Picture 1 of 1
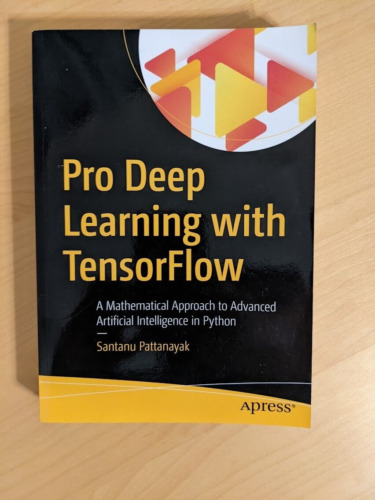
Picture 1 of 1

Pro Deep Learning with TensorFlow: A Mathematical Approach to Advanced Artificia
US $24.95
ApproximatelyS$ 32.58
Condition:
Brand New
A new, unread, unused book in perfect condition with no missing or damaged pages.
Postage:
Located in: Potomac, Maryland, United States
Delivery:
Estimated between Fri, 13 Sep and Mon, 16 Sep to 84606
Returns:
Coverage:
Read item description or contact seller for details. See all detailsSee all details on coverage
(Not eligible for eBay purchase protection programmes)
Seller assumes all responsibility for this listing.
eBay item number:325269809238
Item specifics
- Condition
- Brand New: A new, unread, unused book in perfect condition with no missing or damaged pages. See all condition definitionsopens in a new window or tab
- Book Title
- Pro Deep Learning with TensorFlow: A Mathematical Approach to Adv
- Subject
- Intelligence (Ai) & Semantics, Calculus, Databases / General, Programming Languages / Python
- Level
- Advanced
- ISBN
- 9781484230954
- Subject Area
- Mathematics, Computers
- Publication Name
- Pro Deep Learning with TensorFlow : A Mathematical Approach to Advanced Artificial Intelligence in Python
- Publisher
- Apress L. P.
- Item Length
- 10 in
- Publication Year
- 2017
- Type
- Textbook
- Format
- Trade Paperback
- Language
- English
- Item Weight
- 279.3 Oz
- Item Width
- 7 in
- Number of Pages
- Xxi, 398 Pages
About this product
Product Identifiers
Publisher
Apress L. P.
ISBN-10
1484230957
ISBN-13
9781484230954
eBay Product ID (ePID)
239660319
Product Key Features
Number of Pages
Xxi, 398 Pages
Publication Name
Pro Deep Learning with TensorFlow : A Mathematical Approach to Advanced Artificial Intelligence in Python
Language
English
Subject
Intelligence (Ai) & Semantics, Calculus, Databases / General, Programming Languages / Python
Publication Year
2017
Type
Textbook
Subject Area
Mathematics, Computers
Format
Trade Paperback
Dimensions
Item Weight
279.3 Oz
Item Length
10 in
Item Width
7 in
Additional Product Features
Number of Volumes
1 vol.
Illustrated
Yes
Table Of Content
Chapter 1: Machine Learning Basics and Mathematical Foundation for Deep Learning Chapter Goal: Introduce Machine Learning basics and Mathematical Foundations that are associated with Deep Learning No of pages 70-90 Sub-Topics 1. Linear Algebra basics. 2. Numerical Stability and Conditioning. 3. Probability. 4. Different types of cost functions and introduction to least squares and maximum likelihood methods. 5. Convex and Non-convex function 6. Optimization Techniques such as Gradient Descent and Stochastic Gradient Descent as well as Constrained Optimization problems. 7. Regularization and Early stopping 8. Auto Differentiators and Symbolic Differentiators. Chapter 2: Introduction to Deep Learning Concepts and TensorFlow Chapter Goal: Introduce Deep Learning concepts and its comparison with previous Neural Netwo rks. Reasons for its success and computational efficiency and a start to TensorFlow Development. No of pages 60-70 Sub -Topics 1. Previous Neural Networks and their shortcomings 2. Introduction to Deep Learning Framework and its advantages. 3. Why TensorFlow for Deep Learning and its comparison with other Deep Learning Frameworks like Theano, Caffe, Torch, etc. 4. Hands on in TensorFlow development environment and introduction to Dynamic Computation graphs. 5. Linear and Logistic regression in a TensorFlow environment 6. Feed forward networks through TensorFlow. 7. Leveraging GPUs for Computational efficiency. Chapter 3: Image and Audio Processing in TensorFlow through Convolutional Neural Networks Chapter Goal: Learn to process image and audio data to solve classification, clustering, and recommendation problems using Convolutional Neural Network. No of pages: 70-80 Sub - Topics: 1. Convolution and Image processing through Convolution. 2. Different Kinds of Image processing filters like Guassian Filter, Sobel Filter, Canny''s edge detection filter. 3. Different Layers of Convolutional Neural Network - Convolution layer, Pooling Layers, activation layers using RELUs, Dropout layers and fully connected layer. Intuition of features learned in Different layers. Concepts of strides, padding and kernels. 4. Solving image classification, clustering and recommendation problems through Convolutional Neural network. 5. Feature transfer in Convolutional Neural Network. 6. Audio classification problems through Convolutional Neural networks. Chapter 4: Restricted Boltzmann Deep Learning Architectures through TensorFlow for Various Problems Chapter Goal: Leverage Restricted Boltzmann Machines (R BMs) for solving Recommendation problems, weight initialization in Deep Learning Networks and for Layer by Layer training of Deep Neural Networks. No of pages:50-60 Sub - Topics: 1. Introduction to Restricted Boltzmann Machines (RBMs) and its architecture. 2. Using RBMs to build Recommendation engines. 3. RBMs for smart weight initialization of Deep Learning Networks. 4. Train complex deep learning networks layer by layer (one layer at a time) through RBMs Chapter 5: Deep Learning for Natural Language Processing through TensorFlow Chapter Goal: Leverage TensorFlow Deep learning capabilities for Natural Language processing No of pages: 50-60 1. Text processing basics such as Word2Vec Representation, Semantic and Syntactic Analysis. 2. Recurrent Neural network(RNNs) for language modelling through TensorFl ow 3. Backpropagation through time and problems of Vanishing and Exploding gradients. 4. Gradient Clipping and LSTM (Long Short-Term Memory) to overcome Exploding and Vanishing gradient problems. 5. Applications of RNN in generating sequences and words. Chapter 6: Unsupervised Learning in TensorFlow through Autoencoders Chapter Goal: Leverage Autoencoders for doing Unsupervised Learning No of pages: 30-40 1. Data Compression through Autoencoders. 2. Feature Learning through Auto Encoders. 3. A comparison of feature learning through PCA and Stacked Auto Encoders.
Synopsis
Deploy deep learning solutions in production with ease using TensorFlow. You'll also develop the mathematical understanding and intuition required to invent new deep learning architectures and solutions on your own. Pro Deep Learning with TensorFlow provides practical, hands-on expertise so you can learn deep learning from scratch and deploy meaningful deep learning solutions. This book will allow you to get up to speed quickly using TensorFlow and to optimize different deep learning architectures. All of the practical aspects of deep learning that are relevant in any industry are emphasized in this book. You will be able to use the prototypes demonstrated to build new deep learning applications. The code presented in the book is available in the form of iPython notebooks and scripts which allow you to try out examples and extend them in interesting ways. You will be equipped with the mathematical foundation and scientific knowledge to pursue research in this field and give back to the community. What You'll Learn Understand full stack deep learning using TensorFlow and gain a solid mathematical foundation for deep learning Deploy complex deep learning solutions in production using TensorFlow Carry out research on deep learning and perform experiments using TensorFlow Who This Book Is For Data scientists and machine learning professionals, software developers, graduate students, and open source enthusiasts, Deploy deep learning solutions in production with ease using TensorFlow. You'll also develop the mathematical understanding and intuition required to invent new deep learning architectures and solutions on your own. Pro Deep Learning with TensorFlow provides practical, hands-on expertise so you can learn deep learning from scratch and deploy meaningful deep learning solutions. This book will allow you to get up to speed quickly using TensorFlow and to optimize different deep learning architectures.All of the practical aspects of deep learning that are relevant in any industry are emphasized in this book. You will be able to use the prototypes demonstrated to build new deep learning applications. The code presented in the book is available in the form of iPython notebooks and scripts which allow you to try out examples and extend them in interesting ways.You will be equipped with the mathematical foundation and scientific knowledge to pursue research in this field and give back to the community. What You'll Learn Understand full stack deep learning using TensorFlow and gain a solid mathematical foundation for deep learning Deploy complex deep learning solutions in production using TensorFlow Carry out research on deep learning and perform experiments using TensorFlow Who This Book Is For Data scientists and machine learning professionals, software developers, graduate students, and open source enthusiasts
LC Classification Number
Q334-342
Item description from the seller
Seller assumes all responsibility for this listing.
eBay item number:325269809238
Postage and handling
Item location:
Potomac, Maryland, United States
Post to:
Afghanistan, Albania, Algeria, Andorra, Angola, Anguilla, Antigua and Barbuda, Argentina, Armenia, Aruba, Australia, Austria, Azerbaijan Republic, Bahamas, Bahrain, Bangladesh, Belgium, Belize, Benin, Bermuda, Bhutan, Bolivia, Bosnia and Herzegovina, Botswana, Brunei Darussalam, Bulgaria, Burkina Faso, Burundi, Cambodia, Cameroon, Canada, Cape Verde Islands, Cayman Islands, Central African Republic, Chad, Chile, China, Colombia, Costa Rica, Cyprus, Czech Republic, Côte d'Ivoire (Ivory Coast), Democratic Republic of the Congo, Denmark, Djibouti, Dominican Republic, Ecuador, Egypt, El Salvador, Equatorial Guinea, Eritrea, Estonia, Ethiopia, Fiji, Finland, France, Gabon Republic, Gambia, Georgia, Germany, Ghana, Gibraltar, Greece, Greenland, Grenada, Guatemala, Guinea, Guinea-Bissau, Guyana, Haiti, Honduras, Hong Kong, Hungary, Iceland, India, Indonesia, Ireland, Israel, Italy, Jamaica, Japan, Jordan, Kazakhstan, Kenya, Kiribati, Kuwait, Kyrgyzstan, Laos, Latvia, Lebanon, Lesotho, Liberia, Liechtenstein, Lithuania, Luxembourg, Macau, Macedonia, Madagascar, Malawi, Malaysia, Maldives, Mali, Malta, Mauritania, Mauritius, Mexico, Moldova, Monaco, Mongolia, Montenegro, Montserrat, Morocco, Mozambique, Namibia, Nauru, Nepal, Netherlands, New Zealand, Nicaragua, Niger, Nigeria, Norway, Oman, Pakistan, Panama, Papua New Guinea, Paraguay, Peru, Philippines, Poland, Portugal, Qatar, Republic of Croatia, Republic of the Congo, Romania, Rwanda, Saint Kitts-Nevis, Saint Lucia, Saint Vincent and the Grenadines, San Marino, Saudi Arabia, Senegal, Serbia, Seychelles, Sierra Leone, Singapore, Slovakia, Slovenia, Solomon Islands, South Korea, Spain, Sri Lanka, Suriname, Swaziland, Sweden, Switzerland, Taiwan, Tajikistan, Tanzania, Thailand, Togo, Tonga, Trinidad and Tobago, Tunisia, Turkey, Turkmenistan, Turks and Caicos Islands, Uganda, United Arab Emirates, United Kingdom, United States, Uruguay, Uzbekistan, Vanuatu, Vatican City State, Vietnam, Wallis and Futuna, Western Samoa, Yemen, Zambia, Zimbabwe
Excludes:
Barbados, French Guiana, French Polynesia, Guadeloupe, Libya, Martinique, New Caledonia, Reunion, Russian Federation, Ukraine, Venezuela
Postage and handling | To | Service | Delivery*See Delivery notes |
---|---|---|---|
Free postage | United States | Standard Postage (UPS Ground) | Estimated between Fri, 13 Sep and Mon, 16 Sep to 84606 |
Handling time |
---|
Will usually ship within 1 business day of receiving cleared payment. |
Taxes |
---|
Taxes may be applicable at checkout. Learn moreLearn more about paying tax on eBay purchases. |
Sales Tax for an item #325269809238
Sales Tax for an item #325269809238
Seller collects sales tax for items shipped to the following states:
State | Sales Tax Rate |
---|
Return policy
Return policy details |
---|
The seller will not accept returns for this item. |
Seller feedback (55)
- e***a (1695)- Feedback left by buyer.Past monthVerified purchasePerfect!!!!
- n***e (1345)- Feedback left by buyer.Past yearVerified purchaseGreat Box had over 160.00 in cards 2 bucks and above with 6 triomes and 3 of them were showcase boarderless.. No Ozolith n the topper was just baby Godzilla but man it was a fun Boaz thanks…
- k***m (1622)- Feedback left by buyer.Past yearVerified purchaseBox arrived as described. Thanks!
This is a private listing and your identity will not be disclosed to anyone except the seller.