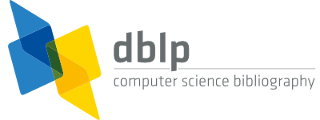


default search action
Ulrike von Luxburg
Person information
- affiliation: University of Tübingen, Germany
- affiliation (former): University of Hamburg, Germany
Refine list

refinements active!
zoomed in on ?? of ?? records
view refined list in
export refined list as
2020 – today
- 2024
- [j16]David-Elias Künstle
, Ulrike von Luxburg:
cblearn: Comparison-based Machine Learning in Python. J. Open Source Softw. 9(98): 6139 (2024) - [i41]Sebastian Bordt, Ulrike von Luxburg:
Statistics without Interpretation: A Sober Look at Explainable Machine Learning. CoRR abs/2402.02870 (2024) - [i40]Robi Bhattacharjee, Ulrike von Luxburg:
Auditing Local Explanations is Hard. CoRR abs/2407.13281 (2024) - [i39]Sebastian Bordt, Suraj Srinivas, Valentyn Boreiko, Ulrike von Luxburg:
How much can we forget about Data Contamination? CoRR abs/2410.03249 (2024) - [i38]Gunnar König, Eric Günther, Ulrike von Luxburg:
Disentangling Interactions and Dependencies in Feature Attribution. CoRR abs/2410.23772 (2024) - 2023
- [j15]Solveig Klepper, Christian Elbracht
, Diego Fioravanti, Jakob Kneip, Luca Rendsburg, Maximilian Teegen
, Ulrike von Luxburg:
Clustering with Tangles: Algorithmic Framework and Theoretical Guarantees. J. Mach. Learn. Res. 24: 190:1-190:56 (2023) - [j14]Leena Chennuru Vankadara, Michael Lohaus, Siavash Haghiri, Faiz Ul Wahab, Ulrike von Luxburg:
Insights into Ordinal Embedding Algorithms: A Systematic Evaluation. J. Mach. Learn. Res. 24: 191:1-191:83 (2023) - [j13]Solveig Klepper, Ulrike von Luxburg:
Relating graph auto-encoders to linear models. Trans. Mach. Learn. Res. 2023 (2023) - [c45]Sebastian Bordt, Ulrike von Luxburg:
From Shapley Values to Generalized Additive Models and back. AISTATS 2023: 709-745 - [c44]Sebastian Bordt, Uddeshya Upadhyay, Zeynep Akata, Ulrike von Luxburg:
The Manifold Hypothesis for Gradient-Based Explanations. CVPR Workshops 2023: 3697-3702 - [c43]Moritz Haas, David Holzmüller, Ulrike von Luxburg, Ingo Steinwart:
Mind the spikes: Benign overfitting of kernels and neural networks in fixed dimension. NeurIPS 2023 - [i37]Philipp Berens, Kyle Cranmer, Neil D. Lawrence, Ulrike von Luxburg, Jessica Montgomery:
AI for Science: An Emerging Agenda. CoRR abs/2303.04217 (2023) - [i36]Sebastian Bordt, Ulrike von Luxburg:
ChatGPT Participates in a Computer Science Exam. CoRR abs/2303.09461 (2023) - [i35]Moritz Haas, David Holzmüller, Ulrike von Luxburg, Ingo Steinwart:
Mind the spikes: Benign overfitting of kernels and neural networks in fixed dimension. CoRR abs/2305.14077 (2023) - 2022
- [c42]Luca Rendsburg, Agustinus Kristiadi, Philipp Hennig, Ulrike von Luxburg:
Discovering Inductive Bias with Gibbs Priors: A Diagnostic Tool for Approximate Bayesian Inference. AISTATS 2022: 1503-1526 - [c41]Sebastian Bordt, Ulrike von Luxburg:
A Bandit Model for Human-Machine Decision Making with Private Information and Opacity. AISTATS 2022: 7300-7319 - [c40]Sebastian Bordt, Michèle Finck, Eric Raidl
, Ulrike von Luxburg:
Post-Hoc Explanations Fail to Achieve their Purpose in Adversarial Contexts. FAccT 2022: 891-905 - [c39]Leena Chennuru Vankadara, Luca Rendsburg, Ulrike von Luxburg, Debarghya Ghoshdastidar:
Interpolation and Regularization for Causal Learning. NeurIPS 2022 - [i34]Sebastian Bordt, Michèle Finck, Eric Raidl, Ulrike von Luxburg:
Post-Hoc Explanations Fail to Achieve their Purpose in Adversarial Contexts. CoRR abs/2201.10295 (2022) - [i33]Leena Chennuru Vankadara, Luca Rendsburg, Ulrike von Luxburg, Debarghya Ghoshdastidar:
Interpolation and Regularization for Causal Learning. CoRR abs/2202.09054 (2022) - [i32]Luca Rendsburg, Agustinus Kristiadi, Philipp Hennig
, Ulrike von Luxburg:
Discovering Inductive Bias with Gibbs Priors: A Diagnostic Tool for Approximate Bayesian Inference. CoRR abs/2203.03353 (2022) - [i31]Sebastian Bordt, Uddeshya Upadhyay, Zeynep Akata, Ulrike von Luxburg:
The Manifold Hypothesis for Gradient-Based Explanations. CoRR abs/2206.07387 (2022) - [i30]Sebastian Bordt, Ulrike von Luxburg:
From Shapley Values to Generalized Additive Models and back. CoRR abs/2209.04012 (2022) - [i29]Solveig Klepper, Ulrike von Luxburg:
Relating graph auto-encoders to linear models. CoRR abs/2211.01858 (2022) - [i28]Luca Rendsburg, Leena Chennuru Vankadara, Debarghya Ghoshdastidar
, Ulrike von Luxburg:
A Consistent Estimator for Confounding Strength. CoRR abs/2211.01903 (2022) - [i27]Moritz Haas, Bedartha Goswami, Ulrike von Luxburg:
Pitfalls of Climate Network Construction: A Statistical Perspective. CoRR abs/2211.02888 (2022) - [i26]Philipp Berens, Kyle Cranmer, Neil D. Lawrence, Ulrike von Luxburg, Jessica Montgomery:
Machine Learning for Science: Bridging Data-Driven and Mechanistic Modelling (Dagstuhl Seminar 22382). Dagstuhl Reports 12(9): 150-199 (2022) - 2021
- [c38]Leena C. Vankadara, Sebastian Bordt, Ulrike von Luxburg, Debarghya Ghoshdastidar:
Recovery Guarantees for Kernel-based Clustering under Non-parametric Mixture Models. AISTATS 2021: 3817-3825 - [i25]Sascha Meyen, Frieder Göppert, Helen Alber, Ulrike von Luxburg, Volker H. Franz:
Specialists Outperform Generalists in Ensemble Classification. CoRR abs/2107.04381 (2021) - [i24]Leena C. Vankadara, Sebastian Bordt, Ulrike von Luxburg, Debarghya Ghoshdastidar:
Recovery Guarantees for Kernel-based Clustering under Non-parametric Mixture Models. CoRR abs/2110.09476 (2021) - 2020
- [c37]Damien Garreau, Ulrike von Luxburg:
Explaining the Explainer: A First Theoretical Analysis of LIME. AISTATS 2020: 1287-1296 - [c36]Michael Lohaus, Michaël Perrot, Ulrike von Luxburg:
Too Relaxed to Be Fair. ICML 2020: 6360-6369 - [c35]Luca Rendsburg, Holger Heidrich, Ulrike von Luxburg:
NetGAN without GAN: From Random Walks to Low-Rank Approximations. ICML 2020: 8073-8082 - [i23]Damien Garreau, Ulrike von Luxburg:
Explaining the Explainer: A First Theoretical Analysis of LIME. CoRR abs/2001.03447 (2020) - [i22]Christian Elbracht
, Diego Fioravanti, Solveig Klepper, Jakob Kneip, Luca Rendsburg, Maximilian Teegen
, Ulrike von Luxburg:
Tangles: From Weak to Strong Clustering. CoRR abs/2006.14444 (2020) - [i21]Sebastian Bordt, Ulrike von Luxburg:
When Humans and Machines Make Joint Decisions: A Non-Symmetric Bandit Model. CoRR abs/2007.04800 (2020) - [i20]Damien Garreau, Ulrike von Luxburg:
Looking deeper into LIME. CoRR abs/2008.11092 (2020)
2010 – 2019
- 2019
- [c34]Michaël Perrot, Ulrike von Luxburg:
Boosting for Comparison-Based Learning. IJCAI 2019: 1844-1850 - [c33]Debarghya Ghoshdastidar, Michaël Perrot, Ulrike von Luxburg:
Foundations of Comparison-Based Hierarchical Clustering. NeurIPS 2019: 7454-7464 - [i19]Siavash Haghiri, Felix A. Wichmann, Ulrike von Luxburg:
Comparison-Based Framework for Psychophysics: Lab versus Crowdsourcing. CoRR abs/1905.07234 (2019) - [i18]Michael Lohaus, Philipp Hennig, Ulrike von Luxburg:
Uncertainty Estimates for Ordinal Embeddings. CoRR abs/1906.11655 (2019) - [i17]Siavash Haghiri, Felix A. Wichmann, Ulrike von Luxburg:
Estimation of perceptual scales using ordinal embedding. CoRR abs/1908.07962 (2019) - [i16]Siavash Haghiri, Leena Chennuru Vankadara, Ulrike von Luxburg:
Large scale representation learning from triplet comparisons. CoRR abs/1912.01666 (2019) - 2018
- [j12]Nihar B. Shah, Behzad Tabibian, Krikamol Muandet, Isabelle Guyon, Ulrike von Luxburg:
Design and Analysis of the NIPS 2016 Review Process. J. Mach. Learn. Res. 19: 49:1-49:34 (2018) - [c32]Siavash Haghiri, Damien Garreau, Ulrike von Luxburg:
Comparison-Based Random Forests. ICML 2018: 1866-1875 - [c31]Cheng Tang, Damien Garreau, Ulrike von Luxburg:
When do random forests fail? NeurIPS 2018: 2987-2997 - [c30]Debarghya Ghoshdastidar, Ulrike von Luxburg:
Practical Methods for Graph Two-Sample Testing. NeurIPS 2018: 3019-3028 - [c29]Leena Chennuru Vankadara, Ulrike von Luxburg:
Measures of distortion for machine learning. NeurIPS 2018: 4891-4900 - [i15]Siavash Haghiri, Damien Garreau, Ulrike von Luxburg:
Comparison-Based Random Forests. CoRR abs/1806.06616 (2018) - [i14]Michaël Perrot, Ulrike von Luxburg:
Boosting for Comparison-Based Learning. CoRR abs/1810.13333 (2018) - [i13]Debarghya Ghoshdastidar, Michaël Perrot, Ulrike von Luxburg:
Foundations of Comparison-Based Hierarchical Clustering. CoRR abs/1811.00928 (2018) - [i12]Debarghya Ghoshdastidar, Ulrike von Luxburg:
Practical methods for graph two-sample testing. CoRR abs/1811.12752 (2018) - 2017
- [j11]Matthäus Kleindessner, Ulrike von Luxburg:
Lens Depth Function and k-Relative Neighborhood Graph: Versatile Tools for Ordinal Data Analysis. J. Mach. Learn. Res. 18: 58:1-58:52 (2017) - [c28]Siavash Haghiri, Debarghya Ghoshdastidar, Ulrike von Luxburg:
Comparison-Based Nearest Neighbor Search. AISTATS 2017: 851-859 - [c27]Debarghya Ghoshdastidar, Maurilio Gutzeit, Alexandra Carpentier, Ulrike von Luxburg:
Two-Sample Tests for Large Random Graphs Using Network Statistics. COLT 2017: 954-977 - [c26]Matthäus Kleindessner, Ulrike von Luxburg:
Kernel functions based on triplet comparisons. NIPS 2017: 6807-6817 - [e4]Isabelle Guyon, Ulrike von Luxburg, Samy Bengio, Hanna M. Wallach, Rob Fergus, S. V. N. Vishwanathan, Roman Garnett:
Advances in Neural Information Processing Systems 30: Annual Conference on Neural Information Processing Systems 2017, December 4-9, 2017, Long Beach, CA, USA. 2017 [contents] - [i11]Siavash Haghiri, Debarghya Ghoshdastidar, Ulrike von Luxburg:
Comparison Based Nearest Neighbor Search. CoRR abs/1704.01460 (2017) - [i10]Nihar B. Shah, Behzad Tabibian, Krikamol Muandet
, Isabelle Guyon, Ulrike von Luxburg:
Design and Analysis of the NIPS 2016 Review Process. CoRR abs/1708.09794 (2017) - 2016
- [j10]Tobias Lang, Florian Flachsenberg
, Ulrike von Luxburg, Matthias Rarey
:
Feasibility of Active Machine Learning for Multiclass Compound Classification. J. Chem. Inf. Model. 56(1): 12-20 (2016) - [c25]Mehdi S. M. Sajjadi, Morteza Alamgir, Ulrike von Luxburg:
Peer Grading in a Course on Algorithms and Data Structures: Machine Learning Algorithms do not Improve over Simple Baselines. L@S 2016: 369-378 - [e3]Daniel D. Lee, Masashi Sugiyama, Ulrike von Luxburg, Isabelle Guyon, Roman Garnett:
Advances in Neural Information Processing Systems 29: Annual Conference on Neural Information Processing Systems 2016, December 5-10, 2016, Barcelona, Spain. 2016 [contents] - [i9]Matthäus Kleindessner, Ulrike von Luxburg:
Lens depth function and k-relative neighborhood graph: versatile tools for ordinal data analysis. CoRR abs/1602.07194 (2016) - [i8]Matthäus Kleindessner, Ulrike von Luxburg:
Kernel functions based on triplet similarity comparisons. CoRR abs/1607.08456 (2016) - [i7]Maria-Florina Balcan, Shai Ben-David, Ruth Urner, Ulrike von Luxburg:
Foundations of Unsupervised Learning (Dagstuhl Seminar 16382). Dagstuhl Reports 6(9): 94-109 (2016) - 2015
- [c24]Matthäus Kleindessner, Ulrike von Luxburg:
Dimensionality estimation without distances. AISTATS 2015 - [i6]Mehdi S. M. Sajjadi, Morteza Alamgir, Ulrike von Luxburg:
Peer Grading in a Course on Algorithms and Data Structures: Machine Learning Algorithms do not Improve over Simple Baselines. CoRR abs/1506.00852 (2015) - 2014
- [j9]Ulrike von Luxburg, Agnes Radl, Matthias Hein:
Hitting and commute times in large random neighborhood graphs. J. Mach. Learn. Res. 15(1): 1751-1798 (2014) - [j8]Kamalika Chaudhuri, Sanjoy Dasgupta, Samory Kpotufe, Ulrike von Luxburg:
Consistent Procedures for Cluster Tree Estimation and Pruning. IEEE Trans. Inf. Theory 60(12): 7900-7912 (2014) - [c23]Matthäus Kleindessner, Ulrike von Luxburg:
Uniqueness of Ordinal Embedding. COLT 2014: 40-67 - [c22]Morteza Alamgir, Gábor Lugosi, Ulrike von Luxburg:
Density-preserving quantization with application to graph downsampling. COLT 2014: 543-559 - [c21]Yoshikazu Terada, Ulrike von Luxburg:
Local Ordinal Embedding. ICML 2014: 847-855 - [c20]Sven Kurras, Ulrike von Luxburg, Gilles Blanchard:
The f-Adjusted Graph Laplacian: a Diagonal Modification with a Geometric Interpretation. ICML 2014: 1530-1538 - 2013
- [c19]Ulrike von Luxburg, Morteza Alamgir:
Density estimation from unweighted k-nearest neighbor graphs: a roadmap. NIPS 2013: 225-233 - 2012
- [c18]Morteza Alamgir, Ulrike von Luxburg:
Shortest path distance in random k-nearest neighbor graphs. ICML 2012 - [c17]Ulrike von Luxburg, Robert C. Williamson, Isabelle Guyon:
Clustering: Science or Art? ICML Unsupervised and Transfer Learning 2012: 65-80 - 2011
- [c16]Samory Kpotufe, Ulrike von Luxburg:
Pruning nearest neighbor cluster trees. ICML 2011: 225-232 - [c15]Dario García-García, Ulrike von Luxburg, Raúl Santos-Rodríguez:
Risk-Based Generalizations of f-divergences. ICML 2011: 417-424 - [c14]Morteza Alamgir, Ulrike von Luxburg:
Phase transition in the family of p-resistances. NIPS 2011: 379-387 - [c13]Sham M. Kakade, Ulrike von Luxburg:
Preface. COLT 2011 - [e2]Sham M. Kakade, Ulrike von Luxburg:
COLT 2011 - The 24th Annual Conference on Learning Theory, June 9-11, 2011, Budapest, Hungary. JMLR Proceedings 19, JMLR.org 2011 [contents] - [r1]Ulrike von Luxburg, Bernhard Schölkopf:
Statistical Learning Theory: Models, Concepts, and Results. Inductive Logic 2011: 651-706 - [i5]Markus Maier, Ulrike von Luxburg, Matthias Hein:
How the result of graph clustering methods depends on the construction of the graph. CoRR abs/1102.2075 (2011) - [i4]Samory Kpotufe, Ulrike von Luxburg:
Pruning nearest neighbor cluster trees. CoRR abs/1105.0540 (2011) - 2010
- [c12]Morteza Alamgir, Ulrike von Luxburg:
Multi-agent Random Walks for Local Clustering on Graphs. ICDM 2010: 18-27 - [c11]Ulrike von Luxburg, Agnes Radl, Matthias Hein:
Getting lost in space: Large sample analysis of the resistance distance. NIPS 2010: 2622-2630 - [i3]Ulrike von Luxburg, Agnes Radl, Matthias Hein:
Hitting times, commute distances and the spectral gap for large random geometric graphs. CoRR abs/1003.1266 (2010)
2000 – 2009
- 2009
- [j7]Ulrike von Luxburg:
Clustering Stability: An Overview. Found. Trends Mach. Learn. 2(3): 235-274 (2009) - [j6]Sébastien Bubeck, Ulrike von Luxburg:
Nearest Neighbor Clustering: A Baseline Method for Consistent Clustering with Arbitrary Objective Functions. J. Mach. Learn. Res. 10: 657-698 (2009) - [j5]Markus Maier, Matthias Hein, Ulrike von Luxburg:
Optimal construction of k-nearest-neighbor graphs for identifying noisy clusters. Theor. Comput. Sci. 410(19): 1749-1764 (2009) - [c10]Stefanie Jegelka, Arthur Gretton
, Bernhard Schölkopf, Bharath K. Sriperumbudur, Ulrike von Luxburg:
Generalized Clustering via Kernel Embeddings. KI 2009: 144-152 - 2008
- [c9]Shai Ben-David, Ulrike von Luxburg:
Relating Clustering Stability to Properties of Cluster Boundaries. COLT 2008: 379-390 - [c8]Markus Maier, Ulrike von Luxburg, Matthias Hein:
Influence of graph construction on graph-based clustering measures. NIPS 2008: 1025-1032 - 2007
- [j4]Matthias Hein, Jean-Yves Audibert, Ulrike von Luxburg:
Graph Laplacians and their Convergence on Random Neighborhood Graphs. J. Mach. Learn. Res. 8: 1325-1368 (2007) - [j3]Ulrike von Luxburg:
A tutorial on spectral clustering. Stat. Comput. 17(4): 395-416 (2007) - [c7]Markus Maier, Matthias Hein, Ulrike von Luxburg:
Cluster Identification in Nearest-Neighbor Graphs. ALT 2007: 196-210 - [c6]Ulrike von Luxburg, Sébastien Bubeck, Stefanie Jegelka, Michael Kaufmann:
Consistent Minimization of Clustering Objective Functions. NIPS 2007: 961-968 - [i2]Ulrike von Luxburg:
A Tutorial on Spectral Clustering. CoRR abs/0711.0189 (2007) - 2006
- [c5]Shai Ben-David, Ulrike von Luxburg, Dávid Pál:
A Sober Look at Clustering Stability. COLT 2006: 5-19 - [i1]Matthias Hein, Jean-Yves Audibert, Ulrike von Luxburg:
Graph Laplacians and their convergence on random neighborhood graphs. CoRR abs/math/0608522 (2006) - 2005
- [c4]Matthias Hein, Jean-Yves Audibert, Ulrike von Luxburg:
From Graphs to Manifolds - Weak and Strong Pointwise Consistency of Graph Laplacians. COLT 2005: 470-485 - 2004
- [b1]Ulrike von Luxburg:
Statistical learning with similarity and dissimilarity functions. Technical University of Berlin, Germany, Logos-Verlag 2004, ISBN 3-8325-0767-1, pp. 1-166 - [j2]Ulrike von Luxburg, Olivier Bousquet, Bernhard Schölkopf:
A Compression Approach to Support Vector Model Selection. J. Mach. Learn. Res. 5: 293-323 (2004) - [j1]Ulrike von Luxburg, Olivier Bousquet:
Distance-Based Classification with Lipschitz Functions. J. Mach. Learn. Res. 5: 669-695 (2004) - [c3]Ulrike von Luxburg, Olivier Bousquet, Mikhail Belkin:
On the Convergence of Spectral Clustering on Random Samples: The Normalized Case. COLT 2004: 457-471 - [c2]Ulrike von Luxburg, Olivier Bousquet, Mikhail Belkin:
Limits of Spectral Clustering. NIPS 2004: 857-864 - [e1]Olivier Bousquet, Ulrike von Luxburg, Gunnar Rätsch
:
Advanced Lectures on Machine Learning, ML Summer Schools 2003, Canberra, Australia, February 2-14, 2003, Tübingen, Germany, August 4-16, 2003, Revised Lectures. Lecture Notes in Computer Science 3176, Springer 2004, ISBN 3-540-23122-6 [contents] - 2003
- [c1]Ulrike von Luxburg, Olivier Bousquet:
Distance-Based Classification with Lipschitz Functions. COLT 2003: 314-328
Coauthor Index

manage site settings
To protect your privacy, all features that rely on external API calls from your browser are turned off by default. You need to opt-in for them to become active. All settings here will be stored as cookies with your web browser. For more information see our F.A.Q.
Unpaywalled article links
Add open access links from to the list of external document links (if available).
Privacy notice: By enabling the option above, your browser will contact the API of unpaywall.org to load hyperlinks to open access articles. Although we do not have any reason to believe that your call will be tracked, we do not have any control over how the remote server uses your data. So please proceed with care and consider checking the Unpaywall privacy policy.
Archived links via Wayback Machine
For web page which are no longer available, try to retrieve content from the of the Internet Archive (if available).
Privacy notice: By enabling the option above, your browser will contact the API of archive.org to check for archived content of web pages that are no longer available. Although we do not have any reason to believe that your call will be tracked, we do not have any control over how the remote server uses your data. So please proceed with care and consider checking the Internet Archive privacy policy.
Reference lists
Add a list of references from ,
, and
to record detail pages.
load references from crossref.org and opencitations.net
Privacy notice: By enabling the option above, your browser will contact the APIs of crossref.org, opencitations.net, and semanticscholar.org to load article reference information. Although we do not have any reason to believe that your call will be tracked, we do not have any control over how the remote server uses your data. So please proceed with care and consider checking the Crossref privacy policy and the OpenCitations privacy policy, as well as the AI2 Privacy Policy covering Semantic Scholar.
Citation data
Add a list of citing articles from and
to record detail pages.
load citations from opencitations.net
Privacy notice: By enabling the option above, your browser will contact the API of opencitations.net and semanticscholar.org to load citation information. Although we do not have any reason to believe that your call will be tracked, we do not have any control over how the remote server uses your data. So please proceed with care and consider checking the OpenCitations privacy policy as well as the AI2 Privacy Policy covering Semantic Scholar.
OpenAlex data
Load additional information about publications from .
Privacy notice: By enabling the option above, your browser will contact the API of openalex.org to load additional information. Although we do not have any reason to believe that your call will be tracked, we do not have any control over how the remote server uses your data. So please proceed with care and consider checking the information given by OpenAlex.
last updated on 2024-12-02 21:30 CET by the dblp team
all metadata released as open data under CC0 1.0 license
see also: Terms of Use | Privacy Policy | Imprint