Abstract
In order that such adverse factors as complex background, low contrast between the background and overhead ground wire and blurred edges of the wire in on-site environment of hand-eye vision can be overcome, an object segmentation method based on straight lines clustering (SLC) is designed by improving the segmentation algorithm based on pixel clustering, so that pose parameters of ground wire can be detected from images. After edge detection of the images is completed, the edges are divided into straight lines with certain straightness. The lines are detected and such data as normal angles θ, distances d to the image origin and the endpoint coordinates are acquired. Then the parameter pairs (d, θ) are clustered as points on plane dθ, and the lines with similar parameter pairs are classified into the same category. For each category, outer lines are eliminated, and the remaining lines are used to determine its segmentation region, at the same time, the pose parameters of it are obtained. For the segmented objects, their local binary pattern features are extracted and SVM classifier is used to determine whether they are ground wire. The test result shows that the detection accuracy of the pose parameters of the wire can reach 90.8% and the proposed method is effective.



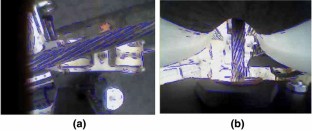






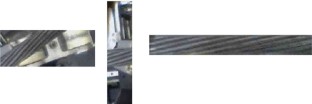


Similar content being viewed by others
References
Park, J.H., Yoo, H.R., Kim, D.K., et al.: Development of RFECT system for in-line inspection robot considering extendibility of receiving sensors based on parallel lock-in amplifier. Int. J. Precis. Eng. Manuf. 18, 145–153 (2017)
Guo, P.L., Mao, W.S., Hang, M., et al.: Design of an inspection robot with surmounting all obstacles ability for overhead transmission lines. Chin. J. Eng. Des. 23, 481–488 (2016)
Cao, W.M.: Research on visual control methods of high voltage transmission line deicing robot. Ph.D. dissertation, School of Control Science and Engineering, Hunan University, Changsha, China (2014).
Wang, L.D., Wang, H.G., Fang, L.J., et al.: Visual servo based line grasping control for power transmission line inspection robot. Robot 29, 451–455 (2007)
Wang, C., Sun, W.: Line-grasping control for the deicing robot on high voltage transmission line. J. Mech. Eng. 47, 8–15 (2011)
Guo, W.B., Wang, H.G., Jiang, Y., et al.: Visual servo control for automatic line-grasping of a power transmission line inspection robot. Robot 34, 620–627 (2012)
Hyunho, L., Changhwan, K.: Vision-based automatic real time inspection of power transmission line. In: 44th International Symposium on Robotics, Seoul, pp. 1–3 (2013)
Li, Z.H., Wang, H.G., Wang, Y.C., et al.: Line-grasping control for a power transmission line inspection robot. J. Jilin Univ. 45, 1519–1526 (2015)
Song, Y., Wang, H., Zhang, J.: A vision-based broken strand detection method for a power-line maintenance robot. IEEE Trans. Power Deliv. 29, 2154–2161 (2014)
Ye, X.H., Wu, G.P., Fan, F., et al.: Overhead ground wire detection by fusion global and local features and supervised learning method for a cable inspection robot. Sens. Rev. 38, 376–386 (2018)
Chen, Y., Zhao, T.: Balance adjustment of power-line inspection robot using general type-2 fractional order fuzzy PID controller. Symmetry 12, 479–499 (2020)
Wang, W., Wu, G.P., Bai, Y.C., et al.: Hand-eye-vision based control for an inspection robot’s autonomous line grasping. J. Cent. South Univ. 21, 2216–2227 (2014)
Otsu, N.: A threshold selection method from gray-level histograms. IEEE Trans. Syst. Man Cybern. 9, 62–66 (1979)
Adams, R., Bischof, L.: Seeded region growing. IEEE Trans. Pattern Anal. Mach. Intell. 16, 641–647 (1994)
Liu, X., Dai, J.: A method of video shot-boundary detection based on grey modeling for histogram sequence. IJSIP. 9, 265–280 (2016)
Jiang, F., Gu, Q., Hao, H.Z., et al.: Survey on content-based image segmentation methods. J. Softw. 28, 160–183 (2017)
Liu, G.C., Zhang, Y., Huang, J.H., et al.: A method for image segmentation and recognition of spider mites based on K-means clustering algorithm. Acta Entomol. Sin. 58, 1338–1343 (2015)
Yang, H.Y., Zhang, X.J., Wang, X.Y.: LS-SVM-based image segmentation using pixel color-texture descriptors. Pattern Anal. Appl. 17, 341–359 (2014)
Guo, S., Pridmore, T.P., Kong, Y., et al.: An improved Hough transform voting scheme utilizing surround suppression. Pattern Recogn. Lett. 30, 1241–1252 (2009)
Han, J.F., Song, L.L.: An improved thinning algorithm for character image. J. Comput.-Aided Des. Comput. Graph. 25, 62–66 (2013)
Lam, L., Lee, S.W., Suen, C.Y.: Thinning methodologies—a comprehensive survey. IEEE Trans. Pattern Anal. Mach. Intell. 14, 869–885 (2002)
Carmonapoyato, A., Madridcuevas, F.J., Medinacarnicer, R., et al.: Polygonal approximation of digital planar curves through break point suppression. Pattern Recogn. 41, 14–25 (2010)
Zhang, W., Hua, X., Yu, K., et al.: A novel WiFi indoor positioning strategy based on weighted squared Euclidean distance and local principal gradient direction. Sensor Rev. 39, 99–106 (2019)
Fu, L.W., Wu, S.: A new internal clustering validation index for categorical data based on concentration of attribute values. Chin. J. Eng. 41, 682–693 (2019)
Zhou, Z.H.: Machine Learning, pp. 28–33. Tsinghua University Press, Beijing, China (2016)
Ojala, T., Pietikäinen, M., Mäenpää, T.: Multiresolution gray-scale and rotation invariant texture classification with local binary patterns. IEEE Trans. Pattern Anal. Mach. Intell. 24, 971–987 (2002)
Khammari, M.: Robust face antispoofing using CNN with LBP and WLD. IET Image Process. 13, 1880–1884 (2019)
Acknowledgements
This research was funded by the National Natural Science Foundation of China (Grant No. 61976226).
Author information
Authors and Affiliations
Corresponding author
Additional information
Publisher's Note
Springer Nature remains neutral with regard to jurisdictional claims in published maps and institutional affiliations.
Rights and permissions
About this article
Cite this article
Cheng, L., Yao, W. Detection for overhead ground wire by lines clustering. SIViP 16, 447–455 (2022). https://doi.org/10.1007/s11760-021-01967-6
Received:
Revised:
Accepted:
Published:
Issue Date:
DOI: https://doi.org/10.1007/s11760-021-01967-6