covering Semantic Scholar.
Citation data
Add a list of citing articles from and
to record detail pages.
load citations from opencitations.net
Privacy notice: By enabling the option above, your browser will contact the API of opencitations.net and semanticscholar.org to load citation information. Although we do not have any reason to believe that your call will be tracked, we do not have any control over how the remote server uses your data. So please proceed with care and consider checking the OpenCitations privacy policy as well as the AI2 Privacy Policy covering Semantic Scholar.
OpenAlex data
Load additional information about publications from .
Privacy notice: By enabling the option above, your browser will contact the API of openalex.org to load additional information. Although we do not have any reason to believe that your call will be tracked, we do not have any control over how the remote server uses your data. So please proceed with care and consider checking the information given by OpenAlex.
last updated on 2024-12-10 20:48 CET by the dblp team
all metadata released as open data under CC0 1.0 license
see also: Terms of Use | Privacy Policy | Imprint