第 1/1 張圖片
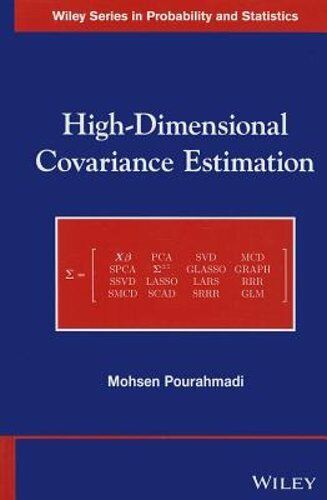
第 1/1 張圖片
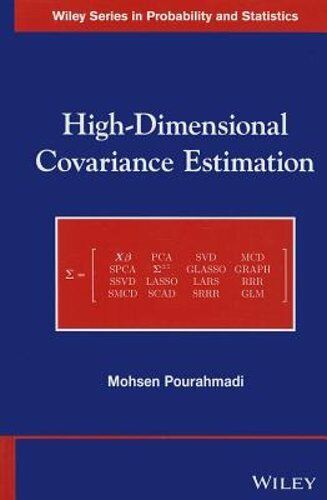
High-Dimension al Covariance Estimation: With High-Dimension al Data by Pourahmadi
US $106.18
大約HK$ 827.53
狀況:
全新
全新,未閱讀過和使用過的書籍,狀況完好,不存在缺頁或內頁受損。
運費:
所在地:Sparks, Nevada, 美國
送達日期:
估計於 9月12日, 四至 9月17日, 二之間送達 運送地點 84606
退貨:
保障:
請參閱物品說明或聯絡賣家以取得詳細資料。閱覽全部詳情查看保障詳情
(不符合「eBay 買家保障方案」資格)
安心購物
物品細節
- 物品狀況
- 全新: 全新,未閱讀過和使用過的書籍,狀況完好,不存在缺頁或內頁受損。 查看所有物品狀況定義會在新視窗或分頁中開啟
- Book Title
- High-Dimensional Covariance Estimation: With High-Dimensional Dat
- Publication Date
- 2013-08-09
- ISBN
- 9781118034293
- Subject Area
- Mathematics, Computers
- Publication Name
- High-Dimensional Covariance Estimation : with High-Dimensional Data
- Publisher
- Wiley & Sons, Incorporated, John
- Item Length
- 9.7 in
- Subject
- Probability & Statistics / General, Probability & Statistics / Multivariate Analysis, Probability & Statistics / Regression Analysis, Databases / Data Mining
- Publication Year
- 2013
- Series
- Wiley Series in Probability and Statistics Ser.
- Type
- Textbook
- Format
- Hardcover
- Language
- English
- Item Height
- 0.6 in
- Item Weight
- 15.2 Oz
- Item Width
- 6.4 in
- Number of Pages
- 208 Pages
關於產品
Product Identifiers
Publisher
Wiley & Sons, Incorporated, John
ISBN-10
1118034295
ISBN-13
9781118034293
eBay Product ID (ePID)
160004605
Product Key Features
Number of Pages
208 Pages
Publication Name
High-Dimensional Covariance Estimation : with High-Dimensional Data
Language
English
Subject
Probability & Statistics / General, Probability & Statistics / Multivariate Analysis, Probability & Statistics / Regression Analysis, Databases / Data Mining
Publication Year
2013
Type
Textbook
Subject Area
Mathematics, Computers
Series
Wiley Series in Probability and Statistics Ser.
Format
Hardcover
Dimensions
Item Height
0.6 in
Item Weight
15.2 Oz
Item Length
9.7 in
Item Width
6.4 in
Additional Product Features
Intended Audience
Scholarly & Professional
Series Volume Number
882
Illustrated
Yes
Table Of Content
Preface xi PART I MOTIVATION AND THE BASICS 1 Introduction 3 1.1 Least-Squares and Regularized Regression 4 1.2 Lasso: Survival of the Bigger 6 1.3 Thresholding the Sample Covariance Matrix 9 1.4 Sparse PCA and Regression 10 1.5 Graphical Models: Nodewise Regression 12 1.6 Cholesky Decomposition and Regression 13 1.7 The Bigger Picture: Latent Factor Models 14 1.8 Further Reading 16 2 Data, Sparsity and Regularization 21 2.1 Data Matrix: Examples 22 2.2 Shrinking the Sample Covariance Matrix 26 2.3 Distribution of the Sample Eigenvalues 29 2.4 Regularizing Covariances Like a Mean 30 2.5 The Lasso Regression 32 2.6 Lasso, Variable Selection and Prediction 36 2.7 Lasso, Degrees of Freedom and BIC 37 2.8 Some Alternatives to the Lasso Penalty 38 3 Covariance Matrices 45 3.1 Definition and Basic Properties 46 3.2 The Spectral Decomposition 49 3.3 Structured Covariance Matrices 52 3.4 Functions of a Covariance Matrix 55 3.5 PCA: The Maximum Variance Property 59 3.6 Modified Cholesky Decomposition 61 3.7 Latent Factor Models 65 3.8 GLM for Covariance Matrices 71 3.9 GLM via the Cholesky Decomposition 73 3.10 The GLM for Incomplete Longitudinal Data 76 3.11 A Data Example: Fruit Fly Mortality Rate 81 3.12 Simulating Random Correlation Matrices 85 3.13 Bayesian Analysis of Covariance Matrices 88 PART II COVARIANCE ESTIMATION: REGULARIZATION 4 Regularizing the Eigenstructure 95 4.1 Shrinking the Eigenvalues 96 4.2 Regularizing The Eigenvectors 101 4.3 A Duality between PCA and SVD 103 4.4 Implementing Sparse PCA: A Data Example 106 4.5 Sparse Singular Value Decomposition (SSVD) 108 4.6 Consistency of PCA 109 4.7 Principal Subspace Estimation 113 4.8 Further Reading 114 5 Sparse Gaussian Graphical Models 115 5.1 Covariance Selection Models: Two Examples 116 5.2 Regression Interpretation of Entries of ∑-1 118 5.3 Penalized Likelihood and Graphical Lasso 120 5.4 Penalized Quasi-Likelihood Formulation 126 5.5 Penalizing the Cholesky Factor 127 5.6 Consistency and Sparsistency 130 5.7 Joint Graphical Models 130 5.8 Further Reading 132 6 Banding, Tapering and Thresholding 135 6.1 Banding the Sample Covariance Matrix 136 6.2 Tapering the Sample Covariance Matrix 137 6.3 Thresholding the Sample Covariance Matrix 138 6.4 Low-Rank Plus Sparse Covariance Matrices 142 6.5 Further Reading 143 7 Multivariate Regression: Accounting for Correlation 145 7.1 Multivariate Regression & LS Estimators 146 7.2 Reduced Rank Regressions (RRR) 148 7.3 Regularized Estimation of B 150 7.4 Joint Regularization of (B;) 152 7.5 Implementing MRCE: Data Examples 155 7.5.1 Intraday Electricity Prices 155 7.5.2 Predicting Asset Returns 158 7.6 Further Reading 161
Synopsis
Methods for estimating sparse and large covariance matrices Covariance and correlation matrices play fundamental roles in every aspect of the analysis of multivariate data collected from a variety of fields including business and economics, health care, engineering, and environmental and physical sciences. High-Dimensional Covariance Estimation provides accessible and comprehensive coverage of the classical and modern approaches for estimating covariance matrices as well as their applications to the rapidly developing areas lying at the intersection of statistics and machine learning. Recently, the classical sample covariance methodologies have been modified and improved upon to meet the needs of statisticians and researchers dealing with large correlated datasets. High-Dimensional Covariance Estimation focuses on the methodologies based on shrinkage, thresholding, and penalized likelihood with applications to Gaussian graphical models, prediction, and mean-variance portfolio management. The book relies heavily on regression-based ideas and interpretations to connect and unify many existing methods and algorithms for the task. High-Dimensional Covariance Estimation features chapters on: Data, Sparsity, and Regularization Regularizing the Eigenstructure Banding, Tapering, and Thresholding Covariance Matrices Sparse Gaussian Graphical Models Multivariate Regression The book is an ideal resource for researchers in statistics, mathematics, business and economics, computer sciences, and engineering, as well as a useful text or supplement for graduate-level courses in multivariate analysis, covariance estimation, statistical learning, and high-dimensional data analysis., Methods for estimating sparse and large covariance matrices Covariance and correlation matrices play fundamental roles in every aspect of the analysis of multivariate data collected from a variety of fields including business and economics, health care, engineering, and environmental and physical sciences.
LC Classification Number
QA279.P68 2013
賣家提供的物品說明
運費與處理費
物品所在地:
Sparks, Nevada, 美國
運送地點
不丹, 中國, 中國台灣, 中國澳門, 中國香港, 中非共和國, 丹麥, 乍得, 也門, 亞爾及利亞, 亞美尼亞, 以色列, 伯利茲, 佛得角群島, 保加利亞, 克羅地亞共和國, 冰島, 列支敦士登, 利比里亞, 剛果共和國, 剛果民主共和國, 加拿大, 加納, 加蓬共和國, 匈牙利, 南韓, 博茨瓦納, 卡塔爾, 印尼, 印度, 危地馬拉, 厄瓜多爾, 厄立特里亞, 吉布提, 吉爾吉斯斯坦, 哈薩克斯坦, 哥倫比亞, 哥斯達黎加, 喀麥隆, 土庫曼斯坦, 土耳其, 圭亞那, 坦桑尼亞, 埃及, 埃塞俄比亞, 基里巴斯, 塔吉克斯坦, 塞內加爾, 塞拉利昂, 塞浦路斯, 塞爾維亞, 塞舌爾, 墨西哥, 多哥, 多米尼加共和國, 奧地利, 孟加拉, 安哥拉, 安圭拉島, 安提瓜和巴布達, 安道爾, 寮國, 尼加拉瓜, 尼日利亞, 尼日爾, 尼泊爾, 岡比亞, 巴哈馬, 巴基斯坦, 巴布亞新幾內亞, 巴拉圭, 巴拿馬, 巴林, 布基納法索, 布隆迪, 希臘, 幾內亞, 幾內亞比紹, 德國, 意大利, 愛沙尼亞, 愛爾蘭, 所羅門群島, 拉脫維亞, 挪威, 捷克共和國, 摩洛哥, 摩爾多瓦, 摩納哥, 文萊, 斐濟, 斯威士蘭, 斯洛伐克, 斯洛文尼亞, 斯里蘭卡, 新加坡, 新西蘭, 日本, 智利, 柬埔寨, 格林納達, 格陵蘭, 格魯吉亞, 梵蒂岡, 比利時, 毛里塔尼亞, 毛里求斯, 沙特阿拉伯, 法國, 波多黎各, 波斯尼亞和黑塞哥維那, 波蘭, 泰國, 津巴布韋, 洪都拉斯, 海地, 湯加, 澳洲, 烏干達, 烏拉圭, 烏茲別克斯坦, 牙買加, 特克斯和凱科斯群島, 特立尼達和多巴哥, 玻利維亞, 瑙魯, 瑞典, 瑞士, 瓦利斯和富圖納群島, 瓦努阿圖, 百慕達群島, 盧旺達, 盧森堡, 直布羅陀, 科威特, 科特迪瓦(象牙海岸), 秘魯, 突尼斯, 立陶宛, 約旦, 納米比亞, 羅馬尼亞, 美國, 聖基茨-尼維斯, 聖文森特和格林納丁斯, 聖盧西亞, 聖馬力諾, 肯尼亞, 芬蘭, 英國, 荷蘭, 莫桑比克, 菲律賓, 萊索托, 葡萄牙, 蒙古, 蒙特塞拉特島, 薩爾瓦多, 蘇里南, 西班牙, 西薩摩亞, 貝寧, 贊比亞, 赤道幾內亞, 越南, 開曼群島, 阿塞拜疆共和國, 阿富汗, 阿拉伯聯合酋長國, 阿曼, 阿根廷, 阿爾巴尼亞, 阿魯巴, 馬來西亞, 馬其頓, 馬拉維, 馬爾代夫, 馬耳他, 馬達加斯加, 馬里, 黎巴嫩, 黑山
排除:
APO/FPO, 伊拉克, 俄羅斯聯邦, 利比亞, 委內瑞拉, 巴巴多斯, 巴西, 帕勞, 新喀里多尼亞, 法屬圭亞那, 法屬玻里尼西亞, 烏克蘭, 瓜德羅普島, 留尼汪島, 白俄羅斯, 米克羅尼西亞, 美屬維爾京群島, 美屬薩摩亞, 關島, 阿拉斯加/夏威夷, 馬提尼克島, 馬紹爾群島
運費與處理費 | 運送地點 | 運送方式 | 運送*查看送達備註 |
---|---|---|---|
免運費 | 美國 | Standard Shipping | 估計於 9月12日, 四至 9月17日, 二之間送達 運送地點 84606 |
US $5.50(大約 HK$ 42.86) | 美國 | Expedited Shipping | 估計於 9月12日, 四至 9月14日, 六之間送達 運送地點 84606 |
處理時間 |
---|
通常會在收到所有款項後的 2 個工作日內發貨。 |
稅項 |
---|
賣家將對以下州別的買家收取銷售稅: |
物品編號 284559191776 的銷售稅
物品編號 284559191776 的銷售稅
賣家會對寄往以下各州的物品收取銷售稅:
州/省 | 銷售稅稅率 |
---|
退貨政策
收到物品後聯絡賣家的期限: | 退款方式 |
---|---|
30 日 | 退款 |
買家負責支付退貨運費。
賣家信用評價 (471,981)
- p***0 (536)- 買家留下的信用評價。過去 1 個月購買已獲認證Never got the book but had good customer service/communication and got my refund.
- m***s (942)- 買家留下的信用評價。過去 1 個月購買已獲認證A++++ ebayer. Nice transaction. Thank you
- 7***j (2688)- 買家留下的信用評價。過去 1 個月購買已獲認證Just as described. Fast shipping. Thank you!!