Graph-based multi-view clustering can effectively reveal the latent cluster structure of multi-view data, however, it remains challenging to construct high-quality graphs by exploring the discriminant information from data. Most of the existing graph-based methods usually construct the graph in the original space or subspace, which may not take the differences of semantic information in different spaces into considerations. Moreover, existing approaches generally measure the relevance of the pair-wise views, which may result in insufficient information exploitation and limited graph representation. To remedy these issues, we proposed an innovative multi-view clustering algorithm called reciprocal consistency graph learning (RCGL), which learns consistency representation by aligning the semantic information from three spaces simultaneously to uncover latent complementarity and consistency among different views. Specifically, we construct the low-dimensional space based on linear projection to preserve essential semantic information. Furthermore, RCGL maps the low-dimension representation from linear space into nonlinear space via Hilbert–Schmidt independence criterion, to explore the nonlinear information hidden in data. Moreover, multiple graphs in kernel space are extended to tensor space for learning a high-order graph, which is helpful to better measure the correlation between multiple views. Finally, the objective function is solved by an alternating optimization scheme. Extensive experiments on five benchmark multi-view datasets demonstrate that RCGL outperforms state-of-the-art baselines markedly. |
ACCESS THE FULL ARTICLE
No SPIE Account? Create one
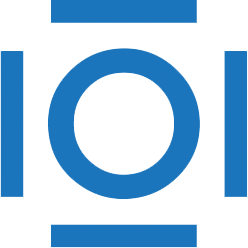
CITATIONS
Cited by 1 scholarly publication.
Matrices
Visualization
Data modeling
MATLAB
Nonlinear dynamics
Optimization (mathematics)
Information science