|
ACCESS THE FULL ARTICLE
No SPIE Account? Create one
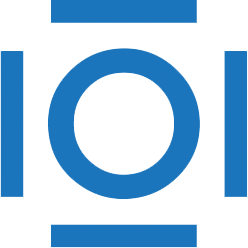
CITATIONS
Cited by 6 scholarly publications.
Lung
Image segmentation
Computed tomography
Lung cancer
Neural networks
Image processing
3D image processing