Abstract
Autonomy offers significant advantages for mobile robots by eliminating the need for human operators, thereby enhancing safety and cost-effectiveness. Path planning is an essential component of achieving autonomy, as it empowers robots to thoughtfully navigate between different areas. This study explores the most recent developments in automated guided vehicles (AGVs) and autonomous mobile robots during the previous ten years. It encompasses a wide range of AGV research topics from both historical and contemporary perspectives. AGVs play a vital role in modern logistics networks, offering time savings and the potential to minimize wear and capital costs through efficient path planning. Numerous approaches to aid in the path-planning procedure for mobile robotics have been suggested and documented in scholarly research. While perfection is not guaranteed, these methods have demonstrated impressive efficacy in practical applications. The study evaluates models, optimization benchmarks, and solution techniques employed for charting optimal courses for mobile robots. Both field researchers and AGV developers encounter challenges in navigating the expanding array of algorithms designed for diverse applications. Digital twins emerge as pivotal tools in AGV systems, contributing to the development and implementation of control algorithms. This research aims to do a comprehensive examination of various AGV-related control strategies and cutting-edge algorithms, including those used in early models and more recent AGV systems.



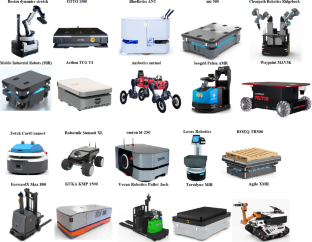
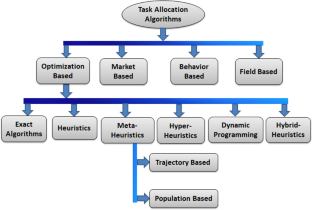

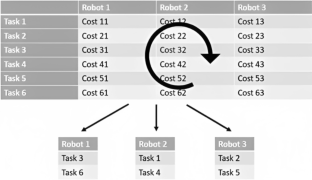

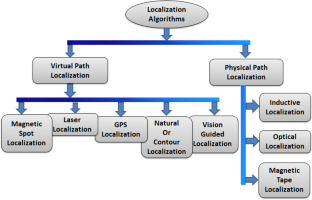


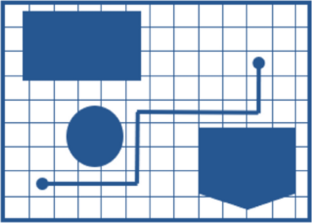
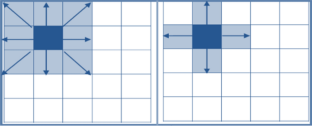
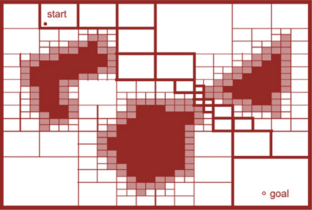




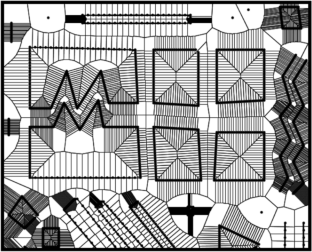


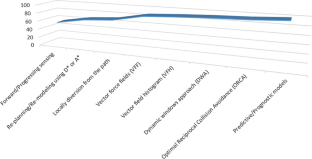
Similar content being viewed by others
References
Singholi AK, Mittal M, Bhargava A (2019) A review on IoT-based hybrid navigation system for mid-sized autonomous vehicles, advances in electromechanical technologies: select proceedings of TEMT, Springer, Singapore
Xing Y, Yang Y, Zu Q, Yu J (2019) Application of AGV technology and design and calculation of driving system. doi:https://doi.org/10.1063/1.5090682
Pedan M, Gregor M, Plinta D (2017) Implementation of automated guided vehicle system in healthcare facility. Procedia Eng 192:665–670. https://doi.org/10.1016/j.proeng.2017.06.115
Fragapane G, Hvolby H-H, Sgarbossa F, Strandhagen JO (2021) Autonomous mobile robots in sterile instrument logistics: an evaluation of the material handling system for a strategic fit framework. Prod Plan Control 34(1):53–67. https://doi.org/10.1080/09537287
Fragapane G, de Koster R, Sgarbossa F, Strandhagen JO (2021) Planning and control of autonomous mobile robots for intralogistics: literature review and research agenda. Eur J Oper Res 294(2):405–426. https://doi.org/10.1016/j.ejor.2021.01.019
Zhong M, Yang Y, Dessouky Y, Postolache O (2020) Multi-AGV scheduling for conflict-free path planning in automated container terminals. Comput Ind Eng 142:106371. https://doi.org/10.1016/j.cie.2020.106371
Li J, Ran M, Wang H, Xie L (2019) MPC-based unified trajectory planning and tracking control approach for automated guided vehicles*. In: 2019 IEEE 15th international conference on control and automation (ICCA). doi:https://doi.org/10.1109/icca.2019.8899955.
J Li, W Gu, H Wu (2015) The literature review of research on modeling of automated guided vehicle’s picking route. In: proc. SPIE 9794, sixth international conference on electronics and information engineering, 97942V, https://doi.org/10.1117/12.2205738
Herrero-Pérez D, Martínez-Barberá H (2011) Decentralized traffic control for non-holonomic flexible automated guided vehicles in industrial environments. Adv Robot 25(6–7):739–763. https://doi.org/10.1163/016918611x563283
Wang C, Mao J (2019) Summary of AGV path planning. In: 2019 3rd international conference on electronic information technology and computer engineering (EITCE). doi:https://doi.org/10.1109/eitce47263.2019.90948.
Ludwig S, Karrenbauer M, Fellan A, Schotten HD, Buhr H, Seetaraman S, Schildknecht T (2018) A5G architecture for the factory of the future. In: 2018 IEEE 23rd international conference on emerging technologies and factory automation (ETFA). doi:https://doi.org/10.1109/etfa.2018.8502642.
Li D, Tang H, Wang S et al (2017) A big data enabled load-balancing control for smart manufacturing of Industry 4.0. Cluster Comput 20:1855–1864. https://doi.org/10.1007/s10586-017-0852-1
Nakimuli W et al (2021) Deployment and evaluation of an industry 4.0 use case over 5G. IEEE Commun Mag 59(7):14–20
De Matthias R et al (2021) Decentral task allocation for industrial AGV-systems with resource constraints. J Manuf Syst 59:310–319
Quan S, Chen J (2019) AGV localization based on odometry and LiDAR. In: 2019 2nd World conference on mechanical engineering and intelligent manufacturing (WCMEIM). doi:https://doi.org/10.1109/wcmeim48965.2019.0010.
Martínez-Gutiérrez A, Díez-González J, Ferrero-Guillén R, Verde P, Álvarez R, Perez H (2021) Digital twin for automatic transportation in industry 4.0. Sensors 21:3344. https://doi.org/10.3390/s21103344
Lynch L, Newe T, Clifford J, Coleman J, Walsh J, Toal D (2018) Automated ground vehicle (AGV) and sensor technologies- A Review. In: 2018 12th international conference on sensing technology (ICST). doi:https://doi.org/10.1109/icsenst.2018.8603640
Hong-Jie T, et al (2018) PID control of magnetic navigation differential AGV trajectory. DEStech transactions on engineering and technology research, 500–506
Cui G, Bai Y, Li S (2021) AGV Research based on inertial navigation and vision fusion. In: 2021 5th CAA international conference on vehicular control and intelligence (CVCI), Tianjin, China, pp 1–6, doi: https://doi.org/10.1109/CVCI54083.2021.9661175
Lin PT, Liao C-A, Liang S-H (2021) Probabilistic indoor positioning and navigation (PIPN) of autonomous ground vehicle (AGV) based on wireless measurements. IEEE Access 9:25200–25207
Oyekanlu EA, Smith AC, Thomas WP, Mulroy G, Hitesh D, Ramsey M, Sun D (2020) A review of recent advances in automated guided vehicle technologies: integration challenges and research areas for 5G-based smart manufacturing applications. IEEE access 8:202312–202353
Kampen AL, Fojcik M, Cupek R, Stoj J (2021) The requirements for using wireless networks with AGV communication in an industry environment. In: 2021 17th international conference on wireless and mobile computing, networking and communications (WiMob) (pp 212–218). IEEE
López J, Zalama E, Gómez-García-Bermejo J (2022) A simulation and control framework for AGV based transport systems. Simul Model Pract Theory 116:102430
Zhang H, Watanabe K, Motegi K, Shiraishi Y (2019) ROS based framework for autonomous driving of AGVs. Proceedings of ICMEMIS, 4–6
Li S, Yan J, Li L (2018) Automated guided vehicle: the direction of intelligent logistics. In 2018 IEEE international conference on service operations and logistics, and informatics (SOLI) (pp 250–255). IEEE
Vancea AP, Orha I (2019) A survey in the design and control of automated guided vehicle systems. Carpathian J Electr Comput Eng 12(2):41–49
Khosiawan Y, Khalfay A, Nielsen I (2018) Scheduling unmanned aerial vehicle and automated guided vehicle operations in an indoor manufacturing environment using differential evolution-fused particle swarm optimization. Int J Adv Rob Syst 15(1):1729881417754145
Ballinger GA, Johnson RE (2015) Editors’ comments: your first AMR review. Acad Manag Rev 40(3):315–322
Dang QV, Nielsen I, Bøgh S, Bocewicz G (2013) Modelling and scheduling autonomous mobile robot for a real-world industrial application. IFAC Proc Vol 46(9):2098–2103
Abdelsalam A (2019) Efficient FPGA-based inference architectures for deep learning networks (Doctoral dissertation, Ecole Polytechnique, Montreal (Canada))
Chen Z, Tondi B, Li X, Ni R, Zhao Y, Barni M (2019) Secure detection of image manipulation by means of random feature selection. IEEE Trans Inf Forensics Secur 14(9):2454–2469
Löcklin A, Dettinger F, Artelt M, Jazdi N, Weyrich M (2022) Trajectory prediction of workers to improve AGV and AMR operation based on the manufacturing schedule. Procedia CIRP 107:283–288
Zhang J, Yang X, Wang W, Guan J, Ding L, Lee VC (2023) Automated guided vehicles and autonomous mobile robots for recognition and tracking in civil engineering. Autom Constr 146:104699
Bogh S, Jensen PG, Kristjansen M, Larsen KG, Nyman U (2022) Distributed fleet management in noisy environments via model-predictive control. In: proceedings of the international conference on automated planning and scheduling, 32, pp 565–573
Yang Y, Zhong M, Yao H, Yu F, Fu X, Postolache O (2018) Internet of things for smart ports: technologies and challenges. IEEE Instrum Meas Mag 21(1):34–43
Wang Z, Ai C, Wang Z, Ren G, Xu Q (2020) Research on AGV scheduling system based on internet of things. J Phys Conf Ser 1678(1):012085
Žerovnik J (2015) Heuristics for NP-hard optimization problems-simpler is better!? Logist Supply Chain Sustain Global Chall 6(1):1–10
Kabir QS, Suzuki Y (2019) Comparative analysis of different routing heuristics for the battery management of automated guided vehicles. Int J Prod Res 57(2):624–641
Tavakoli MM, Sajadi SM, Aghili SAS (2018) Proposing a new mathematical model and a meta-heuristic algorithm for scheduling and allocating automated guided vehicle. Int J Math Op Res 13(2):202–218
Molina D, LaTorre A, Herrera F (2018) SHADE with iterative local search for large-scale global optimization. In 2018 IEEE congress on evolutionary computation (CEC) (pp 1–8). IEEE
Hansen P, Mladenović N, Todosijević R, Hanafi S (2017) Variable neighborhood search: basics and variants. Euro J Comput Optim 5(3):423–454
Chen C, Tiong LK (2019) Using queuing theory and simulated annealing to design the facility layout in an AGV-based modular manufacturing system. Int J Prod Res 57(17):5538–5555
Qiuyun T, Hongyan S, Hengwei G, Ping W (2021) Improved particle swarm optimization algorithm for AGV path planning. IEEE Access 9:33522–33531
Chawla VK, Chanda AK, Angra S (2018) Multi-load AGVs scheduling by application of modified memetic particle swarm optimization algorithm. J Braz Soc Mech Sci Eng 40:1–13
Zhou Y, Huang N (2022) Airport AGV path optimization model based on ant colony algorithm to optimize Dijkstra algorithm in urban systems. Sustain Comput: Inform Syst 35:100716
Deemyad T, Moeller R, Sebastian A (2020) Chassis design and analysis of an autonomous ground vehicle (AGV) using genetic algorithm. In: 2020 Intermountain Engineering, Technology and Computing (IETC) (pp 1–6). IEEE
Maashi M, Kendall G, Özcan E (2015) Choice function based hyper-heuristics for multi-objective optimization. Appl Soft Comput 28:312–326
Zhang Z, Chen J, Guo Q (2021) AGVs route planning based on region-segmentation dynamic programming in smart road network systems. Sci Progr 2021:1–13
Hu Y, Wu X, Zhai J, Lou P, Qian X, Xiao H (2022) Hybrid task allocation of an AGV system for task groups of an assembly line. Appl Sci 12(21):10956
De Ryck M, Pissoort D, Holvoet T, Demeester E (2021) Decentral task allocation for industrial AGV-systems with resource constraints. J Manuf Syst 59:310–319
Verma P, Olm JM, Suarez R (2024) Traffic management of Multi-AGV systems by improved dynamic resource reservation. In: IEEE Access, vol 12, pp 19790–19805. https://doi.org/10.1109/ACCESS.2024.3362293
Xin J et al (2023) Flexible time–space network formulation and hybrid metaheuristic for conflict-free and energy-efficient path planning of automated guided vehicles. J Clean Prod 398:136472
Li M, Guo B, Zhang J, Liu J, Liu S, Yu Z, Xiang L (2021) Decentralized multi-agv task allocation based on multi-agent reinforcement learning with information potential field rewards. In: 2021 IEEE 18th international conference on mobile Ad Hoc and smart systems (MASS) (pp 482–489). IEEE
Meng J, Wang S, Jiang L, Hu Z, Xie Y (2023) Accurate and efficient self-localization of AGV relying on trusted area information in dynamic industrial scene. IEEE Trans Veh Technol 71:7148–7159
Oščádal P, Huczala D, Bém J, Krys V, Bobovský Z (2020) Smart building surveillance system as shared sensory system for localization of AGVs. Appl Sci 10(23):8452
Xu H, Li X, Wang H, Chen Y (2021) Study on the virtual localization system of AGV in printing workshop based on digital twin technology. In advances in graphic communication, printing and packaging technology and materials: proceedings of 2020 11th China academic conference on printing and packaging, Springer, Singapore, pp 548–553
Mohamad Nasyir T, Risnumawan A, A Basith Abdurrohman (2021) Fork Agv: estimation of combined magnetic sensor with vision guide applied on automatic mobile transporter for navigation and accurate positioning. In 2021 international electronics symposium (IES), pp 5–11. IEEE
Shi D, Mi H, Collins EG, Wu J (2020) An indoor low-cost and high-accuracy localization approach for AGVs. IEEE Access 8:50085–50090
Wen X, Zhang H, Li H, Wang H, Ming W, Zhang Y, Zhang L (2024) Fusion q-learning algorithm for open shop scheduling problem with AGVs. Mathematics 12:452. https://doi.org/10.3390/math12030452
Zhao X et al (2022) Analysis of UAV lidar information loss and its influence on the estimation accuracy of structural and functional traits in a meadow steppe. Ecol Indic 135:108515
Rosen DM et al (2021) Advances in inference and representation for simultaneous localization and mapping. Ann Rev Control Robot Auton Syst 4:215–242
Liu X, Wang G, Chen K (2022) High-precision vision localization system for autonomous guided vehicles in dusty industrial environments. Navig J Inst Navig 69(1):502
Mohamad Nasyir T, Risnumawan A, Basith Abdurrohman A (2021) Fork Agv: estimation of combined magnetic sensor with vision guide applied on automatic mobile transporter for navigation and accurate positioning. In: 2021 international electronics symposium (IES). IEEE
Hu X, Luo Z, Jiang W (2020) AGV localization system based on ultra-wideband and vision guidance. Electronics 9(3):448
Panjan S, Tonggoed T (2022) Design and development of autonomous guided vehicle for flexible manufacturing. In: 2022 7th international conference on robotics and automation engineering (ICRAE) (pp 60–64). IEEE
Jang J-Y, Yoon S-J, Lin C-H (2023) Automated guided vehicle (AGV) driving system using vision sensor and color code. Electronics 12(6):1415
Ito S et al (2018) Small imaging depth LIDAR and DCNN-Based localization for automated guided vehicle. Sensors 18(1):177
Jiang Z, Xu Y, Sun L (2021) A novel path tracking controller for magnetic guided AGVs. In: 2021 33rd Chinese control and decision conference (CCDC) (pp. 3292–3296). IEEE
Hyunhak C et al. (2013) Method and development of magnetic positioning device for magnetic guided vehicle. In: intelligent robotics and applications: 6th international conference, ICIRA 2013, Busan, South Korea, September 25–28, Proceedings, Part I 6. Springer, Berlin
Kurup A, Kysar S, Bos J (2020) Svm-based sensor fusion for improved terrain classification. Auton Systms: Sens Process Secur Veh Infrastruct 11415:121–128
Adam Z et al. (2021) Challenges associated with sensors and data fusion for AGV-driven smart manufacturing. Computational science–ICCS 2021: 21st international conference, Krakow, Poland, June 16–18, 2021, proceedings, Part IV. Springer, Cham
Han W et al. (2021) F-loam: fast lidar odometry and mapping. In: 2021 IEEE/RSJ international conference on intelligent robots and systems (IROS). IEEE
Wang T-C, Tong C-S, Ben-ling Xu (2020) AGV navigation analysis based on multi-sensor data fusion. Multimed Tools Appl 79:5109–5124
Vladimir P, Chugunov A, Kulikov R (2019) Investigation of impact of UWB RTLS errors on AGV positioning accuracy. In 2019 international russian automation conference (RusAutoCon), pp 1–5. IEEE
Hongfei X et al. (2019) A research on AGV integrated navigation system based on fuzzy PID adaptive kalman filter. In: 2019 IEEE 3rd advanced information management, communicates, electronic and automation control conference (IMCEC). IEEE
Andreas L et al. (2020) Trajectory prediction of humans in factories and warehouses with real-time locating systems. In: 2020 25th IEEE international conference on emerging technologies and factory automation (ETFA). Vol 1. IEEE
Oščádal P et al (2020) Smart building surveillance system as shared sensory system for localization of AGVs. Appl Sci 10(23):8452
Geng K et al (2020) Deep dual-modal traffic objects instance segmentation method using camera and lidar data for autonomous driving. Remote Sens 12(20):3274
Cai J et al (2021) Collaborative optimization of storage location assignment and path planning in robotic mobile fulfillment systems. Sustainability 13(10):5644
Fragapane G et al (2021) Planning and control of autonomous mobile robots for intralogistics: literature review and research agenda. Eur J Op Res 294(2):405–426
Chunbao W et al (2015) Path planning of automated guided vehicles based on improved A-Star algorithm. In: 2015 IEEE international conference on information and automation. IEEE
Guo Q, Zheng Z, Yue X (2017) Path-planning of automated guided vehicle based on improved Dijkstra algorithm. In: 2017 29th Chinese control and decision conference (CCDC). IEEE
Zhong M et al (2020) Multi-AGV scheduling for conflict-free path planning in automated container terminals. Comput Ind Eng 142:106371
Guney MA, Raptis IA (2021) Dynamic prioritized motion coordination of multi-AGV systems. Robot Auton Syst 139:103534
Sun Y, Fang M, Yixin S (2021) AGV path planning based on improved Dijkstra algorithm. J Phys: Conf Ser 1746(1):012052
Gao S et al (2022) Throughput evaluation of AGV transportation systems based on queueing modeling and AGV route decomposition. Proc Inst Mech Eng Part B: J Eng Manuf 237(6–7):1024–1035
Guruprasad KR, Ranjitha TD (2021) CPC algorithm: exact area coverage by a mobile robot using approximate cellular decomposition. Robotica 39(7):1141–1162
Kanoon ZE, Araji ASA, Abdullah MN (2022) Enhancement of cell decomposition path-planning algorithm for autonomous mobile robot based on an intelligent hybrid optimization method. Int J Intell Eng Syst 15(3):161–175
Huifang K, Sun J, Hu J (2020) Real-time motion planning based on layered cost map for AGV navigation. 2020 Chinese Automation Congress (CAC). IEEE
Bui H-A et al (2022) Development of navigation system for autonomous guided vehicle localization with measurement uncertainties. Vietnam J Sci Technol 60(3):513–526
Runda Z et al. (2023) Design and practical implementation of a high efficiency two-layer trajectory planning method for AGV. IEEE transactions on industrial electronics
Tao W et al (2021) A novel integrated path planning algorithm for warehouse AGVs. Chin J Electr 30(2):331–338
Chengzhen M, Dai H (2021) An obstacle avoidance method based on advanced rapidly-exploring random tree for autonomous navigation. In: 2021 IEEE intl conf on parallel & distributed processing with applications, big data & cloud computing, sustainable computing & communications, social computing & networking (ISPA/BDCloud/SocialCom/SustainCom). IEEE
Billy PLL et al. (2022) Multi-AGV's temporal memory-based RRT exploration in unknown environment. arXiv e-prints: arXiv-2207
Yuan Z et al (2020) A bi-level path planning algorithm for multi-AGV routing problem. Electronics 9(9):1351
Chen Z, Bing X (2021) AGV path planning based on improved artificial potential field method. In: 2021 IEEE international conference on power electronics, computer applications (ICPECA). IEEE
Kawabe T, Nishi T, Liu Z (2023) Flexible route planning for multiple mobile robots by combining Q-learning and graph search algorithm. Appl Sci 13(3):1879
Zhang X-j et al (2022) An effective multi-AGVs dispatching method applied to matrix manufacturing workshop. Comput Ind Eng 163:107791
Yu D et al (2022) A parallel algorithm for multi-AGV systems. J Ambient Intell Humaniz Comput 13(4):2309–2323
Zou W-Q, Pan Q-K, Fatih Tasgetiren M (2021) An effective iterated greedy algorithm for solving a multi-compartment AGV scheduling problem in a matrix manufacturing workshop. Appl Soft Comput 99:106945
Lian Y, Zhang L, Xie W, Wang K (2020) An improved heuristic path planning algorithm for minimizing energy consumption in distributed multi-AGV systems. In: 2020 International symposium on autonomous systems (ISAS), Guangzhou, China, pp 70–75. https://doi.org/10.1109/ISAS49493.2020.9378876
Ye L et al. (2022) Research summary of intelligent optimization algorithm for warehouse AGV path planning. In: LISS 2021: proceedings of the 11th international conference on logistics, informatics and service sciences. Springer, Singapore
Hu H et al (2023) Anti-conflict AGV path planning in automated container terminals based on multi-agent reinforcement learning. Int J Prod Res 61(1):65–80
Yongyang Z et al. (2023) AGV path planning for logistics warehouse by using an improved D* Lite algorithm. In: proceedings of TEPEN 2022: efficiency and performance engineering network. Springer, Cham, 1018–1027
Mugarza I, Mugarza JC (2021) A coloured Petri net-and D* Lite-based traffic controller for automated guided vehicles. Electronics 10(18):2235
Xing L et al (2020) A novel tabu search algorithm for multi-AGV routing problem. Mathematics 8(2):279
Zacharia PT, Xidias EK (2020) AGV routing and motion planning in a flexible manufacturing system using a fuzzy-based genetic algorithm. Int J Adv Manuf Technol 109:1801–1813
Demir MH, Demirok M (2023) Designs of particle-swarm-optimization-based intelligent PID controllers and DC/DC buck converters for PEM fuel-cell-powered four-wheeled automated guided vehicle. Appl Sci 13(5):2919
Yi G et al (2019) Multi-AGVs path planning based on improved ant colony algorithm. J Supercomput 75:5898–5913
Wang Z, Yaohua Wu (2023) An Ant colony optimization-simulated annealing algorithm for solving a multiload AGVs workshop scheduling problem with limited buffer capacity. Processes 11(3):861
Zhu X, Yan B, Yue Y (2021) Path planning and collision avoidance in unknown environments for USVs based on an improved D* Lite. Appl Sci 11:7863. https://doi.org/10.3390/app11177863
Chen X et al (2022) An ETCEN-based motion coordination strategy avoiding active and passive deadlocks for multi-AGV system. IEEE Trans Automa Sci Eng 20(2):1364–1377
Ma H et al (2022) Bi-Risk-RRT based efficient motion planning for autonomous ground vehicles. IEEE Trans Intell Veh 7(3):722–733
Liu L et al (2022) A new knowledge-guided multi-objective optimisation for the multi-AGV dispatching problem in dynamic production environments. Int J Prod Res 61(17):6030–6051
Nguyen PT-T et al (2021) Autonomous mobile robot navigation in sparse LiDAR feature environments. Appl Sci 11(13):5963
Cao X, Zhu M (2021) Research on global optimization method for multiple AGV collision avoidance in hybrid path. Optim Control Appl Methods 42(4):1064–1080
Yuan M-H et al (2021) Dual-resource integrated scheduling method of AGV and machine in intelligent manufacturing job shop. J Central South Univ 28(8):2423–2435
Guo K, Zhu J, Shen L (2020) An improved acceleration method based on multi-agent system for AGVs conflict-free path planning in automated terminals. IEEE Access 9:3326–3338
Wang D et al (2020) A two-stage reinforcement learning approach for multi-UAV collision avoidance under imperfect sensing. IEEE Robot Autom Lett 5(2):3098–3105
Changsheng A et al. (2021) Research on AGV navigation system based on binocular vision. In: 2021 IEEE international conference on real-time computing and robotics (RCAR). IEEE
Jiayi W, Luo Y, Tan X (2021) Path planning for automatic guided vehicles (AGVs) fusing MH-RRT with improved TEB. Actuators. Vol 10, No 12. Multidisciplinary Digital Publishing Institute
Qingqing W, Liang J, Rao Z (2020) Research and design of trackless AGV system based on global vision. In: 2020 IEEE 10th international conference on electronics information and emergency communication (ICEIEC). IEEE
Kim CY, Kim YH, Ra W-S (2019) Modified 1D virtual force field approach to moving obstacle avoidance for autonomous ground vehicles. J Electr Eng Technol 14:1367–1374
Bolbhat SS et al (2020) Intelligent obstacle avoiding AGV using vector field histogram and supervisory control. J Phys: Conf Ser 1716(1):012030
Basha A, Vijayakumar V (2018) Mobile-robot navigation through fuzzy behavioral algorithm & vector-polar histogram algorithm. Indones J Electr Eng Comput Sci 9(3):565–571
Teso-Fz-Betoño D et al (2019) Predictive dynamic window approach development with artificial neural fuzzy inference improvement. Electronics 8(9):935
Yang D et al (2022) Construction of novel self-adaptive dynamic window approach combined with fuzzy neural network in complex dynamic environments. IEEE Access 10:104375–104383
Trinh LA, Ekström M, Cürüklü B (2022) dependable navigation for multiple autonomous robots with petri nets based congestion control and dynamic obstacle avoidance. J Intell Robot Syst 104(4):69
Farooq B, Bao J, Ma Q (2020) Flow-shop predictive modeling for multi-automated guided vehicles scheduling in smart spinning cyber–physical production systems. Electronics 9(5):799
Fransen KJC, Reniers MA, van Eekelen JAWM (2022) Deadlock avoidance algorithm for AGVs on a tessellated layout. In: 2022 IEEE 18th international conference on automation science and engineering (CASE). IEEE
Priya SK (2023) Conditional petri nets to analyze and simulate automated guided vehicle system. J Pharm Negat Results 14:590–598
Yankson B (2023) Small scale IoT device privacy evaluation using Petri net modeling. Internet Things 22:100725
Jiali X (2022) Cyclic study of graph search algorithm in lower bound of ramsey number. Frontier computing: proceedings of FC 2021. Springer, Singapore, 1071–1075
Xiao H et al (2020) A collision and deadlock prevention method with traffic sequence optimization strategy for UGN-based AGVs. IEEE Access 8:209452–209470
Wu W et al (2022) Petri-net-based deadlock detection and recovery for control of interacting equipment in automated container terminals. IET Intell Transp Syst 16(6):739–753
De Matthias R et al (2022) Decentral task allocation for industrial AGV-systems with routing constraints. J Manuf Systms 62:135–144
Abi-Char, Pierre E., and Chadi Riman (2021) A collision-free path planning algorithm for non-complex ASRS using heuristic functions. In: 2021 44th international conference on telecommunications and signal processing (TSP). IEEE
Yang M et al. (2021) Research on traffic control algorithm based on multi-AGV path planning. In: 2021 IEEE international conference on systems, man, and cybernetics (SMC). IEEE
Pawar S, Kulkarni P, Koli M, Barge R, Rakshe G (2022) Design and fabrication of automated guided vehicle-a review. In: 4th International conference on communication & information processing (ICCIP) 2022. https://doi.org/10.2139/ssrn.4293594
Skapinyecz R, Landschützer C (2022) Application of freely moving AGVS in a discrete event simulation environment: a first model. Adv Logist Syst-Theor Pract 16(2):5–23
Hu H et al (2021) Self-adaptive traffic control model with behavior trees and reinforcement learning for AGV in industry 4.0. IEEE Trans Ind Inform 17(12):7968–7979
Xu R et al (2022) Dynamic spare point application based coordination strategy for multi-AGV systems in a WIP warehouse environment. IEEE Access 10:80249–80263
Xing Z et al (2022) Collision and deadlock avoidance in multi-robot systems based on glued nodes. IEEE/CAA J Autom Sin 9(7):1327–1330
Sun PZ et al (2022) AGV-based vehicle transportation in automated container terminals: a survey. IEEE Trans Intell Transp Syst 24(1):341–356
Boccia M et al (2023) The parallel AGV scheduling problem with battery constraints: a new formulation and a matheuristic approach. Eur J Op Resarch 307(2):590–603
Meziane M-A (2022) Automated guided vehicles battery management for industry 4.0. J Intell Fuzzy Syst 43(1):365–381
Lee MS, Young JJ (2022) The AGV battery swapping policy based on reinforcement learning. In: 2022 IEEE 18th international conference on automation science and engineering (CASE). IEEE
Tubis AA, Poturaj H (2022) Risk related to AGV systems—open-access literature review. Energies 15(23):8910
Maoudj A, Kouider A, Christensen ALhne (2023) The capacitated multi-AGV scheduling problem with conflicting products: model and a decentralized multi-agent approach. Robot Comput-Integr Manuf 81:102514
Harada Naoki et al. (2023) Design of distributed route based on un-utility coefficient under an uncertain transportation condition at logistics center for autonomous AGVs. Int J Mech Eng Robot Res, 12.1
Author information
Authors and Affiliations
Corresponding author
Additional information
Technical Editor: Adriano Almeida Gonçalves Siqueira.
Publisher's Note
Springer Nature remains neutral with regard to jurisdictional claims in published maps and institutional affiliations.
Rights and permissions
Springer Nature or its licensor (e.g. a society or other partner) holds exclusive rights to this article under a publishing agreement with the author(s) or other rightsholder(s); author self-archiving of the accepted manuscript version of this article is solely governed by the terms of such publishing agreement and applicable law.
About this article
Cite this article
Bhargava, A., Suhaib, M. & Singholi, A.S. A review of recent advances, techniques, and control algorithms for automated guided vehicle systems. J Braz. Soc. Mech. Sci. Eng. 46, 419 (2024). https://doi.org/10.1007/s40430-024-04896-w
Received:
Accepted:
Published:
DOI: https://doi.org/10.1007/s40430-024-04896-w