|
ACCESS THE FULL ARTICLE
No SPIE Account? Create one
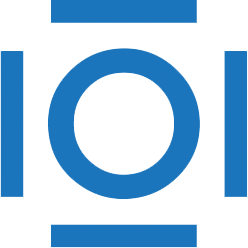
CITATIONS
Cited by 7 scholarly publications and 1 patent.
Image segmentation
Digital mammography
Mammography
Tumor growth modeling
Breast cancer
Computer-aided diagnosis