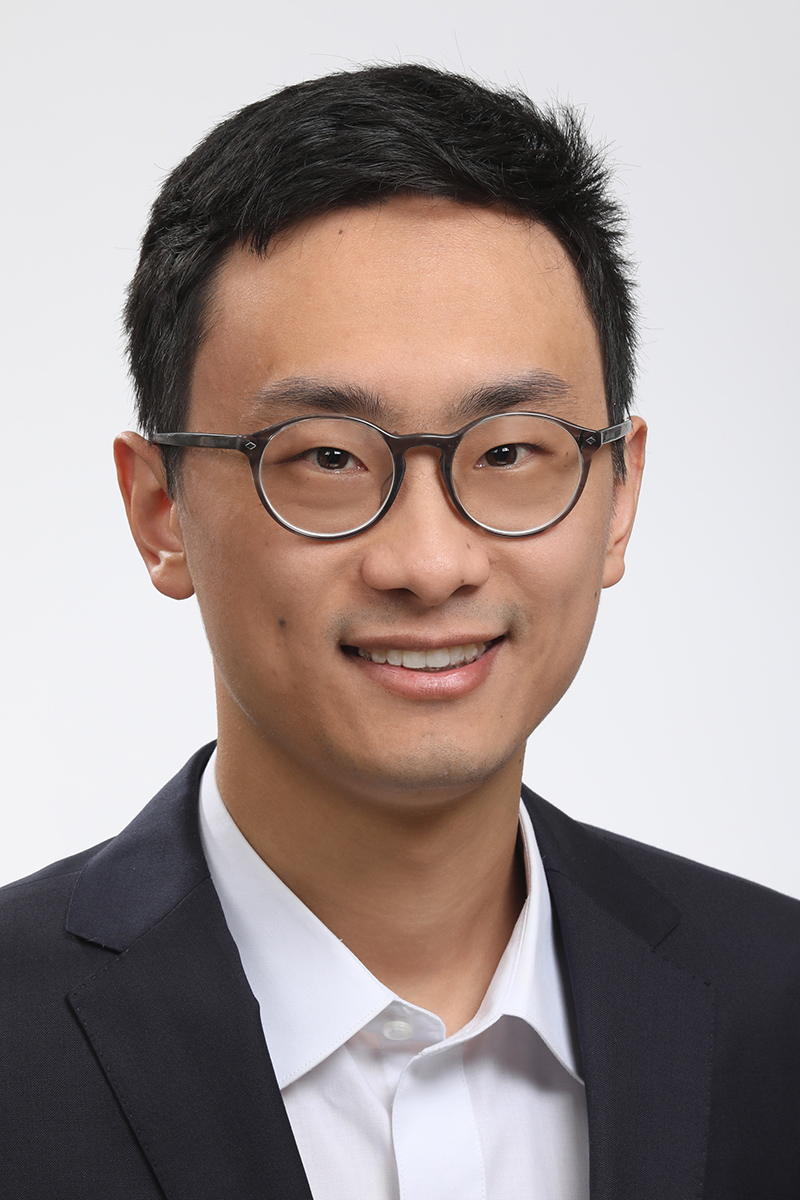
Yang Song (宋飏)
Leading Strategic Explorations team at OpenAI
Incoming Assistant Professor,
Electrical Engineering and Computing + Mathematical Sciences,
California Institute of Technology (Caltech).
Research: My goal is to build powerful AI models capable of understanding, generating and reasoning with high-dimensional data across diverse modalities. I am currently focused on developing transferable techniques to improve generative models, including their training methodologies, architecture design, alignment, robustness, evaluation techniques and inference efficiency. I am also interested in generative modeling as a tool for scientific discovery. I invented many foundational concepts and techniques in (score-based) diffusion models, for which you can find more in a blog post and a quanta magazine article.
Previously: I received my Ph.D. in Computer Science from Stanford University, advised by Stefano Ermon. I was a research intern at Google Brain, Uber ATG, and Microsoft Research. I obtained my Bachelor’s degree in Mathematics and Physics from Tsinghua University, where I worked with Jun Zhu, Raquel Urtasun, and Richard Zemel.
news
Dec 10, 2023 | The Strategic Explorations team at OpenAI is recruiting! Contact me if you are a hardcore researcher/engineer with a passion for advancing fundamental methodologies in the training and inference of diffusion models, consistency models, or large language models. |
---|---|
Dec 10, 2023 | I have postponed my starting date as an Assistant Professor at Caltech EE & CMS until January 2026. I will not take on new students, visitors, or postdocs in the interim. |