Abstract
With the rapid development of the Internet and data-processing technologies, Internet sentiment analysis can be used to explore many possibilities, from Internet news about products or the influence of product price to the influence of sale behaviour and important brand strategies. In this paper, we analyse news affecting the price of products, and establish a new model for price prediction. The results show that significant news events have an impact on the sale prices of electronic products, and can improve the accuracy of price forecasts. Thus, the contribution of this paper is to propose a new forecasting model for the price of e-commerce products.



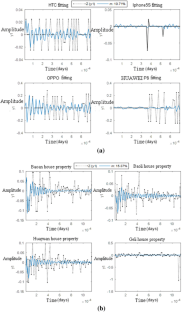




Similar content being viewed by others
References
Li, Z., & Li, Z. (2011). 2020 Internet data will be 44 times the current. Information System Engineering, 6, 11.
Lagarto, J., De Sousa, J., Martins, A., & Ferrão, P. (2012). Price forecasting in the day-ahead Iberian electricity market using a conjectural variations ARIMA model. In 2012 9th International conference on the European Energy Market (EEM) (pp. 1–7). IEEE.
Hsu, C. C., & Chen, C. Y. (2003). Applications of improved grey prediction model for power demand forecasting. Energy Conversion and Management, 44(02), 2241–2249.
González, A. M., Roque, A. M. S., & García-González, J. (2005). Modeling and forecasting electricity prices with input/output hidden Markov models. IEEE Transactions on Power Systems, 20(1), 13–24.
Yousefi, S., Weinreich, I., & Reinarz, D. (2005). Wavelet-based prediction of oil prices. Chaos, Solitons and Fractals, 25(2), 265–275.
Lippmann, R. P. (1987). An introduction to computing with neural nets. IEEE Assp Magazine, 4(2), 4–22.
Kimoto, T., Asakawa, K., Yoda, M., et al. (1990). Stock market prediction system with modular neural network. In 1990 IJCNN international joint conference on neural networks (vol. 1, pp. 1–6). IEEE.
Lawrence, R. (1998). Using neural networks to forecast stock market prices. Manitoba: University of Manitoba.
Mombeini, H., & Yazdani-Chamzini, A. (2015). Modeling gold price via artificial neural network. Journal of Economics, Business and Management, 3(7), 699–703.
Liu, Z., Wang, Y., Zhu, S., Zhang, B., & Wei, L. (2015). Steel Prices Index Prediction in China based on BP neural network. In Z. Zhang, Z. Shen, J. Zhang & R. Zhang (Eds.), LISS 2014 (pp. 603–608). Berlin: Springer.
Li, Z., & Li, G. (2010). Establishment of eggs market price short-term prediction model. Food and Nutrition in China, 6, 36–40.
Xiao, L., & Zhong, W. (2009). Predictive analysis of China’s oil price based upon ARIMA model. Journal of Nanjing University of Aeronautics Astronautics (Social Sciences), 11(4), 41–46.
Li, G., Xu, S., Li, Z., & Dong, X. (2010). Study on super short-term forecasting for market price of agro-products—Based on modern times series modeling of daily wholesale price of tomatoes. Journal of Huazhong Agricultural University (Social Sciences Edition), 6, 40–45.
Ji, J. (2008). Application of support vector machine in prediction of Consumer Price Index. Modern Computer, 6, 64–66.
Wu, J. (2010). Price tendency prediction and countermeasures in Guangxi from 2010 to 2011. Academic Forum, 33(3), 100–103.
Zhang, D., & Ren, X. (2014). Analysis on the characteristics and influencing factors of price fluctuation of agricultural products in China: Based on time series decomposition and VAR model. Price Theory and Practice, 9, 69–71.
Zhao, C. L., & Wang, B. (2014). Forecasting crude oil price with an autoregressive integrated moving average (ARIMA) model. In Fuzzy information and engineering and operations research and management (pp. 275–286). Berlin: Springer.
Mustaffa, Z., Yusof, Y., & Kamaruddin, S. S. (2014). Enhanced Artificial Bee Colony for training least squares support vector machines in commodity price forecasting. Journal of Computational Science, 5(2), 196–205.
Zou, K., Li, B., & Zhou, X. (2009). Application of grey system model in stock prediction. In B. Cao, T. F. Li & C. Y. Zhang (Eds.), Fuzzy information and engineering volume 2: Advance in intelligent and soft computing (vol. 62, pp. 1561–1567). Berlin: Springer.
Hang, T. N., & Nabney, I. T. (2010). Short-term electricity demand and gas price forecasts using wavelet transforms and adaptive models. Energy, 35(9), 3674–3685.
Pai, P. F., & Lin, C. S. (2005). A hybrid ARIMA and support vector machines model in stock price forecasting. Omega, 33(6), 497–505.
Tseng, F. M., Yu, H. C., & Tzeng, G. H. (2001). Combining neural network model with seasonal time series ARIMA model. Technological Forecasting and Social Change, 69(1), 71–87.
Janoski, T., & Isaac, L. W. (1994). Introduction to time-series analysis. Comparative Political Economy of the Welfare State, 90(1), 110.
Cortes, C., & Vapnik, V. (1995). Support-vector networks. Machine Learning, 20(3), 273–297.
McCulloch, W. S., & Pitts, W. (1943). A logical calculus of the ideas immanent in nervous activity. The Bulletin of Mathematical Biophysics, 5(4), 115–133.
Huiwen, W., & Jie, M. (2007). Predictive modeling on multivariate linear regression. Journal of Beijing University of Aeronautics and Astronautics, 33(4), 500–504.
Wei, B. (2004). Nonlinear regression model. In Proceedings of the ninth annual academic conference of Jiangsu provincial Statistical Research Association.
Yong, Z., & Xiaowo, T. (1995). Forecasting with general logistic curves. Application of Statistics and Management, 1, 41–46.
Jank, W., Shmueli, G., & Wang, S. (2006). Dynamic, real-time forecasting of online auctions via functional models. In Acm sigkdd international conference on knowledge discovery and data mining (pp. 580–585).
Enders, B. W. (2010). Applied econometric time series, 3rd Edition[J].
Freeman, J. R. (1983). Granger causality and time series analysis of political relationships[J]. American Journal of Political Science, 27(2), 327–358.
Özdemir, D. (2016). The analysis of time series. In Applied statistics for economics and business (pp. 257–291). Cham: Springer.
Author information
Authors and Affiliations
Corresponding author
Rights and permissions
About this article
Cite this article
Tseng, KK., Lin, RY., Zhou, H. et al. Price prediction of e-commerce products through Internet sentiment analysis. Electron Commer Res 18, 65–88 (2018). https://doi.org/10.1007/s10660-017-9272-9
Published:
Issue Date:
DOI: https://doi.org/10.1007/s10660-017-9272-9