Abstract
Due to the possible lack of primal-dual-type error bounds, it was not clear whether the Karush–Kuhn–Tucker (KKT) residuals of the sequence generated by the augmented Lagrangian method (ALM) for solving convex composite conic programming (CCCP) problems converge superlinearly. In this paper, we resolve this issue by establishing the R-superlinear convergence of the KKT residuals generated by the ALM under only a mild quadratic growth condition on the dual of CCCP, with easy-to-implement stopping criteria for the augmented Lagrangian subproblems. This discovery may help to explain the good numerical performance of several recently developed semismooth Newton-CG based ALM solvers for linear and convex quadratic semidefinite programming.



Similar content being viewed by others
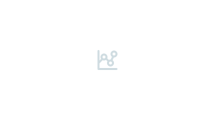
Notes
For the convergence of the PPA, one does not need the parameters to be increasing as long as \(\sigma _k\) is bounded away from zero.
The Lipschitz continuity of a set-valued mapping may refer to other properties elsewhere, such as in [52, Definition 9.26].
For NLP, the constraint nondegeneracy coincides with the linear independence constraint qualification [46].
References
Alizadeh, F., Haeberly, J.-P.A., Overton, M.L.: Complementarity and nondegeneracy in semidefinite programming. Math. Program. 77(2), 111–128 (1997)
Alves, M.M., Svaiter, B.F.: A note on Fejér-monotone sequences in product spaces and its applications to the dual convergence of augmented Lagrangian methods. Math. Program. 155(1), 613–616 (2016)
Artacho, F.J.A., Geoffroy, M.H.: Characterization of metric regularity of subdifferentials. J. Convex Anal. 15(2), 365–380 (2008)
Attouch, H., Soueycatt, M.: Augmented Lagrangian and proximal alternating direction methods of multipliers in Hilbert spaces. Applications to games, PDE’s and control. Pac. J. Optim. 5(1), 17–37 (2009)
Bauschke, H.H., Borwein, J.M., Li, W.: Strong conical hull intersection property, bounded linear regularity, Jameson’s property (G), and error bounds in convex optimization. Math. Program. 86(1), 135–160 (1999)
Bergounioux, M.: Use of augmented Lagrangian methods for the optimal control of obstacle problems. J. Optim. Theory Appl. 95(1), 101–126 (1997)
Bertsekas, D.: Constrained Optimization and Lagrange Multipliers Methods. Academic Press, New York (1982)
Bhansali, V., Wise, B.: Forecasting portfolio risk in normal and stressed market. J. Risk 4(1), 91–106 (2001)
Bonnans, J.F., Shapiro, A.: Perturbation Analysis of Optimization Problems. Springer, New York (2000)
Chen, C.H., Liu, Y.J., Sun, D.F., Toh, K.-C.: A semismooth Newton-CG dual proximal point algorithm for matrix spectral norm approximation problems. Math. Program. 155(1), 435–470 (2016)
Conn, A.R., Gould, N., Sartenaer, A., Toint, P.L.: Convergence properties of an augmented Lagrangian algorithm for optimization with a combination of general equality and linear constraints. SIAM J. Optim. 6(3), 674–703 (1996)
Conn, A.R., Gould, N., Toint, P.L.: A globally convergent augmented Lagrangian algorithm for optimization with general constraints and simple bounds. SIAM J. Numer. Anal. 28(2), 545–572 (1991)
Contesse-Becker, L.: Extended convergence results for the method of multipliers for non-strictly binding inequality constraints. J. Optim. Theory Appl. 79(2), 273–310 (1993)
Cui, Y., Ding, C., Zhao, X.Y.: Quadratic growth conditions for convex matrix optimization problems associated with spectral functions. SIAM J. Optim. 27(4), 2332–2355 (2017)
Cui, Y., Sun, D.F.: A complete characterization on the robust isolated calmness of the nuclear norm regularized convex optimization problems. J. Comput. Math. 36(3), 441–458 (2018)
Ding, C.: An Introduction to a Class of Matrix Optimization Problems. PhD thesis, National University of Singapore (2012)
Ding, C., Sun, D.F., Zhang, L.W.: Characterization of the robust isolated calmness for a class of conic programming problems. SIAM J. Optim. 27(1), 67–90 (2017)
Dontchev, A.L., Rockafellar, R.T.: Characterizations of Lipschitz stability in nonlinear programming. In Mathematical Programming With Data Perturbations, Marcel Dekker, New York, pp. 65–82 (1997)
Dontchev, A.L., Rockafellar, R.T.: Implicit Functions and Solution Mappings. Springer, New York (2009)
Dorsch, D., Gómez, W., Shikhman, V.: Sufficient optimality conditions hold for almost all nonlinear semidefinite programs. Math. Program. 158(1), 77–97 (2016)
Eckstein, J., Silva, P.J.S.: A practical relative error criterion for augmented Lagrangians. Math. Program. 141(1), 319–348 (2013)
Fernández, D., Solodov, M.V.: Local convergence of exact and inexact augmented Lagrangian methods under the second-order sufficient optimality condition. SIAM J. Optim. 22(2), 384–407 (2012)
Fortin, M., Glowinski, R.: Augmented Lagrangian Methods: Applications to Numerical Solutions of Boundary Value Problems. North-Holland, Amsterdam (1983)
Gafni, E.M., Bertsekas, D.P.: Two-metric projection methods for constrained optimization. SIAM J. Control Optim. 22(6), 936–964 (1984)
Golshtein, E.G., Tretyakov, N.V.: Modified Lagrangians and Monotone Maps in Optimization. Wiley, New York (1989)
Han, D.R., Sun, D.F., Zhang, L.W.: Linear rate convergence of the alternating direction method of multipliers for convex composite programming. Math. Oper. Res. 43(2), 622–637 (2018)
Han, D.R., Sun, D.F., Zhang, L.W.: Linear rate convergence of the alternating direction method of multipliers for convex composite quadratic and semi-definite programming. arXiv:1508.02134 (2015)
Hestenes, M.R.: Multiplier and gradient methods. J. Optim. Theory Appl. 4(5), 303–320 (1969)
Higham, N.J.: Computing the nearest correlation matrix—a problem from finance. IMA J. Numer. Anal. 22(3), 329–343 (2002)
Higham, N.J., Strabić, N.: Bounds for the distance to the nearest correlation matrix. SIAM J. Matrix Anal. A 37(3), 1088–1102 (2016)
Ito, K., Kunisch, K.: The augmented Lagrangian method for equality and inequality constraints in Hilbert spaces. Math. Program. 46(1), 341–360 (1990)
Izmailov, A.F., Kurennoy, A.S., Solodov, M.V.: A note on upper Lipschitz stability, error bounds, and critical multipliers for Lipschitz-continuous KKT systems. Math. Program. 142(1), 591–604 (2013)
Jiang, K.F., Sun, D.F., Toh, K.-C.: Solving nuclear norm regularized and semidefinite matrix least squares problems with linear equality constraints. In Discrete Geometry and Optimization. Springer, pp. 133–162 (2013)
Klatte, D.: Upper Lipschitz behavior of solutions to perturbed \(C^{1, 1}\) programs. Math. Program. 88(2), 285–311 (2000)
Leventhal, D.: Metric subregularity and the proximal point method. J. Math. Anal. Appl. 360(2), 681–688 (2009)
Li, X.D., Sun, D.F., Toh, K.-C.: QSDPNAL: a two-phase augmented Lagrangian method for convex quadratic semidefinite programming. Math. Program. Comput. 10, 1–41 (2018). in print
Luque, F.J.: Asymptotic convergence analysis of the proximal point algorithm. SIAM J. Optim. 22(2), 277–293 (1984)
Mordukhovich, B.S., Sarabi, M.E.: Critical multipliers in variational systems via second-order generalized differentiation. Math. Program. 169(2), 605–645 (2018)
Nilssen, T.K., Mannseth, T., Tai, X.-C.: Permeability estimation with the augmented Lagrangian method for a nonlinear diffusion equation. Comput. Geosci. 7(1), 27–47 (2003)
Pataki, G., Tuncȩl, L.: On the generic properties of convex optimization problems in conic form. Math. Program. 89(3), 449–457 (2001)
Pennanen, T.: Local convergence of the proximal point algorithm and multiplier methods without monotonicity. Math. Oper. Res. 27(1), 170–191 (2002)
Powell, M.J.D.: A method for nonlinear constraints in minimization problems. In: Fletcher, R. (ed.) Optimization, pp. 283–298. Academic, New York (1969)
Qi, H.D., Sun, D.F.: A quadratically convergent Newton method for computing the nearest correlation matrix. SIAM J. Matrix Anal. A 28(2), 360–385 (2006)
Robinson, S.M.: An implicit-function theorem for generalized variational inequalties. Technical summary report no. 1672, Mathematics Research Center, University of Wisconsin-Madison, (1976); available from National Technical Information Service under Accession No. ADA031952
Robinson, S.M.: Some continuity properties of polyhedral multifunctions. Math. Program. Study 14, 206–214 (1981)
Robinson, S.M.: Constraint nondegeneracy in variational analysis. Math. Oper. Res. 28(2), 201–232 (2003)
Rockafellar, R.T.: Convex Analysis. Princeton University Press, Princeton (1970)
Rockafellar, R.T.: Local boundedness of nonlinear monotone operators. Mich. Math. J. 16(4), 397–407 (1969)
Rockafellar, R.T.: Conjugate Duality and Optimization. SIAM, Philadelphia (1974)
Rockafellar, R.T.: Augmented Lagrangians and applications of the proximal point algorithm in convex programming. Math. Oper. Res. 1(2), 97–116 (1976)
Rockafellar, R.T.: Monotone operators and the proximal point algorithm. SIAM J. Control Optim. 14(5), 877–898 (1976)
Rockafellar, R.T., Wets, R.J.-B.: Variational Analysis. Springer, New York (1998)
Shapiro, A.: First and second order analysis of nonlinear semidefinite programs. Math. Program. 77(2), 301–320 (1997)
Shapiro, A.: Sensitivity analysis of generalized equations. J. Math. Sci. 115(4), 2554–2565 (2003)
Shapiro, A., Sun, J.: Some properties of the augmented Lagrangian in cone constrained optimization. Math. Oper. Res. 29(3), 479–491 (2004)
Sun, D.F., Sun, J., Zhang, L.W.: The rate of convergence of the augmented Lagrangian method for nonlinear semidefinite programming. Math. Program. 114(2), 349–391 (2008)
Sun, J.: On Monotropic Piecewise Qudratic Programming. PhD thesis, University of Washington, Seattle (1986)
Toint, P.L.: Global convergence of a class of trust-region methods for nonconvex minimization in hilbert space. IMA J. Numer. Anal. 8(2), 231–252 (1988)
Yang, L.Q., Sun, D.F., Toh, K.-C.: SDPNAL+: a majorized semismooth Newton-CG augmented Lagrangian method for semidefinite programming with nonnegative constraints. Math. Program. Comput. 7(3), 1–36 (2015)
Zhao, X.Y., Sun, D.F., Toh, K.-C.: A Newton-CG augmented Lagrangian method for semidefinite programming. SIAM J. Optim. 20(4), 1737–1765 (2010)
Zhou, Z.R., So, A.M.C.: A unified approach to error bounds for structured convex optimization problems. Math. Program. 165(2), 689–728 (2017)
Acknowledgements
The authors would like to thank the associate editor and two anonymous referees for their helpful comments on improving the quality of this paper. Thanks also go to Professor Terry Rockafellar for his comments on the unboundedness of the Lagrangian multipliers of the KKT solution mapping of the original form leading to the current form of Example 2 during his visit to the Hong Kong Polytechnic University.
Author information
Authors and Affiliations
Corresponding author
Additional information
The research of the second author was supported in part by a start-up research grant from the Hong Kong Polytechnic University.
Appendix.
Appendix.
1.1 1: Proof of Lemma 2
Proof
Obviously if the quadratic growth condition for (D2) holds at \(({\bar{w}}, {\bar{y}}, -\mathcal {A}^*{\bar{w}} - \mathcal {B}^*{\bar{y}} - c)\in \mathrm{SOL}_{\mathrm{D2}}\), then the quadratic growth condition for (D) holds at \({\bar{y}}\in \mathrm{SOL}_{\mathrm{D}}\). Now we prove the reverse implication. We first show that there exist positive constants \(\varepsilon \) and \(\mu \) such that
It follows from \({\bar{w}}\in \mathrm{dom}\,h^*\) and the local strong convexity of \(h^*\) that there exist positive constants \(\varepsilon \) and \(\mu \) such that
Let \({\bar{x}}\in \mathrm{SOL}_P\) be given such that \(( {\bar{w}}, {\bar{y}},{\bar{s}}, {\bar{x}})\) satisfies the KKT optimality condition (10). By the convexity of \(p^*\), we also get
Note that \(\mathcal {B}{\bar{x}}- b\in \mathcal {N}_{\mathcal {Q}^\circ }({\bar{y}})\) implies \(\langle \mathcal {B}{\bar{x}}-b, y\rangle \leqslant 0\) for any \(y\in \mathcal {Q}^\circ \) and \(\langle \mathcal {B}{\bar{x}}-b, {\bar{y}}\rangle = 0\). One can thus derive the inequality (31) by adding the two inequalities (32) and (33). Now by shrinking \(\varepsilon \) if necessary, we have, for any \((w,y,s)\in \mathrm{F}_{\mathrm{D2}}\cap \mathbb {B}_{\varepsilon }({\bar{w}}, {\bar{y}}, {\bar{s}})\),
where in the second inequality the first term is due to the assumed quadratic growth condition for (D) at \({\bar{y}}\) with modulus \(\kappa _2\), and the second term comes from (31). Finally, it follows that for any \({\hat{y}}\in \mathrm{SOL}_{\mathrm{D}}\) and any \((w,y,s)\in \mathrm{F}_{\mathrm{D2}}\cap \mathbb {B}_{\varepsilon }({\bar{w}}, {\bar{y}}, {\bar{s}})\),
which, with \({\hat{y}}: = \varPi _{\mathrm{SOL}_{\mathrm{D}}}(y)\), implies
Thus, the quadratic growth condition (11) holds at \(({\bar{w}}, {\bar{y}}, {\bar{s}})\in \mathrm{SOL}_{\mathrm{D2}}\) for (D2). \(\square \)
1.2 2: Proof of Proposition 1(c)
Proof
The convergence of \(\{z^k\}\) under criterion (A) has been proven in Proposition 1. To establish the desired convergence rate, we first recall that the calmness of the mapping \(T^{-1}\) at the origin for \(z^{\infty }\) with modulus \(\kappa \) asks for the existence of positive constants \(\varepsilon \) and \(\delta \) such that
From parts (a) and (b) in Proposition 1 and the convergence of \(\{z^k\}\), we obtain that
which, imply the existence of a nonnegative integer \({\bar{k}}\) such that
Now taking \({\bar{z}} = \varPi _{T^{-1}(0)}(z^k)\) in Proposition 1(b), we deduce that for any \(k\geqslant 0\),
Thus, it holds that
Hence, if criterion (B) is also executed, we have that for any \(k\geqslant {\bar{k}}\),
Then the inequality in part (c) of Proposition 1 readily follows from the fact that \(\text {dist}(z^{k+1}, T^{-1}(0))\leqslant \Vert z^{k+1} - \varPi _{T^{-1}(0)}(P_k(z^k))\Vert \) for any \(k\geqslant 0\). \(\square \)
1.3 3: Proof of Proposition 3(b)
Proof
By Lemma 1(b) and the convergence of \(\{y^k\}\), we have \(\mathrm{dist}\,(0, T_l(x^{k+1},y^{k+1}))\rightarrow 0\) under criterion \(({\widetilde{B}})\). Therefore, by the upper Lipschitz continuity of \(T_l^{-1}\) at the origin, we can derive, for k sufficiently large,
This completes the proof of this part. \(\square \)
Rights and permissions
About this article
Cite this article
Cui, Y., Sun, D. & Toh, KC. On the R-superlinear convergence of the KKT residuals generated by the augmented Lagrangian method for convex composite conic programming. Math. Program. 178, 381–415 (2019). https://doi.org/10.1007/s10107-018-1300-6
Received:
Accepted:
Published:
Issue Date:
DOI: https://doi.org/10.1007/s10107-018-1300-6
Keywords
- Augmented Lagrangian method
- Convex composite conic programming
- R-superlinear
- Quadratic growth condition
- Implementable criteria