|
ACCESS THE FULL ARTICLE
No SPIE Account? Create one
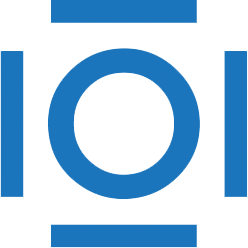
CITATIONS
Cited by 1 scholarly publication.
Performance modeling
Electroluminescent displays
Analytical research
Image analysis
Image segmentation
Data modeling
Digital imaging