Abstract
The degradation of coastal waters and lakes is of a great concern due to their important roles in providing fresh water and food to the neighboring communities. Therefore, a robust monitoring plan is needed to assess the emerging water quality issues and identify the possible sources of pollution. Remote sensing could be an alternative invaluable approach for water quality monitoring compared to the traditional monitoring methods which are limited in terms of spatial coverage and temporal variability. The remote sensing–based water quality data can be estimated by modelling the apparent optical properties (AOPs) and/or the inherent optical properties (IOPs). Retrieving IOPs enables the estimation of aquatic biomass, primary production, and carbon pools. Therefore, several studies have invested significantly in improving the performance of the IOPs models to better estimate the water quality parameters. To assess uncertainty and improve IOP models in estimating water quality parameters, we review here studies of IOP modelling with a focus on coastal and inland waters. The review includes the most common IOP models: the GSM Semi-Analytical Bio-Optical Model, the Quasi-Analytical Algorithm (QAA), and the Generalized IOP Algorithm (GIOP). We review performance of these models and the regions in which they are applied in. Additionally, the limitations of the models are discussed and thus recommendations are proposed to overcome the uncertainties and incorporate better results from the models. This review could directly influence the science of future missions with the ability to monitor coastal and inland waters.

Similar content being viewed by others
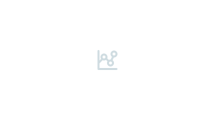
Data Availability
Data sharing is not applicable to this article as no new data were created or analyzed in this study.
References
Aguilar-Maldonado JA, Santamaría-Del-ángel E, Gonzalez-Silvera A, Sebastiá-Frasquet MT (2019) Detection of phytoplankton temporal anomalies based on satellite inherent optical properties: A tool for monitoring phytoplankton blooms. Sensors (Switzerland) 19(15). https://doi.org/10.3390/s19153339
Al Shehhi MR, Abdul Samad Y (2021) Effects Of The Covid-19 Pandemic On The Oceans. Remote Sens Lett 12(4):325–334. https://doi.org/10.1080/2150704X.2021.1880658
Al Shehhi MR, Gherboudj I, Ghedira H (2017) In situ spectral response of the Arabian Gulf and Sea of Oman coastal waters to bio-optical properties. J Photochem Photobiol B Biol 175:235–243
Al Shehhi MR, Gherboudj I, Ghedira H (2019) Detection of algal blooms over optically complex waters of the Arabian Gulf and Sea of Oman using MODIS fluorescence data. Int J Remote Sens 40(10):3751–3771
Al Shehhi MR, Gherboudj I, Zhao J, Ghedira H (2017) Improved atmospheric correction and chlorophyll-a remote sensing models for turbid waters in a dusty environment. ISPRS J Photogramm Remote Sens 133:46–60. https://doi.org/10.1016/j.isprsjprs.2017.09.011
Andrade C, Alcântara E, Bernardo N, Kampel M (2019) An assessment of semi-analytical models based on the absorption coefficient in retrieving the chlorophyll-a concentration from a reservoir. Adv Space Res 63(7):2175–2188
Arora JS (2004) Introduction to optimum design, 3rd edition. Academic Press, Oxford. https://doi.org/10.1016/B978-0-12-064155-0.X5000-9
Balasubramanian SV, Pahlevan N, Smith B, Binding C, Schalles J, Loisel H, Gurlin D, Greb S, Alikas K, Randla M, Bunkei M, Moses W, Nguyễn H, Lehmann MK, O’Donnell D, Ondrusek M, Han TH, Fichot CG, Moore T, Boss E (2020) Robust algorithm for estimating total suspended solids (TSS) in inland and nearshore coastal waters. Remote Sens Environ 246(March):111768. https://doi.org/10.1016/j.rse.2020.111768
Bartram J, Ballance R (1996) Water quality monitoring: A practical guide to the design and implementation of freshwater quality studies and monitoring programmes. London, UK: E&FN Spon
Betancur-Turizo SP, González-Silvera A, Santamaría-del-Ángel E, Tan J, Frouin R (2018) Evaluation of Semi-Analytical Algorithms to Retrieve Particulate and Dissolved Absorption Coefficients in Gulf of California Optically Complex Waters. Remote Sens 10(9):1443
Bierman P, Lewis M, Ostendorf B, Tanner J (2011) A review of methods for analysing spatial and temporal patterns in coastal water quality. Ecol Indic 11(1):103–114
Blondeau-Patissier D, Gower JFR, Dekker AG, Phinn SR, Brando VE (2014) A review of ocean color remote sensing methods and statistical techniques for the detection, mapping and analysis of phytoplankton blooms in coastal and open oceans. Prog Oceanogr 123:123–144. https://doi.org/10.1016/j.pocean.2013.12.008
Bricaud A, Morel A, Babin M, Allali K, Claustre H (1998) Variations of light absorption by suspended particles with chlorophyll a concentration in oceanic (case 1) waters: Analysis and implications for bio-optical models Abstract. Spectral absorption coefficients of total particulate matter mg m-3). As pre. J Geophys Res 103(C13):31033–31044
Chu Q, Zhang Y, Ma R, Hu M, Jing Y (2020) MODIS-based remote estimation of absorption coefficients of an Inland Turbid Lake in China. Remote Sens 12(12). https://doi.org/10.3390/rs12121940
Deng L, Zhou W, Cao W, Wang G, Zheng W, Xu Z, Li C, Yang Y, Xu W, Zeng K (2020) Evaluating semi-analytical algorithms for estimating inherent optical properties in the South China Sea. Opt Express 28(9):13155–13176
Doxaran D, Cherukuru N, Lavender SJ (2006) Apparent and inherent optical properties of turbid estuarine waters: measurements, empirical quantification relationships, and modeling. Appl Opt 45(10):2310–2324
Favareto LR, Rudorff N, Kampel M, Frouin R, Röttgers R, Doxaran D, Murakami H, Dupouy C (2018) Bio-optical characterization and ocean colour inversion in the Eastern Lagoon of New Caledonia, South Tropical Pacific. Remote Sens 10(7). https://doi.org/10.3390/rs10071043
Garver SA, Siegel DA (1997a) Inherent optical property inversion of ocean color spectra and its biogeochemical interpretation: 1. Time series from the Sargasso Sea. J Geophys Res Oceans 102(C8):18607–18625
Garver SA, Siegel DA (1997b) model. 102(96)
Gitelson A, Garbuzov G, Szilagyi F, Mittenzwey KH, Karnieli A, Kaiser A (1993) Quantitative remote sensing methods for real-time monitoring of inland waters quality. Int J Remote Sens 14(7):1269–1295. https://doi.org/10.1080/01431169308953956
Gordon HR (1986) Ocean color remote sensing: influence of the particle phase function and the solar zenith angle. EOS Trans Am Geophys Union 14(1055):897–912
Gordon HR, Morel AY (1983) Remote assessment of ocean color for interpretation of satellite visible imagery; a review. In: Barber RT, Mooers CNK, Bowman MJ, Zeitschel B (eds) Lecture notes on coastal and estuarine studies. Springer-Verlag, New York
Gordon HR (1993) Radiative transfer in the atmosphere for correction of ocean color remote sensors. In Ocean colour: theory and applications in a decade of CZCS experience (pp. 33–77). Springer, Dordrecht
Gordon Howard R (1997) Atmospheric correction of ocean color imagery in the Earth Observing System era. J Geophys Res Atmos 102(D14):17081–17106
Gordon Howard R, Brown OB, Evans RH, Brown JW, Smith RC, Baker KS, Clark DK (1988) A semianalytic radiance model of ocean color. J Geophys Res Atmos 93(D9):10909–10924
Hoge FE, Lyon PE (1996) Satellite retrieval of inherent optical properties by linear matrix inversion of oceanic radiance models: an analysis of model and radiance measurement errors. J Geophys Res Oceans 101(C7):16631–16648
Hooker SB, Maritorena S (2000) An evaluation of oceanographic radiometers and deployment methodologies. J Atmos Ocean Technol 17(6):811–830
Huang CC, Li YM, Wang Q, Sun DY, Le CF, Shi K (2012) Scattering spectrum properties and their relationship to biogeochemical parameters: A case study in Taihu Lake. Limnology 13(1):1–11. https://doi.org/10.1007/s10201-011-0346-4
Joshi ID, D’Sa EJ (2018) An estuarine-tuned quasi-analytical algorithm (QAA-V): assessment and application to satellite estimates of SPM in Galveston Bay following Hurricane Harvey. Biogeosciences 15(13):4065–4086
Kuchinke CP, Gordon HR, Franz BA (2009) Spectral optimization for constituent retrieval in Case 2 waters I: Implementation and performance. Remote Sens Environ 113(3):571–587
Lacava T, Ciancia E, Di Polito C, Madonia A, Pascucci S, Pergola N, Piermattei V, Satriano V, Tramutoli V (2018) Evaluation of MODIS-Aqua chlorophyll-a algorithms in the Basilicata Ionian Coastal waters. Remote Sens 10(7):1–24. https://doi.org/10.3390/rs10070987
Laliberté J, Larouche P, Devred E, Craig S (2018) Chlorophyll-a concentration retrieval in the optically complex waters of the St. Lawrence Estuary and Gulf using principal component analysis. Remote Sens 10(2):265. https://doi.org/10.3390/rs10020265
Le CF, Li YM, Zha Y, Sun D, Yin B (2009) Validation of a quasi-analytical algorithm for highly turbid eutrophic water of Meiliang Bay in Taihu Lake, China. IEEE Trans Geosci Remote Sens 47(8):2492–2500
Lee Z, Lubac B, Werdell J, Arnone R (2009) An update of the quasi-analytical algorithm (QAA_v5). International Ocean Color Group Software Report, 1–9
Lee Z, Lubac B, Werdell J, Arnone R (2014) Update of the quasi-analytical algorithm (QAA_v6)[R/OL]. Interna-Tional Ocean Color Group Software Report [2013-04-03]. Retrieved August 26, 2021 from http://www.Ioccg.Org/Groups/Software-OCA/OAA_5
Lee Z (2006) Remote sensing of inherent optical properties: fundamentals, tests of algorithms, and applications, vol 5. Reports of the International Ocean Colour Coordinating Group, IOCCG, Dartmouth, Canada
Lee ZhongPing, Arnone R, Hu C, Werdell PJ, Lubac B (2010) Uncertainties of optical parameters and their propagations in an analytical ocean color inversion algorithm. Appl Opt 49(3):369–381
Lee Z, Carder KL, Arnone RA (2002) Deriving inherent optical properties from water color: a multiband quasi-analytical algorithm for optically deep waters. Applied optics 41(27):5755–5772
Lee Zhongping, Carder KL, Mobley CD, Steward RG, Patch JS (1999) Hyperspectral remote sensing for shallow waters: 2 Deriving bottom depths and water properties by optimization. Appl Opt 38(18):3831. https://doi.org/10.1364/ao.38.003831
Lee ZhongPing, Carder KL, Steward RG, Peacock TG, Davis CO, Patch JS (1998) An empirical algorithm for light absorption by ocean water based on color. J Geophys Res Oceans 103(C12):27967–27978
Lee Z, Weidemann A, Kindle J, Arnone R, Carder KL, Davis C (2007) Euphotic zone depth: Its derivation and implication to ocean-color remote sensing. J Geophys Res Oceans 112(C3):1–11
Li J, Yu Q, Tian YQ, Becker BL (2017) Remote sensing estimation of colored dissolved organic matter (CDOM) in optically shallow waters. ISPRS J Photogramm Remote Sens 128:98–110
Li S, Song K, Mu G, Zhao Y, Ma J, Ren J (2016) Evaluation of the Quasi Analytical Algorithm (QAA) for estimating total absorption coefficient of turbid inland Waters in Northeast China. IEEE J Sel Top Appl Earth Obs Remote Sens 9(9):4022–4036
Loisel H, Stramski D (2000) Estimation of the inherent optical properties of natural waters from the irradiance attenuation coefficient and reflectance in the presence of Raman scattering. Appl Opt 39(18):3001. https://doi.org/10.1364/ao.39.003001
Lotliker AA, Baliarsingh SK, Sahu KC, Kumar TS (2015) Performance of Semianalytical Algorithm and Associated Inherent Optical Properties in Coastal Waters of North Western Bay of Bengal. J Indian Soc Remote Sens 43(1):143–149. https://doi.org/10.1007/s12524-014-0399-z
Maritorena Stéphane, d’Andon OHF, Mangin A, Siegel DA (2010) Merged satellite ocean color data products using a bio-optical model: Characteristics, benefits and issues. Remote Sens Environ 114(8):1791–1804
Maritorena Stephane, Morel A, Gentili B (1994) Diffuse reflectance of oceanic shallow waters: Influence of water depth and bottom albedo. Limnol Oceanogr 39(7):1689–1703
Maritorena Stéphane, Siegel DA (2005) Consistent merging of satellite ocean color data sets using a bio-optical model. Remote Sens Environ 94(4):429–440
Maritorena Stephane, Siegel DA, Peterson AR (2002) Optimization of a semianalytical ocean color model for global-scale applications. Appl Opt 41(15):2705–2714
McClain CR, Feldman GC, Hooker SB (2004) An overview of the SeaWiFS project and strategies for producing a climate research quality global ocean bio-optical time series. Deep-Sea Res Part II Top Stud Oceanogr 51(1–3):5–42. https://doi.org/10.1016/j.dsr2.2003.11.001
McClain M, IOCCG. (2012). Mission requirements for future ocean-colour sensors. Reports of the International Ocean-Colour Coordinating Group, No. 13.
McKinna LIW, Werdell PJ, Proctor CW (2016) Implementation of an analytical Raman scattering correction for satellite ocean-color processing. Opt Express 24(14):A1123. https://doi.org/10.1364/oe.24.0a1123
Mobley C, Boss E, Roesler C (2016) Overview of optical oceanography: Inherent optical properties. Ocean Optics Web Book. Retrieved August 26, 2021 from http://www.Oceanopticsbook.Info/View/Overview_of_optical_oceanography/InherEnt_optical_properties
Mobley CD (1994) Light and Water: Radiative Transfer in Natural Waters. Academic Press, San Diego, California, p 59
Moses WJ, Gitelson AA, Berdnikov S, Povazhnyy V (2009) Estimation of chlorophyll-a concentration in case II waters using MODIS and MERIS data—successes and challenges. Environ Res Lett 4(4):45005
Mouw CB, Greb S, Aurin D, DiGiacomo PM, Lee Z, Twardowski M, Binding C, Hu C, Ma R, Moore T, Moses W, Craig SE (2015) Aquatic color radiometry remote sensing of coastal and inland waters: Challenges and recommendations for future satellite missions. Remote Sens Environ 160:15–30. https://doi.org/10.1016/j.rse.2015.02.001
Mueller, J. L., & Fargion, G. S. (2002). Ocean optics protocols for satellite ocean color sensor validation, revision 3 (Vol. 210004). National Aeronautics and Space Administration, Goddard Space Flight Center
Mueller, J. L., Morel, A., Frouin, R., Davis, C., Arnone, R., Carder, K., Lee, Z. P., Steward, R. G., Hooker, S., & Mobley, C. D. (2003). Ocean Optics Protocols For Satellite Ocean Color Sensor Validation, Revision 4. Volume III: Radiometric Measurements and Data Analysis Protocols.
Ortega-Retuerta E, Siegel DA, Nelson NB, Duarte CM, Reche I (2010) Observations of chromophoric dissolved and detrital organic matter distribution using remote sensing in the Southern Ocean: Validation, dynamics and regulation. J Mar Syst 82(4):295–303. https://doi.org/10.1016/j.jmarsys.2010.06.004
Palmer SC, Kutser T, Hunter PD (2015) Remote sensing of inland waters: Challenges, progress and future directions. Remote Sens Environ 157:1–8
Pan H, Lyu H, Wang Y, Jin Q, Wang Q, Li Y, Fu Q (2015) An Improved Approach to Retrieve IOPs Based on a Quasi-Analytical Algorithm (QAA) for Turbid Eutrophic Inland Water. IEEE J Sel Top Appl Earth Obs Remote Sens 8(11):5177–5189. https://doi.org/10.1109/JSTARS.2015.2480102
Pitarch J, van der Woerd HJ, Brewin RJW, Zielinski O (2019) Optical properties of Forel-Ule water types deduced from 15 years of global satellite ocean color observations. Remote Sens Environ 231:111249
Qin Y, Brando VE, Dekker AG, Blondeau-Patissier D (2007) Validity of SeaDAS water constituents retrieval algorithms in Australian tropical coastal waters. Geophys Res Lett 34(21):1–4. https://doi.org/10.1029/2007GL030599
Rodrigues TWP (2017) From oligo to eutrophic inland waters: Advancements and challenges for bio-optical modeling. (Doctoral dissertation). Retrieved August 26, 2021 from https://repositorio.unesp.br/bitstream/handle/11449/150315/rodrigues_twp_dr_prud.pdf?sequence=3&isAllowed=y
Roesler CS, Perry MJ (1995) In situ phytoplankton absorption, fluorescence emission, and particulate backscattering spectra determined from reflectance. J Geophys Res Oceans 100(C7):13279–13294
Sahay A, Chauhan P, Nagamani PV, Sanwalani N, Dwivedi RM (2011) Backscattering of light by coastal waters using hyperspectral in-situ measurements: a case study off Veraval, Gujarat, India. Indian J Mar Sci 40:762–769
Salama MS, Dekker A, Su Z, Mannaerts CM, Verhoef W (2009) Deriving inherent optical properties and associated inversion-uncertainties in the Dutch Lakes. Hydrol Earth Syst Sci 13(7):1113
Salem SI, Strand MH, Higa H, Kim H, Kazuhiro K, Oki K, Oki T (2017) Evaluation of meris chlorophyll-a retrieval processors in a complex turbid lake kasumigaura over a 10-year mission. Remote Sens 9(10):1–21. https://doi.org/10.3390/rs9101022
Sathyendranath S (2000) Remote sensing of ocean colour in coastal, and other optically-complex, waters.
Shang SL, Dong Q, Hu CM, Lin G, Li YH, Shang SP (2014) On the consistency of MODIS chlorophyll $ a $ products in the northern South China Sea. Biogeosciences 11:269
Shanmugam P, Ahn Y-H, Ryu J-H, Sundarabalan B (2010) An evaluation of inversion models for retrieval of inherent optical properties from ocean color in coastal and open sea waters around Korea. J Oceanogr 66(6):815–830
Smyth TJ, Moore GF, Hirata T, Aiken J (2007) Erratum: Semianalytical model for the derivation of ocean color inherent optical properties: Description, implementation, and performance assessment (Applied Optics (2006) 45 (8116–8131)). Appl Opt 46(3):429–430. https://doi.org/10.1364/AO.46.000429
Song Q, Junwu T, Tingwei CUI (2011) Retrieval of inherent optical properties of the Yellow Sea and East China Sea using a quasi-analytical algorithm *. 29(1):33–45. https://doi.org/10.1007/s00343-011-9967-z
Spyrakos E, O’Donnell R, Hunter PD, Miller C, Scott M, Simis SGH, Neil C, Barbosa CCF, Binding CE, Bradt S, Bresciani M, Dall’Olmo G, Giardino C, Gitelson AA, Kutser T, Li L, Matsushita B, Martinez-Vicente V, Matthews MW, … Tyler AN (2018) Optical types of inland and coastal waters. Limnol Oceanogr. https://doi.org/10.1002/lno.10674
Stramski D, Bricaud A, Morel A (2001) Modeling the inherent optical properties of the ocean based on the detailed composition of the planktonic community. Appl Opt 40(18):2929. https://doi.org/10.1364/ao.40.002929
Tiwari SP, Shanmugam P (2011) An optical model for the remote sensing of coloured dissolved organic matter in coastal/ocean waters. Estuar Coast Shelf Sci 93(4):396–402
Wang P, Boss ES, Roesler C (2005) Uncertainties of inherent optical properties obtained from semianalytical inversions of ocean color. Appl Opt 44(19):4074–4085
Watanabe T, Oishi Y, Nakajima TY (2016) Characterization of surface solar-irradiance variability using cloud properties based on satellite observations. Solar Energy 140:83–92
Werdell PJ, Franz BA, Bailey SW, Feldman GC, Boss E, Brando VE, Dowell M, Hirata T, Lavender SJ, Lee Z (2013) Generalized ocean color inversion model for retrieving marine inherent optical properties. Appl Opt 52(10):2019–2037
Werdell PJ, Franz BA, Bailey SW, Feldman GC, Boss E, Brando VE, Dowell M, Hirata T, Lavender SJ, Lee Z, Loisel H, Maritorena S, Mélin F, Moore TS, Smyth TJ, Antoine D, Devred E (2019) Werdell et al. (2013) Generalized ocean color inversion model for retrieving marine inherent optical properties.pdf. Appl Opt 52(10):2019–2037
Werdell PJ, McKinna LIW, Boss E, Ackleson SG, Craig SE, Gregg WW, Lee Z, Maritorena S, Roesler CS, Rousseaux CS, Stramski D, Sullivan JM, Twardowski MS, Tzortziou M, Zhang X (2018) An overview of approaches and challenges for retrieving marine inherent optical properties from ocean color remote sensing. Prog Oceanogr 160(October 2017), 186–212. https://doi.org/10.1016/j.pocean.2018.01.001
Yang W, Matsushita B, Chen J, Yoshimura K, Fukushima T (2013) Retrieval of inherent optical properties for turbid inland waters from remote-sensing reflectance. IEEE Trans Geosci Remote Sens 51(6):3761–3773. https://doi.org/10.1109/TGRS.2012.2220147
Zhan J, Zhang DJ, Zhang GY, Wang CX, Zhou GQ (2020) Estimation of Optical Properties using QAA-V6 model based on MODIS data. Int Arch Photogramm Remote Sens Spat Inf Sci 42:937–940
Zheng G, DiGiacomo PM (2018) Detecting phytoplankton diatom fraction based on the spectral shape of satellite-derived algal light absorption coefficient. Limnol Oceanogr 63(S1):S85–S98
Acknowledgements
This study was conducted in the frame of the “Modelling of IOPs of the Arabian Gulf waters” Project. The authors thank Khalifa University in the UAE for funding this research (FSU Award 8474000241).
Author information
Authors and Affiliations
Corresponding author
Ethics declarations
Conflict of Interest
The authors declare no competing interests.
Additional information
Publisher's Note
Springer Nature remains neutral with regard to jurisdictional claims in published maps and institutional affiliations.
Rights and permissions
About this article
Cite this article
Najah, A., Al-Shehhi, M.R. Performance of the Ocean Color Algorithms: QAA, GSM, and GIOP in Inland and Coastal Waters. Remote Sens Earth Syst Sci 4, 235–248 (2021). https://doi.org/10.1007/s41976-022-00068-3
Received:
Revised:
Accepted:
Published:
Issue Date:
DOI: https://doi.org/10.1007/s41976-022-00068-3