Abstract
We study four proofs that the Gittins index priority rule is optimal for alternative bandit processes. These include Gittins’ original exchange argument, Weber’s prevailing charge argument, Whittle’s Lagrangian dual approach, and Bertsimas and Niño-Mora’s proof based on the achievable region approach and generalized conservation laws. We extend the achievable region proof to infinite countable state spaces, by using infinite dimensional linear programming theory.
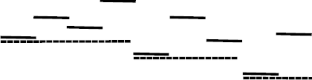
Similar content being viewed by others
References
Anderson, E., & Nash, P. (1987). Linear programming in infinite dimensional spaces. Theory and application. Chichester: Wiley-Interscience.
Barvinok, A. (2002). A course in convexity. AMS graduate studies in mathematics: Vol. 54.
Battacharya, P., Georgiadis, L., & Tsoucas, P. (1992). Extended polymatroids, properties and optimization. In E. Balas, G. Cornnéjols, & R. Kannan edrs (Eds.), Integer programming and combinatorial optimization, IPCO2 (pp. 298–315). Pittsburgh: Carnegie-Mellon University Press.
Bellman, R. (1956). A problem in the sequential design of experiments. Sankhia, 16, 221–229.
Bertsimas, D., & Niño-Mora, J. (1996). Conservation laws, extended polymatroids and multi-armed bandit problems. Mathematics of Operations Research, 21, 257–306.
Chakravorty, J., & Mahajan, A. (2013). Multi-armed bandits, Gittins index, and its calculation. http://www.ece.mcgill.ca/~amahaj1/projects/bandits/book/2013-bandit-computations.pdf.
Dacre, M., Glazebrook, K., & Niño-Mora, J. (1999). The achievable region approach to the optimal control of stochastic systems. Journal of the Royal Statistical Society, Series B, Methodological, 61, 747–791. With discussion.
Denardo, E. V., Park, H., & Rothblum, U. G. (2007). Risk-sensitive and risk-neutral multiarmed bandits. Mathematics of Operations Research, 32(2), 374–394.
Denardo, E. V., Feinberg, E. A., & Rothblum, U. G. (2013). The multi-armed bandit, with constraints. Annals of Operations Research, 208, 37–62. Volume 1 of this publication.
Edmonds, J. (1970). Submodular functions, matroids and certain polyhedra. In R. Guy, H. Hanani, N. Sauer, & J. Schönheim (Eds.), Proceedings of Calgary international conference on combinatorial structures and their applications (pp. 69–87). New York: Gordon and Breach.
Federgruen, A., & Groenevelt, H. (1988). Characterization and optimization of achievable performances in general queuing systems. Operations Research, 36, 733–741.
Gittins, J. C., & Jones, D. M. (1974). A dynamic allocation indices for the sequential design of experiments. In J. Gani, K. Sarkadi, & I. Vince (Eds.), Progress in statistics, European Meeting of statisticians 1972 (Vol. 1, pp. 241–266). Amsterdam: North Holland.
Gittins, J. C. (1979). Bandit processes and dynamic allocation indices. Journal of the Royal Statistical Society, Series B, 14, 148–167.
Gittins, J. C. (1989). Multiarmed bandits allocation indices. New York: Wiley.
Gittins, J. C., Glazebrook, K., & Weber, R. R. (2011). Multiarmed bandits allocation indices (2nd ed.). New York: Wiley.
Glazebrook, K. D., & Garbe, R. (1999). Almost optimal policies for stochastic systems which almost satisfy conservation laws. Annals of Operations Research, 92, 19–43.
Glazebrook, K., & Niño-Mora, J. (2001). Parallel scheduling of multiclass M/M/m queues: approximate and heavy-traffic optimization of achievable performance. Operations Research, 49, 609–623.
Harrison, J. M. (1975). Dynamic scheduling of a multiclass queue, discount optimality. Operations Research, 23, 270–282.
Ishikada, A. T., & Varaiya, P. (1994). Multi-armed bandit problem revisited. Journal of Optimization Theory and Applications, 83, 113–154.
Kaspi, H., & Mandelbaum, A. (1998). Multi-armed bandits in discrete and continuous time. The Annals of Applied Probability, 8, 1270–1290.
Katehakis, M. N., & Derman, C. (1986). Computing optimal sequential allocation rules in clinical trials. In J. Van Ryzin (Ed.), I.M.S. Lecture notes-monograph series: Vol. 8. Adaptive statistical procedures and related topics (pp. 29–39).
Katehakis, M. N., & Veinott, A. F. (1987). The multi-armed bandit problem: decomposition and computation. Mathematics of Operations Research, 12, 262–268.
Katehakis, M. N., & Rothblum, U. (1996). Finite state multi-armed bandit sensitive-discount, average-reward and average-overtaking optimality. The Annals of Applied Probability, 6(3), 1024–1034.
Klimov, G. P. (1974). Time sharing service systems I. Theory of Probability and Its Applications, 19, 532–551.
Meilijson, I., & Weiss, G. (1977). Multiple feedback at a single server station. Stochastic Processes and Their Applications, 5, 195–205.
Mandelbaum, A. (1986). Discrete multi-armed bandits and multiparameter processes. Probability Theory and Related Fields, 71, 129–147.
Mitten, L. G. (1960). An analytic solution to the least cost testing sequence problem. Journal of Industrial Engineering, 11(1), 17.
Niño-Mora, J. (2001). Restless bandits, partial conservation laws and indexability. Advances in Applied Probability, 33, 76–98.
Niño-Mora, J. (2002). Dynamic allocation indices for restless projects and queuing admission control: a polyhedral approach. Mathematical Programming Series A, 93, 361–413.
Niño-Mora, J. (2006). Restless bandit marginal productivity indices, diminishing returns and optimal control of make-to-order/make-to-stock M/G/1 queues. Mathematics of Operations Research, 31, 50–84.
Niño-Mora, J. (2007). A 2/3n 3 fast-pivoting algorithm for the Gittins index and optimal stopping of a Markov chain. INFORMS Journal on Computing, 19, 596–606
Ross, S. M. (1983). Introduction to stochastic dynamic programming. New York: Academic Press.
Royden, H. L. (1971). Real analysis. New York: Macmillan.
Rudin, W. (1964). Principles of mathematical analysis. New York: McGraw-Hill.
Sevcik, K. C. (1974). Scheduling for minimum total loss using service time distributions. Journal of the Association for Computing Machinery, 21, 66–75.
Shanthikumar, J. G., & Yao, D. D. (1992). Multiclass queuing systems: polymatroidal structure and optimal scheduling control. Operations Research 40, 293–299.
Shapiro, A. (2001). On duality theory of conic linear problems. In M. A. Goberna & M. A. Lopez (Eds.), Semi-infinite programming (pp. 135–165). Netherlands: Kluwer.
Sonin, I. M. (2008). A generalized Gittins index for a Markov chain and its recursive calculation. Statistics & Probability Letters, 78(12), 1526–1533.
Tcha, D., & Pliska, S. R. (1975). Optimal control of single server queuing networks and multi-class M/G/1 queues with feedback. Operations Research, 25, 248–258.
Tsitsiklis, J. N. (1994). A short proof of the Gittins index theorem. The Annals of Applied Probability, 4, 194–199.
Tsoucas, P. (1991). The region of achievable performance in a model of Klimov. Research Report RC16543, IBM T.J. Watson Research Center Yorktown Heights, New York.
Varaiya, P., Walrand, J., & Buyukkoc, C. (1985). Extensions of the multiarmed bandit problem: the discounted case. IEEE Transactions on Automatic Control, AC-30, 426–439.
Weber, R. R. (1992). On the Gittins index for multiarmed bandits. Annals of Probability, 2, 1024–1033.
Weber, R. R., & Weiss, G. (1990). On an index policy for restless bandits. Journal of Applied Probability, 27, 637–648.
Weber, R. R., & Weiss, G. (1991). Addendum to ‘on an index policy for restless bandits’. Advances in Applied Probability, 23, 429–430.
Weiss, G. (1988). Branching bandit processes. Probability in the Engineering and Informational Sciences, 2, 269–278.
Whittle, P. (1980). Multi-armed bandits and the Gittins index. Journal of the Royal Statistical Society, Series B, 42, 143–149.
Whittle, P. (1981). Arm acquiring bandits. Annals of Probability, 9, 284–292.
Whittle, P. (1988). Restless bandits: activity allocation in a changing world. Journal of Applied Probability, 25A, 287–298. In J. Gani (Ed.), A celebration of applied probability.
Whittle, P. (1990). Risk-sensitive optimal control. New York: Wiley.
Author information
Authors and Affiliations
Corresponding author
Additional information
E. Frostig’s research supported in part by Network of Excellence Euro-NGI.
G. Weiss’s research supported in part by Israel Science Foundation Grants 249/02, 454/05, 711/09 and 286/13.
Appendix: Proofs of index properties
Appendix: Proofs of index properties
In this appendix we present the proofs of some results postponed in the paper.
Proof of Theorem 2.1
The direct proof is quite straightforward. Note that in (2.1) the value of ν(i,σ) for each stopping time σ is a ratio of sums over consecutive times. Hence to compare these we use the simple inequality:
We show that if we start from i and wish to maximize ν(i,σ) it is never optimal to stop in state j if ν(j)>ν(i) or to continue in state j if ν(j)<ν(i), which allows us to consider only stopping time of the form (2.3). We then assume that no stopping time achieves the supremum, and we construct an increasing sequence of stopping times with increasing ratio, which converges to τ(i) which therefore must achieve the supremum, and from this contradiction we deduce that the supremum is achieved. Finally we show that since the supremum is achieved, it is achieved by τ(i) as well as by all stopping times of the form (2.3).
Step 1: Any stopping time which stops while the ratio is >ν(Z(0)) does not achieve the supremum. Assume that Z(0)=i, fix j such that ν(j)>ν(i), and consider a stopping time σ such that:
By the definition (2.1) there exists a stopping time σ′ such that \(\nu(j,\sigma ')> \frac{\nu(j)+\nu(i)}{2}\). Define σ′=0 for all initial values ≠j. Then:
Step 2: Any stopping time which continues when the ratio is <ν(Z(0)) does not achieve the supremum. Assume that Z(0) = i, fix j such that ν(j) < ν(i), and let σ′ = min{t:Z(t) = j}. Consider any stopping time σ which does not always stop when it reaches state j, and assume that:
Then:
Steps 1, 2 show that the supremum can be taken over stopping times σ>0 which satisfy (2.3), and we restrict attention to such stopping times only.
Step 3: The supremum is achieved. If τ(i) is the unique stopping time which satisfies (2.3) then it achieves the supremum and there is nothing more to prove. Assume that the supremum is not achieved. We now consider a fixed stopping time σ>0 which satisfies (2.3) and:
This is possible, since τ is not unique, and since the supremum is not achieved. Assume that σ stops at a time <τ(i) when the state is Z(σ)=j. By (2.3), ν(j)=ν(i). We can then find σ′ such that \(\nu(j,\sigma ') \ge \frac{\nu_{0}+\nu(i)}{2}\). Define σ′ accordingly for the value of Z(σ) whenever σ<τ(i), and let σ′=0 if σ=τ(i). Let σ 1=σ+σ′. Clearly we have (repeat the argument of step 1):
We can now construct a sequence of stopping times, with
which will continue indefinitely, or will reach \(\mathbb{P}(\sigma_{n_{0}} = \tau(i))=1\), in which case we define σ n =τ(i),n>n 0.
It is easy to see that min(n,σ n )=min(n,τ(i)), hence σ n ↗τ(i) a.s. It is then easy to see (use dominated or monotone convergence) that ν(i,σ n )↗ν(i,τ(i)). But this implies that ν(i,σ)<ν(i,τ(i)). Hence the assumption that the supremum is not achieved implies that the supremum is achieved by τ(i), which is a contradiction. Hence, for any initial state Z(0)=i the supremum is achieved by some stopping time, which satisfies (2.3).
Step 4: The supremum is achieved by τ(i). Start from Z(0)=i, and assume that a stopping time σ satisfies (2.3) and achieves the supremum. Assume
and take the event that σ stops at a time <τ(i) when the state is Z(σ)=j. By (2.3) ν(j)=ν(i). We can then find σ′ which achieves the supremum, ν(j,σ′)=ν(j)=ν(i). Define σ′ accordingly for the value of Z(σ) whenever σ<τ(i), and let σ′=0 if σ=τ(i). Let σ 1=σ+σ′. Clearly we have:
We can now construct an increasing sequence of stopping times, σ n ↗τ(i) a.s., and all achieving ν(i,σ n )=ν(i). Hence (again use dominated or monotone convergence) ν(i,τ(i))=ν(i).
Step 5: The supremum is achieved by any stopping time which satisfies ( 2.3 ). Let σ satisfy (2.3). Whenever σ<τ(i) and Z(σ)=j, we will have τ(i)−σ=τ(j), and ν(j,τ(i)−σ)=ν(i). Hence:
This completes the proof. □
Proof of Proposition 2.2
Step 1: We show that ν(i)≤γ(i). Consider any y<ν(i), let \(M=\frac{y}{1-\alpha}\). By definition (2.1) there exists a stopping time τ for which ν(i,τ)>y.
Hence, a policy π which from state i will play up to time τ and then stop and collect the reward M, will have:
Hence V r (i,M)>M, and i belongs to the continuation set, for standard arm reward y, (or fixed charge y, or terminal reward M). Hence, M(i)≥M, and γ(i)≥y. But y<ν(i) was arbitrary. Hence, γ(i)≥ν(i).
Step 2: We show that ν(i)≥γ(i). Consider any y<γ(i). Let \(M=\frac{y}{1-\alpha}\), and consider τ(i,M) and V r (i,M). Writing (2.14), and using the fact that for M<M(i) we have i∈C M and V r (i,M)>M:
But this means that ν(i,τ(i,M))>y. Hence, ν(i)>y. But y<γ(i) was arbitrary. Hence, ν(i)≥γ(i).
Step 3: Identification of lim m↗M(i) τ(i;m) with τ(i) in ( 2.1 ). Clearly, starting from state i, τ(i;m) with m<M(i) will continue for all states j with M(j)≥M(i), and for every state j with M(j)<M(i) it will retire at state j if M(j)<m<M(i), so lim m↗M(i) τ(i;m)=τ(i;M(i))=τ(i). The last equality holds since we showed that \(\frac{M(i)}{1-\alpha}=\gamma(i)=\nu(i)\). □
Proof of Proposition 2.9
The equivalence of the two algorithms is easily seen. Step 1 is identical. Assume that the two algorithms are the same for steps 1,…,k−1. We then have in step k, for any \(i \in S_{\varphi(k-1)}^{-}\) that:
and so the supremum in step k is achieved by the same φ(k) in both versions of the algorithm. The quantities y S appear in the 4th proof, Sect. 3.4. □
Proof of Proposition 4.2
(i) The definition of Ax(S) implies that Ax(∅)=0 because it is an empty sum. Trivially \(T_{j}^{\emptyset}=\infty\), so \(\alpha^{T_{j}^{\emptyset}}=0\), hence the definition (3.20) of b(S) implies b(∅)=0. Thus (i) holds.
(ii) First we show that if x∈X, and y(S)=Ax(S), then g y is of bounded variation. Let \(x^{+}=\{x_{i}^{+} = \max(x_{i},0)\}_{i\in E}\), and \(x^{-}=\{x_{i}^{-} = \max(-x_{i},0)\}_{i\in E}\). Clearly x +,x −∈X and g y (v)=Ax +(S(v))−Ax −(S(v)). We have:
S(v) is increasing in v, as a result \(A_{j}^{S(v)}\) is decreasing in v. Also E∖S(v) is decreasing in v. Hence both terms in (6.9) decrease in v. Thus Ax +(S(v)), as the difference of two decreasing functions of v is of bounded variation. Similarly Ax −(S(v)), is of bounded variation. Hence, g y is of bounded variation.
Next we take y=b, and consider g y . g y (v)=b(S(v)) increases in v thus it is of bounded variation. This proves (ii).
(iii) To calculate the limits from the left we need to prove some continuity results: consider an increasing sequence {v n }, such that lim n→∞ v n =v, and v n <v. Consider first S(v n ) and S −(v). Then S(v n ) are increasing and \(\lim_{n\to \infty} S(v_{n}) = \bigcup_{n=0}^{\infty} S(v_{n}) = S^{-}(v)\). To see this, note the if i∈S −(v) then ν(i)<v, hence for some n 0 we have ν(i)≤v n for all n≥n 0, hence i∈S(v n ),n≥n 0.
Next consider \(T_{j}^{S(v_{n})}\) and \(T_{j}^{S^{-}(v)}\) for some given sample path. If \(T_{j}^{S^{-}(v)}= \infty\) then \(T_{j}^{S(v_{n})}= \infty\) for all n. If \(T_{j}^{S^{-}(v)}= t < \infty\) then we have Z(t)=i∈S −(v), but in that case i∈S(v n ),n>n 0, so \(T_{j}^{S(v_{n})}=t\) for all N≥n 0. Thus we have that \(T_{j}^{S(v_{n})}\) is non-increasing in n and converges to \(T_{j}^{S^{-}(v)}\) for this sample path. Hence \(T_{j}^{S(v_{n})} \searrow_{\rm a.s.} T_{j}^{S^{-}(v)}\).
We now have that \(\sum_{t=0}^{T_{j}^{S(v_{n})}-1} \alpha^{t} \searrow_{\rm a.s.} \sum_{t=0}^{T_{j}^{S^{-}(v)}-1}\alpha^{t}\), and because ∑ t α t are uniformly bounded, \(A_{j}^{S(v_{n})} \searrow A_{j}^{S^{-}(v)}\).
Consider now Ax(S(v n )) and Ax(S −(v)). We need to show that as n→∞,
which follows from \(A_{i}^{S}\) bounded by \(\frac{1}{1-\alpha}\), x i absolutely convergent, and S(v n )↗S −(v). To explain this a little further: Since x j are absolutely convergent, for every ϵ>0 we can find a finite subset of states E 0 such that \(\frac{2}{1-\alpha} \sum_{j\in E\backslash E_{0}} |x_{j}| < \frac {1}{2}\epsilon\). If we now examine the sums only over j∈E 0, clearly the first sum can be made arbitrarily small as n→∞, and the second sum becomes empty as n→∞.
Finally for any given Z(0), \(\mathbb{E}(\alpha^{T_{Z_{k}(0)}^{S(v_{n})}}) \nearrow \mathbb{E}(\alpha^{T_{Z_{k}(0)}^{S^{-}(v)}}),k=1,\ldots,N\) and hence by definition (3.20) b(S(v n ))↗b(S −(v)). This completes the proof that g y (v n )→y(S −(v)).
To show continuity from the right, consider a decreasing sequence {v n }, such that lim n→∞ v n =v, and v n >v. Consider the sequence of sets S(v n ) and the set S(v). Then S(v n ) are decreasing and \(\lim_{n\to \infty} S(v_{n}) = \bigcap_{n=0}^{\infty} S(v_{n}) = S(v)\). To see this, note the if i∉S(v) then ν(i)>v, hence for some n 0 we have ν(i)>v n for all n≥n 0, hence i∉S(v n ),n≥n 0.
The remaining steps of the proof are as for the limit from the left: one shows that \(T_{j}^{S(v_{n})} \nearrow_{\rm a.s.} T_{j}^{S(v)}\), and so on. This completes the proof of (iii).
(iv) We wish to show
Clearly, if {i:ν(i)=v}=∅ then both sides are 0, and if {i:ν(i)=v} consists of a single state {i} then \(S(v)=S_{i}, S^{-}(v)=S^{-}_{i}\) and there is nothing to prove. If {i:ν(i)=v} consists of a finite set of states with i 1≺i 2…≺i M , then S(i k )=S −(i k+1) and the summation over i k is a collapsing sum. If {i:ν(i)=v} is infinite countable but well ordered, then we can order {i:ν(i)=v} as i 1≺i 2≺… (ordinal type ω), and the infinite sum on the right is a collapsing sum, which converges to the right hand side.
The difficulty here is that in the general case {i:ν(i)=v} may not be well ordered by ≺, and it is this general case which we wish to prove. We do so by a sample path argument, using the fact that the sequence of activation times, t=1,2,… is well ordered. In our sample path argument we make use of the sequence of stopping times and states \({\mathcal {T}}_{\ell}\) and k ℓ , defined in (2.21). Recall that this is the sequence of stopping times and states at which the index sample path ‘loses priority height’, in that at time \({\mathcal {T}}_{\ell}\) it reaches a state k ℓ which is of lower priority than all the states encountered in \(0<t<{\mathcal {T}}_{\ell}\).
Fix a value of v, and consider a sample path starting from Z(0)=j. Then for this sample path we will have integers \(0 \le \underline{L} < \overline{L} \le \infty\) such that:
It is possible that \(\underline{L} = \infty\) because the sample path never reaches S(v). It is also possible that \(\overline{L} = \underline{L}+1\), either because {i:ν(i)=v}=∅, or because the first visit of the sample path to S(v) is directly to a state with index <v; in each of these cases \(T_{j}^{S(v)} = T_{j}^{S^{-}(v)}\). Otherwise, if \(\overline{L} - \underline{L} > 1\), then \({\mathcal {T}}_{\underline{L}+1}= T_{j}^{S_{k_{\underline{L}+1}}}=T_{j}^{S(v)}\) will be the first visit of the process in S(v), and \({\mathcal {T}}_{\overline{L}}= T_{j}^{S^{-}_{k_{\overline{L}-1}}}=T_{j}^{S^{-}(v)}\) will be the first visit of the process in S −(v).
We can then write:
where the second equality follows from \(T_{j}^{S^{-}_{i}}=T_{j}^{S_{i}}\) for all i:ν(i)=v except for \(k_{\ell}, \underline{L} < \ell < \overline{L}\).
By taking expectations we now get that:
and also, for all j∈S(v)∖S −(v):
Next we note that
where \(T_{\mathbf{Z}(0)}^{S} = \sum_{n=1}^{N} T_{Z_{n}(0)}^{S}\). Hence, using the same sample path result (6.10) for each of Z n (0) we get
We now come to evaluate Ax(S −(v))−Ax(S(v)). We need to show that for all x∈X:
Since this has to hold for all x, we need to show for every j∈E that the coefficients of x j satisfy the equalities individually.
For j∈S −(v) clearly \(j\in S(v), S_{i}, S^{-}_{i}, i:\nu(i)=v\), we need to check that:
which we have just shown.
For j∈S(v)∖S −(v) we have: j∈S(v), and for i:ν(i)=v: j∈S i if i⪰j, and \(j\in S^{-}_{i}\) if i≻j. Hence, the coefficients of j which we need to compare are:
Which amounts to:
which we have also shown. □
Rights and permissions
About this article
Cite this article
Frostig, E., Weiss, G. Four proofs of Gittins’ multiarmed bandit theorem. Ann Oper Res 241, 127–165 (2016). https://doi.org/10.1007/s10479-013-1523-0
Published:
Issue Date:
DOI: https://doi.org/10.1007/s10479-013-1523-0