Abstract
Recommender system is a computer-based intelligent technique which facilitates the customers to fulfill their purchase requirements. In addition to this, it also helps retailers to manage the supply chain of their business and to develop different business strategies keeping in pace with the current market. Supply chain management (SCM) involves the streamlining of a business’s supply-side activities to remain competitive in the business landscape. Maximizing the customer value is another important activity of SCM to gain an advantage in the market. In this work, the K-Means clustering algorithm has been used for the effective segmentation of customers who have bought apparel items. PCA has been used for dimensionality reduction of different features of products and customers. The main focus of this work is to determine the different possible associations of customers in terms of brand, product, and price from their purchase habits. The result shows that the clusters made by the algorithm based on PCA and K-Means are similar and the results are acceptable on the basis of feedback received from existing customers and satisfies the customers’ requirements based on the amount of money (price range) the customers want to spend while doing online shopping. The features of products purchased by customers were combined together to generate a unique product key for business, and a model was prepared to segment products based on the volume of products sold and revenue generated, and the price of products sold and revenue generated. This work, in the long run, will help business houses to build a sustainable, profitable, and scalable e-commerce business. Environmental, social, and economic aspects are important to make e-commerce more sustainable for the benefit of the society.

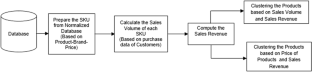
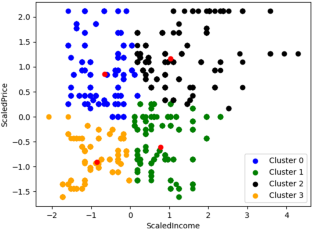


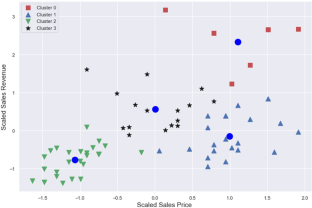
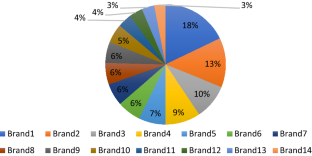
Similar content being viewed by others
References
Kyoung-jae K, Hyunchul A (2008) A recommender system using GA K-Means clustering in an online shopping market. Expert Syst Appl 34(2):1200–1209
Shinde SK, Kulkarni U (2012) Hybrid personalized recommender system using centering-bunching based clustering algorithm. Expert Syst Appl 39(1):1381–1387
Gong S (2010) A collaborative filtering recommendation algorithm based on user clustering and item clustering. J Softw 5(7):745–752
Cho YS, Moon SC, Jeong S, Oh IB, Ryu KH (2014) Clustering method using weighted preference based on RFM score for personalized recommendation system in u-Commerce. In: Jeong YS, Park YH, Hsu CH, Park J (eds) Ubiquitous information technologies and applications, vol 280. Lecture Notes in Electrical Engineering. Springer, Berlin
Mohamed F, Mohamed C (2014) Application of data mining in e-Commerce. J Inf Technol Res 7(4):79–91
Ester M, Kriegel H P, Sander J, Xu X (1996) A density-based algorithm for discovering clusters in large spatial databases with noise. In: Proceedings of the second international conference on knowledge discovery and data mining, Portland, Oregon, pp 226–231
Pitsilis G, Zhang X, Wang W (2011) Clustering recommenders in collaborative filtering using explicit trust information. In: Wakeman I, Gudes E, Jensen CD, Crampton J (eds) Trust Management V. IFIPTM 2011. IFIP Advances in Information and Communication Technology. Springer, Berlin, pp 82–97
Choi K, Yoo D, Kim G, Suh Y (2012) A hybrid online-product recommendation system: combining implicit rating-based collaborative filtering and sequential pattern analysis. Electron Commer Res Appl 11(4):309–317
Oyelade OJ, Oladipupo OO, Obagbuwa IC (2010) Application of k-Means clustering algorithm for prediction of students academic performance. Int J Comput Sci Inf Secur 7(1):292–295
Mathivanan NMN, Ghani NA, Janor RM (2018) Improving classification accuracy using clustering technique. Bull Electr Eng Inform 7(3):465–470
Jolliffe IT (2002) Principal component analysis, 2nd edn. Springer, New York
Zha H, Ding C, Gu M, He X, Simon H (2001) Spectral relaxation for K-Means clustering. In: Advances in neural information processing systems 14 (NIPS’01), pp 1057–1064
Ding C, He X (2004) K-Means clustering via principal component analysis. In: Proceedings of the international conference on machine learning, pp 225–232
Chuan NK, Sivaji A, Shahimin MM, Saad N (2013) Kansei engineering for e-commerce sunglasses selection in Malaysia. In: Procedia—Social and Behavioral Sciences, the 9th international conference on cognitive science 2013, vol 97, pp 707–714
Nilashi M, Ibrahim OB, Ithnin N, Sarmin NH (2015) A multi-criteria collaborative filtering recommender system for the tourism domain using Expectation Maximization (EM) and PCA–ANFIS. Electron Commer Res Appl 14(6):542–562
Wang Y, Wu Z, Bu Z, Cao J, Yang D (2016) Discovering shilling groups in a real e-commerce platform. Online Inf Rev 40:62–78
Shang W, Zhu H, Huang H, Qu Y, Lin Y (2006) The improved ontology kNN algorithm and its application. 2006. ICNSC ‘06. In: Proceedings of the 2006 IEEE international conference on networking, sensing and control, ICNSC2006, pp 198–203
Celebi M, Kingravi H, Vela P (2013) A comparative study of efficient initialization methods for the k-Means clustering algorithm. Expert Syst Appl 40(1):200–210
Consumers above 37 years are highest e-commerce spenders: WATConsult’s e-commerce report. https://bestmediainfo.com/2018/08/consumers-above-37-years-are-highest-e-commerce-spenders-watconsult-s-e-commerce-report/ Accessed 30 Aug 2018
Ahmed AI (2018) Understanding the factors affecting the adoption of green computing in the Gulf Universities. Int J Adv Comput Sci Appl 9(3):304–3011
Author information
Authors and Affiliations
Corresponding author
Additional information
Publisher's Note
Springer Nature remains neutral with regard to jurisdictional claims in published maps and institutional affiliations.
Rights and permissions
About this article
Cite this article
Bandyopadhyay, S., Thakur, S.S. & Mandal, J.K. Product recommendation for e-commerce business by applying principal component analysis (PCA) and K-means clustering: benefit for the society. Innovations Syst Softw Eng 17, 45–52 (2021). https://doi.org/10.1007/s11334-020-00372-5
Received:
Accepted:
Published:
Issue Date:
DOI: https://doi.org/10.1007/s11334-020-00372-5