Photo 1 sur 1
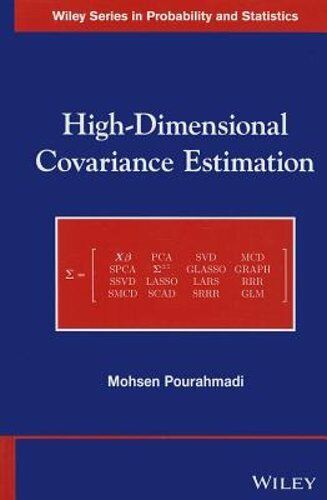
Galerie
Photo 1 sur 1
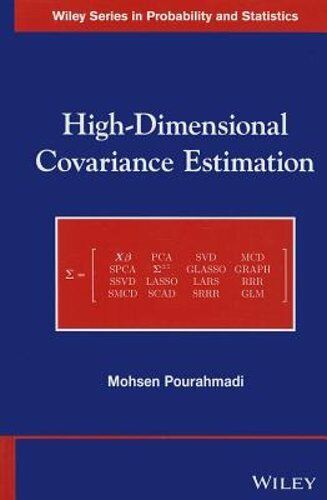
Estimation de covariance haute dimension : avec données haute dimension par Pourahmadi
106,18 $US
Environ146,06 $C
État :
Entièrement neuf
Un livre neuf, non lu, non utilisé et en parfait état, sans aucune page manquante ni endommagée.
Expédition :
Sans frais Standard Shipping.
Lieu : Sparks, Nevada, États-Unis
Livraison :
Livraison prévue entre le mer. 16 oct. et le lun. 21 oct. à 84606
Renvois :
Renvoi sous 30jours. L'acheteur paie les frais de renvoi.
Paiements :
Magasinez en toute confiance
Le vendeur assume l'entière responsabilité de cette annonce.
Numéro de l'objet eBay :284559191776
Dernière mise à jour : août 20, 2024 08:05:26 HAEAfficher toutes les modificationsAfficher toutes les modifications
Caractéristiques de l'objet
- État
- Book Title
- High-Dimensional Covariance Estimation: With High-Dimensional Dat
- Publication Date
- 2013-08-09
- ISBN
- 9781118034293
- Subject Area
- Mathematics, Computers
- Publication Name
- High-Dimensional Covariance Estimation : with High-Dimensional Data
- Publisher
- Wiley & Sons, Incorporated, John
- Item Length
- 9.7 in
- Subject
- Probability & Statistics / General, Probability & Statistics / Multivariate Analysis, Probability & Statistics / Regression Analysis, Databases / Data Mining
- Publication Year
- 2013
- Series
- Wiley Series in Probability and Statistics Ser.
- Type
- Textbook
- Format
- Hardcover
- Language
- English
- Item Height
- 0.6 in
- Item Weight
- 15.2 Oz
- Item Width
- 6.4 in
- Number of Pages
- 208 Pages
À propos de ce produit
Product Identifiers
Publisher
Wiley & Sons, Incorporated, John
ISBN-10
1118034295
ISBN-13
9781118034293
eBay Product ID (ePID)
160004605
Product Key Features
Number of Pages
208 Pages
Publication Name
High-Dimensional Covariance Estimation : with High-Dimensional Data
Language
English
Subject
Probability & Statistics / General, Probability & Statistics / Multivariate Analysis, Probability & Statistics / Regression Analysis, Databases / Data Mining
Publication Year
2013
Type
Textbook
Subject Area
Mathematics, Computers
Series
Wiley Series in Probability and Statistics Ser.
Format
Hardcover
Dimensions
Item Height
0.6 in
Item Weight
15.2 Oz
Item Length
9.7 in
Item Width
6.4 in
Additional Product Features
Intended Audience
Scholarly & Professional
Series Volume Number
882
Illustrated
Yes
Table Of Content
Preface xi PART I MOTIVATION AND THE BASICS 1 Introduction 3 1.1 Least-Squares and Regularized Regression 4 1.2 Lasso: Survival of the Bigger 6 1.3 Thresholding the Sample Covariance Matrix 9 1.4 Sparse PCA and Regression 10 1.5 Graphical Models: Nodewise Regression 12 1.6 Cholesky Decomposition and Regression 13 1.7 The Bigger Picture: Latent Factor Models 14 1.8 Further Reading 16 2 Data, Sparsity and Regularization 21 2.1 Data Matrix: Examples 22 2.2 Shrinking the Sample Covariance Matrix 26 2.3 Distribution of the Sample Eigenvalues 29 2.4 Regularizing Covariances Like a Mean 30 2.5 The Lasso Regression 32 2.6 Lasso, Variable Selection and Prediction 36 2.7 Lasso, Degrees of Freedom and BIC 37 2.8 Some Alternatives to the Lasso Penalty 38 3 Covariance Matrices 45 3.1 Definition and Basic Properties 46 3.2 The Spectral Decomposition 49 3.3 Structured Covariance Matrices 52 3.4 Functions of a Covariance Matrix 55 3.5 PCA: The Maximum Variance Property 59 3.6 Modified Cholesky Decomposition 61 3.7 Latent Factor Models 65 3.8 GLM for Covariance Matrices 71 3.9 GLM via the Cholesky Decomposition 73 3.10 The GLM for Incomplete Longitudinal Data 76 3.11 A Data Example: Fruit Fly Mortality Rate 81 3.12 Simulating Random Correlation Matrices 85 3.13 Bayesian Analysis of Covariance Matrices 88 PART II COVARIANCE ESTIMATION: REGULARIZATION 4 Regularizing the Eigenstructure 95 4.1 Shrinking the Eigenvalues 96 4.2 Regularizing The Eigenvectors 101 4.3 A Duality between PCA and SVD 103 4.4 Implementing Sparse PCA: A Data Example 106 4.5 Sparse Singular Value Decomposition (SSVD) 108 4.6 Consistency of PCA 109 4.7 Principal Subspace Estimation 113 4.8 Further Reading 114 5 Sparse Gaussian Graphical Models 115 5.1 Covariance Selection Models: Two Examples 116 5.2 Regression Interpretation of Entries of ∑-1 118 5.3 Penalized Likelihood and Graphical Lasso 120 5.4 Penalized Quasi-Likelihood Formulation 126 5.5 Penalizing the Cholesky Factor 127 5.6 Consistency and Sparsistency 130 5.7 Joint Graphical Models 130 5.8 Further Reading 132 6 Banding, Tapering and Thresholding 135 6.1 Banding the Sample Covariance Matrix 136 6.2 Tapering the Sample Covariance Matrix 137 6.3 Thresholding the Sample Covariance Matrix 138 6.4 Low-Rank Plus Sparse Covariance Matrices 142 6.5 Further Reading 143 7 Multivariate Regression: Accounting for Correlation 145 7.1 Multivariate Regression & LS Estimators 146 7.2 Reduced Rank Regressions (RRR) 148 7.3 Regularized Estimation of B 150 7.4 Joint Regularization of (B;) 152 7.5 Implementing MRCE: Data Examples 155 7.5.1 Intraday Electricity Prices 155 7.5.2 Predicting Asset Returns 158 7.6 Further Reading 161
Synopsis
Methods for estimating sparse and large covariance matrices Covariance and correlation matrices play fundamental roles in every aspect of the analysis of multivariate data collected from a variety of fields including business and economics, health care, engineering, and environmental and physical sciences. High-Dimensional Covariance Estimation provides accessible and comprehensive coverage of the classical and modern approaches for estimating covariance matrices as well as their applications to the rapidly developing areas lying at the intersection of statistics and machine learning. Recently, the classical sample covariance methodologies have been modified and improved upon to meet the needs of statisticians and researchers dealing with large correlated datasets. High-Dimensional Covariance Estimation focuses on the methodologies based on shrinkage, thresholding, and penalized likelihood with applications to Gaussian graphical models, prediction, and mean-variance portfolio management. The book relies heavily on regression-based ideas and interpretations to connect and unify many existing methods and algorithms for the task. High-Dimensional Covariance Estimation features chapters on: Data, Sparsity, and Regularization Regularizing the Eigenstructure Banding, Tapering, and Thresholding Covariance Matrices Sparse Gaussian Graphical Models Multivariate Regression The book is an ideal resource for researchers in statistics, mathematics, business and economics, computer sciences, and engineering, as well as a useful text or supplement for graduate-level courses in multivariate analysis, covariance estimation, statistical learning, and high-dimensional data analysis., Methods for estimating sparse and large covariance matrices Covariance and correlation matrices play fundamental roles in every aspect of the analysis of multivariate data collected from a variety of fields including business and economics, health care, engineering, and environmental and physical sciences.
LC Classification Number
QA279.P68 2013
Description de l'objet du vendeur
Évaluations comme vendeur (475 654)
- p***p (47)- Évaluation laissée par l'acheteur.Six derniers moisAchat vérifiéGreat seller! Item is what I ordered; good communication; shipped promptly; good value. NOTE TO SELLER: packaging was NOT appropriate for item; it was a flimsy, plastic envelope, with no stiff material to prevent creases. The book came with two deep creases that involved the *entire* item: one is a 1" triangle lower left side (bound edge); the other is a 7" triangle on upper right side (open edge).Beautiful Music for Two String Instruments, Bk 3: 2 Violins by Samuel Applebaum (#403989405345)
- s***s (129)- Évaluation laissée par l'acheteur.Six derniers moisAchat vérifiéThis is an outstanding seller to deal with. Fair prices that are more than reasonable in this economy. The product is in better condition than described, a true value for my money. Packaged and shipped well shows seller has concern for the products he sells to arrive in excellent condition. The seller is friendly and communicates timely with his customers. I highly recommend this seller and would do business again anytime. Thank you!
- o***o (80)- Évaluation laissée par l'acheteur.Six derniers moisAchat vérifiéThe seller charged a very reasonable price and shipped it quickly in a sturdy package. It arrived fast and in perfect condition. There was no need for further communication. They did a great job and I strongly recommend this seller.