Embodied Laser Attack:Leveraging Scene Priors to Achieve Agent-based Robust Non-contact Attacks
Abstract
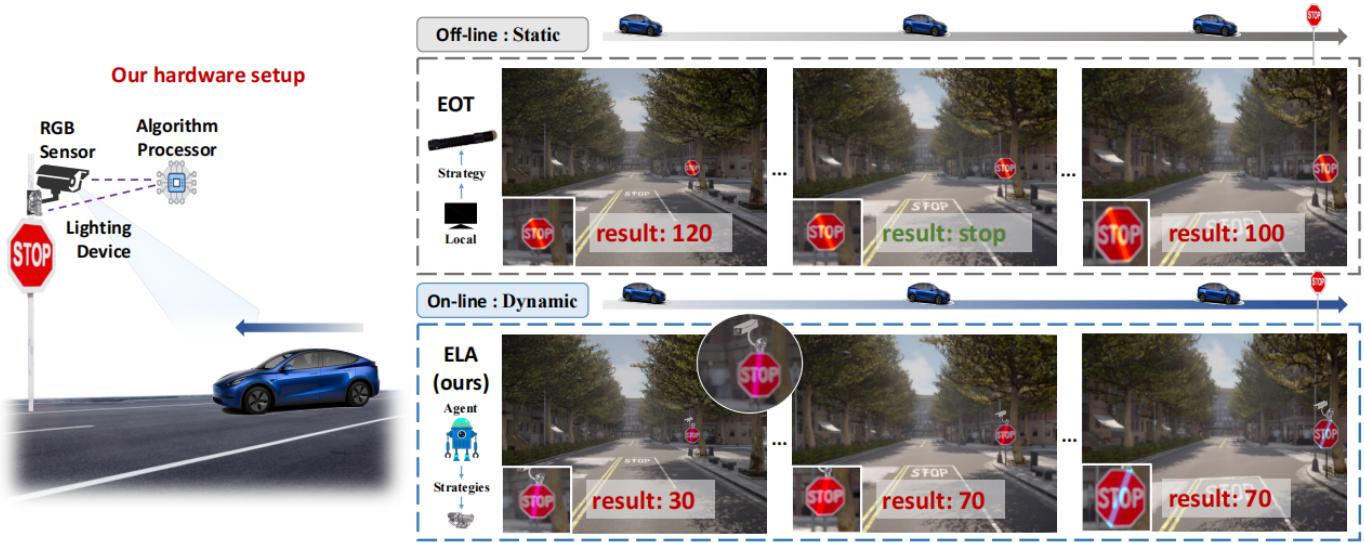
References
Index Terms
- Embodied Laser Attack:Leveraging Scene Priors to Achieve Agent-based Robust Non-contact Attacks
Recommendations
Robust profiled attacks: should the adversary trust the dataset?
Side‐channel attacks provide tools to analyse the degree of resilience of a cryptographic device against adversaries measuring leakages (e.g. power traces) on the target device executing cryptographic algorithms. In 2002, Chari et al. introduced template ...
Laser Shield: a Physical Defense with Polarizer against Laser Attacks on Autonomous Driving Systems
DAC '24: Proceedings of the 61st ACM/IEEE Design Automation ConferenceAutonomous driving systems (ADS) are boosted with deep neural networks (DNN) to perceive environments, while their security is doubted by DNN's vulnerability to adversarial attacks. Among them, a diversity of laser attacks emerges to be a new threat due ...
Defending against flooding-based distributed denial-of-service attacks: a tutorial
Flooding-based distributed denial-of-service (DDoS) attack presents a very serious threat to the stability of the Internet. In a typical DDoS attack, a large number of compromised hosts are amassed to send useless packets to jam a victim, or its ...
Comments
Information & Contributors
Information
Published In

- General Chairs:
- Jianfei Cai,
- Mohan Kankanhalli,
- Balakrishnan Prabhakaran,
- Susanne Boll,
- Program Chairs:
- Ramanathan Subramanian,
- Liang Zheng,
- Vivek K. Singh,
- Pablo Cesar,
- Lexing Xie,
- Dong Xu
Sponsors
Publisher
Association for Computing Machinery
New York, NY, United States
Publication History
Check for updates
Author Tags
Qualifiers
- Research-article
Funding Sources
Conference
Acceptance Rates
Contributors
Other Metrics
Bibliometrics & Citations
Bibliometrics
Article Metrics
- 0Total Citations
- 147Total Downloads
- Downloads (Last 12 months)147
- Downloads (Last 6 weeks)92
Other Metrics
Citations
View Options
Login options
Check if you have access through your login credentials or your institution to get full access on this article.
Sign in