|
ACCESS THE FULL ARTICLE
No SPIE Account? Create one
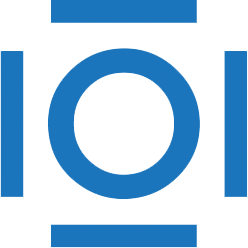
CITATIONS
Cited by 1 scholarly publication.
Data analysis
Hyperspectral imaging
Image processing
Matrix multiplication
Minerals
Image compression
Monte Carlo methods