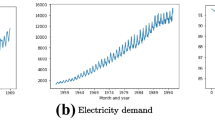
Overview
- Presents methods and applications of time series analysis and forecasting using Python
- Addresses common statistical methods as well as modern machine learning procedures
- Provides a step-by-step demonstration of the Python code, and exercises for each chapter
Part of the book series: Statistics and Computing (SCO)
Access this book
Tax calculation will be finalised at checkout
Other ways to access
About this book
Similar content being viewed by others
Keywords
- Time Series Analysis
- Python
- Forecasting
- Big Data Analysis
- Data Visualization
- Machine Learning for Time Series
- Artificial Intelligence
- Stationary Time Series
- Nonstationary Time Series
- Multivariate Time Series
- Financial Time Series
- State Space Models
- Markov Switching Models
- ARMA and ARIMA
- Data Science
Table of contents (10 chapters)
Authors and Affiliations
About the authors
Alla Petukhina is a Lecturer at the School of Computing, Communication and Business, HTW Berlin, Germany. She was a postdoctoral researcher at the School of Business and Economics at the Humboldt-Universität zu Berlin, where she obtained her PhD in Statistics in 2018. Her research interests include asset allocation strategies, regression shrinkage techniques, quantiles and expectiles, history of statistics and investment strategies with crypto-currencies.
Bibliographic Information
Book Title: Applied Time Series Analysis and Forecasting with Python
Authors: Changquan Huang, Alla Petukhina
Series Title: Statistics and Computing
DOI: https://doi.org/10.1007/978-3-031-13584-2
Publisher: Springer Cham
eBook Packages: Mathematics and Statistics, Mathematics and Statistics (R0)
Copyright Information: The Editor(s) (if applicable) and The Author(s), under exclusive licence to Springer Nature Switzerland AG 2022
Hardcover ISBN: 978-3-031-13583-5Published: 20 October 2022
Softcover ISBN: 978-3-031-13586-6Published: 20 October 2023
eBook ISBN: 978-3-031-13584-2Published: 19 October 2022
Series ISSN: 1431-8784
Series E-ISSN: 2197-1706
Edition Number: 1
Number of Pages: X, 372
Number of Illustrations: 3 b/w illustrations, 246 illustrations in colour
Topics: Statistical Theory and Methods, Statistics and Computing/Statistics Programs, Econometrics, Python, Machine Learning, Statistics for Business, Management, Economics, Finance, Insurance