Anomalous objects detection for hyperspectral imagery is a significant branch in the area of remote sensing. Although enormous advancements have been developed, issues of redundancy of spectral information and correlation between pixels should be further explored and improved. To address these problems, we proposed a method that is on the basis of integrating collaborative representation with multipurification processing and local salient weight. Multipurification processing consists of spectral bands purification (SBP) and background purification (BGP). First, to alleviate the interference of redundant spectral information, we remove unnecessary spectral bands by adopting SBP based on considering the global spectral intensity of each band. Then, we remove the outliers in the local dual window by BGP to avoid the effect of heterogeneous pixels. Simultaneously, we obtain the local salient weight by calculating the similarity and difference of pixels in the dual window. Next, we obtain the initial detection result by a collaborative representation, which has been testified to be very effective. Finally, combined with the local salient weight map, the initial detection map is improved to the final detection map. To demonstrate the superiority of the proposed method, we conducted the comprehensive experiment on three public benchmark datasets that contain 15 hyperspectral images. |
ACCESS THE FULL ARTICLE
No SPIE Account? Create one
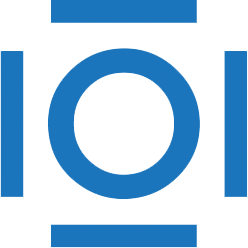
CITATIONS
Cited by 3 scholarly publications.
Sensors
Hyperspectral imaging
Visualization
Databases
Remote sensing
Statistical analysis
Target detection