
Enjoy fast, free delivery, exclusive deals, and award-winning movies & TV shows with Prime
Try Prime
and start saving today with fast, free delivery
Amazon Prime includes:
Fast, FREE Delivery is available to Prime members. To join, select "Try Amazon Prime and start saving today with Fast, FREE Delivery" below the Add to Cart button.
Amazon Prime members enjoy:- Cardmembers earn 5% Back at Amazon.com with a Prime Credit Card.
- Unlimited Free Two-Day Delivery
- Streaming of thousands of movies and TV shows with limited ads on Prime Video.
- A Kindle book to borrow for free each month - with no due dates
- Listen to over 2 million songs and hundreds of playlists
- Unlimited photo storage with anywhere access
Important: Your credit card will NOT be charged when you start your free trial or if you cancel during the trial period. If you're happy with Amazon Prime, do nothing. At the end of the free trial, your membership will automatically upgrade to a monthly membership.
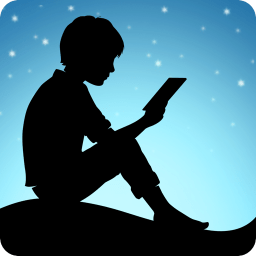
Download the free Kindle app and start reading Kindle books instantly on your smartphone, tablet, or computer - no Kindle device required.
Read instantly on your browser with Kindle for Web.
Using your mobile phone camera - scan the code below and download the Kindle app.
Deep Learning Architectures: A Mathematical Approach (Springer Series in the Data Sciences) 1st ed. 2020 Edition
Purchase options and add-ons
This book describes how neural networks operate from the mathematical point of view. As a result, neural networks can be interpreted both as function universal approximators and information processors. The book bridges the gap between ideas and concepts of neural networks, which are used nowadays at an intuitive level, and the precise modern mathematical language, presenting the best practices of the former and enjoying the robustness and elegance of the latter.
This book can be used in a graduate course in deep learning, with the first few parts being accessible to senior undergraduates. In addition, the book will be of wide interest to machine learning researchers who are interested in a theoretical understanding of the subject.
- ISBN-103030367207
- ISBN-13978-3030367206
- Edition1st ed. 2020
- PublisherSpringer
- Publication dateFebruary 14, 2020
- LanguageEnglish
- Dimensions6.5 x 1.6 x 9.3 inches
- Print length790 pages
Frequently bought together
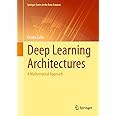
Customers who bought this item also bought
Editorial Reviews
Review
“This book is useful to students who have already had an introductory course in machine learning and are further interested to deepen their understanding of the machine learning material from the mathematical point of view.” (T. C. Mohan, zbMATH 1441.68001, 2020)
From the Back Cover
This book describes how neural networks operate from the mathematical point of view. As a result, neural networks can be interpreted both as function universal approximators and information processors. The book bridges the gap between ideas and concepts of neural networks, which are used nowadays at an intuitive level, and the precise modern mathematical language, presenting the best practices of the former and enjoying the robustness and elegance of the latter.
This book can be used in a graduate course in deep learning, with the first few parts being accessible to senior undergraduates. In addition, the book will be of wide interest to machine learning researchers who are interested in a theoretical understanding of the subject.
About the Author
Product details
- Publisher : Springer; 1st ed. 2020 edition (February 14, 2020)
- Language : English
- Hardcover : 790 pages
- ISBN-10 : 3030367207
- ISBN-13 : 978-3030367206
- Item Weight : 4.06 pounds
- Dimensions : 6.5 x 1.6 x 9.3 inches
- Best Sellers Rank: #1,829,455 in Books (See Top 100 in Books)
- #75 in Physics of Entropy
- #2,912 in Artificial Intelligence & Semantics
- #2,977 in Probability & Statistics (Books)
- Customer Reviews:
About the author

Ovidiu Calin is Professor of Mathematics and Statistics at Eastern Michigan University. He obtained his PhD. from the University of Toronto in 2000. He was a Visiting professor at Princeton University (2016-2017) and University of Notre Dame (2000-2002). His variate mathematical interests includes deep learning, mathematical finance, stochastic calculus, information theory, geometric analysis and calculus of variations.
Customer reviews
Customer Reviews, including Product Star Ratings help customers to learn more about the product and decide whether it is the right product for them.
To calculate the overall star rating and percentage breakdown by star, we don’t use a simple average. Instead, our system considers things like how recent a review is and if the reviewer bought the item on Amazon. It also analyzed reviews to verify trustworthiness.
Learn more how customers reviews work on Amazon-
Top reviews
Top reviews from the United States
There was a problem filtering reviews right now. Please try again later.
Top reviews from other countries




Reviewed in Mexico on November 6, 2020



( BTW: G. Kutyniok and P. Grohs are writing a book „Deep Learning Theory,“ which is about to appear in Cambridge University Press soon; those are two leading researchers, I have very high hopes for the book to close this gap on analytic theory )
I personally like the somewhat large font (and therefore short lines) in the book, which makes it pleasant to read, but others may disagree.
Needless to say, this is not a book for the average computer science graduate student. It’s a book for readers with curiosity who bring the necessary mathematical maturity.

