We propose a dual-branch Siamese network for visual object tracking. Our network architecture comprises two distinct branches: a shallow network branch and a deep network branch. The shallow network branch focuses on precise object localization and improving resistance to interference from similar objects. Meanwhile, the deep network branch emphasizes capturing abstract semantic features of the object. To enhance localization accuracy, we integrate a multi-scale KFFM into the shallow network. In addition, we leverage the attention mechanism to further enhance the model’s robustness. Through extensive experiments on three publicly available datasets, we demonstrate that our method surpasses state-of-the-art tracking algorithms in terms of performance and accuracy. The source code of this work is available online at https://github.com/mbgzwn/SiamDUL.git. |
ACCESS THE FULL ARTICLE
No SPIE Account? Create one
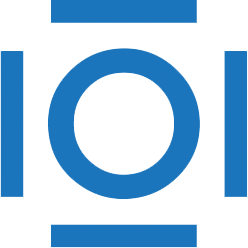
CITATIONS
Cited by 5 scholarly publications.
Detection and tracking algorithms
Feature extraction
Optical tracking
Visualization
Network architectures
Semantics
Education and training