Abstract
Recovery procedures are targeted at correcting issues encountered by robots. What are people’s opinions of a robot during these recovery procedures? During an experiment that examined how a mobile robot moved, the robot would unexpectedly pause or rotate itself to recover from a navigation problem. The serendipity of the recovery procedure and people’s understanding of it became a case study to examine how future study designs could consider breakdowns better and look at suggestions for better robot behaviors in such situations. We present the original experiment with the recovery procedure. We then examine the responses from the participants in this experiment qualitatively to see how they interpreted the breakdown situation when it occurred. Responses could be grouped into themes of sentience, competence, and the robot’s forms. The themes indicate that the robot’s movement communicated different information to different participants. This leads us to introduce the concept of movement acts to help examine the explicit and implicit parts of communication in movement. Given that we developed the concept looking at an unexpected breakdown, we suggest that researchers should plan for the possibility of breakdowns in experiments and examine and report people’s experience around a robot breakdown to further explore unintended robot communication.
1 Introduction
Robots are developed to do specific tasks, and people interacting with them expect them to perform these tasks correctly and efficiently. In a dynamic and unpredictable environment, however, robots are vulnerable to unforeseen issues. If, for instance, a robot suddenly becomes unaware of where it is, it will have to reorient itself. Even in controlled environments, robots can still function incorrectly, and people seeing the robot will inevitably interpret its malfunction.
In an earlier experiment we ran, participants collaborated with a mobile robot to tidy up in a home environment [1]. The goal of the experiment was to see if the way robot sped up and slowed down changed people’s opinion about the robot. During the experiment, an unplanned event sometimes occurred where the robot would become “stuck” in the navigation stack. This made the robot pause or go into a recovery procedure to free itself. The experiment did not lead to an interesting quantitative result, but participants remarked about the recovery procedure when answering questions during the experiment. So, we used the serendipity of the situation to examine if statements from the participants could provide insights into future study design or help to develop new recovery procedures.
In this article, we present a case study to systematically evaluate unanticipated breakdown situations that occurred in the original experiment. We analyze the participants’ qualitative responses on how well the robot handled the task. We identify three themes in the responses after the robot paused or ran its recovery procedure. The themes show that the robot’s movement communicated different things to the participants. We introduce the concept of movement acts to examine different aspects of a movement’s implicit and explicit communication to better communicate with human participants. The participants’ statements show that examining unplanned breakdown situations can yield interesting data that might otherwise be ignored.
In particular, insights from our analysis help to better understand the nature of a robot’s social signals and they are thus valuable for application in real-world scenarios. People need to trust robots to work with them or accept their services, and a mismatch between the expectation and reality can lead to a loss of trust [2]. Furthermore, even single violations can lead to a significant reduction of trust in the technology [3]. It is therefore important to design robots to compensate for possible negative feelings or concerns. By examining people’s opinions in a human–robot interaction (HRI) scenario where the robot does not work as expected, we may get a better understanding of people’s feelings toward robots in other breakdown situations as well. Thus, the study might help to identify factors that could affect trust in encounters where the robot faces an issue but also in those with a flawless robot performance. Finally, there is a benefit from examining breakdowns in an experiment. The examination may produce interesting quantitative results to inform future study design and supplement already suggested best practices [4].
We begin by presenting how a robot’s movement can carry meaning explicitly and implicitly (Section 2). We then review other studies that have examined breakdown situations in HRI (Section 3). Then, the original experiment design is presented (Section 4), which is the setting for the case study. Next, the case study is presented with an elaboration on the unplanned recovery procedure, a description of our analytical procedure, and the presentation of results that include common themes we identify from participants’ opinions (Section 5). We discuss the communicative nature of each themes, introducing and discussing the term movement acts (Section 6). We provide suggestions for incorporating unexpected movement acts in study designs and limitations of our analysis before concluding (Section 7).
2 Social signals and cues
A central challenge in social robotics is to understand how humans interpret the meaning of a robot’s actions and behaviors [5]. Since information between humans and robots is typically exchanged through seeing, hearing, and touch [6], a person can interpret a robot’s capabilities and intentions through non-verbal communication such as gestures, facial expressions, or movement in space. These have been called communication modalities [7]. Each modality can be thought of as having an explicit and an implicit dimension, where the latter often has an unintended component [8].
Before presenting the case study, let us establish some background on how movement can implicitly communicate social cues alongside its explicit meaning. We start first with examining how people can find meaning in movement itself. Then, we will review how robot behavior, in particular, is interpreted socially by humans. This will help to explain why it is interesting to consider a robot’s movement in a breakdown situation.
2.1 Communication through movements
Speech act theory posits that humans are attuned to a speaker’s intended meaning (i.e., the content of the words and sentences themselves) and to the speaker’s utterances (i.e., the acts of speaking or not speaking). The utterance itself can contain “requests, warnings, invitations, promises, apologies, predictions, and the like” [9, p. 1]. The theory draws parallels to Watzlawick et al.’s [10] first axiom of communication that states “[...]no matter how one might try, one cannot not communicate. Activity or inactivity, words or silence all have message value” [10, p. 30]. That is, it is impossible to not communicate and there is no such thing as a non-behavior. Expanding this to include movement, humans, as social beings, are sensitive to both the implicit and explicit dimensions of movement as well. They actively look for and interpret signals of social behavior.
While all explicit communication signals transport information with a defined and intended meaning from the sender to the receiver on purpose, implicit communication requires interpretation of the information on the receiver’s end [11]. This implicit communication can be misinterpreted as other information is often inadvertently conveyed that may or may not be incidental. This information could include the sender’s emotional state, inner motivation, or intention behind an utterance or an action [7], and can be interpreted by the receiver consciously and unconsciously. That is, information can be sent and received without an intended message, and the unintended message can lead to misunderstandings. For example, some movements are intended to explicitly signal a message, like waving to a friend. Upon receiving such a signal, the receiver might interpret the intended message, while at the same time be sensitive to all layers of social information implicit in the act of waving and the context in which it occurs [12]. Yet, many movements and behaviors are often merely incidental. For example, a friend moving and extending arms trying to find the proper angle for stretching gets incorrectly interpreted by you that your friend is waving hello to you.
Moreover, movement itself can generate meaning for humans even if it is not exhibited by a living being. It is now generally recognized that most people will assume intentions of objects and figures that move in a certain way, even though they are aware that the objects and figures are not actually alive. The phenomenon, usually referred to as anthropomorphizing, was demonstrated in a study where humans observed the movements of geometrical shapes and the observers assigned the shapes agency, motive, and personality [13]. Recently, the phenomenon was categorized as a type of experienced sociality [14]. A related but slightly different kind of experienced sociality is sociomorphing. It occurs when a person interacts with a non-human agent and attributes to the agent social capabilities although it might not necessarily have human-like properties [14].
How do we examine these phenomena? One solution is to use semiotics, the study of signs and their usage. The most common understanding of signs is a dyadic relationship between the signifier and the signified: A sign represents its object in some respect. Semiotics is often associated with text and media analysis, but signs do not necessarily need to be linguistic symbols. Furthermore, the study of signs is not exclusively looking for symbolism and hidden meaning in the different forms of storytelling in text and media. In Pierce’s pragmatic tradition of semiotics [15], a sign is not a dyadic relationship; instead a sign is a triadic relationship between the signifier, the object signified, and an interpreter (or “translator”) of what is represented. The study of signs in the pragmatic tradition of semiotics is thus concerned with the study of how meaning is generated in this triadic relation. So, according to the pragmatic tradition of semiotics, communication can be unconscious and pre-reflexive, and forms of unconscious communication and sign processing exist beyond human language [16]. Thus, anthropomorphizing and sociomorphing are two examples of pre-linguistic meaning-making phenomenon occurring in everyday experiences.
Because of the asymmetrical social capabilities of humans and robots [17], the first axiom of communication might not translate perfectly to HRI; robots move and behave in human social spaces, but cannot truly be considered to have feelings, moods, purpose, etc. The semiotic perspective, however, is sensitive to any layer of meaning implicit within any and every movement and enables us to analyze robot movements and non-movements, for example in a breakdown situation, as meaningful, even if no message was intended to be communicated to a user.
2.2 Interpreting robot behaviors socially
A robot’s core functionality is often enhanced using social features to make the interaction more robust [18]. That is, by using shapes that can be socially interpreted or by actively communicating the robot’s current state, it is easier for people to interpret the robot’s function and behavior [19]. Knepper et al. [20] argued that actions performed in collaboration between humans and robots will be interpreted as functional and communicative. As with other humans and inanimate objects, humans interpret a robot’s signals and cues even when these signals and cues might not have an intended or well-designed social meaning. That is, the robot’s blinking lights, noises from motors, or body movements sometimes have an unintended effect on a robot’s social perception [8]. For example, even though robots may deliberately make sounds intended to communicate with people (intentional sounds), the noise produced by actuation servos for robot functionality (consequential sounds) also shaped people’s interaction with the robot [21]. Because consequential movements and noises are inevitable to get the robot to move, designers and developers were encouraged to consider what might be implicitly communicated to the user through these modalities, especially considering that robots do not need to be anthropomorphic to be sociable [22]. For example, the Fetch robot (Figure 1) uses its pan-tilt camera in its head to support its navigation algorithm. In our experiment (Section 4), the robot’s movements of this part could be misinterpreted as head movements bearing social gaze.
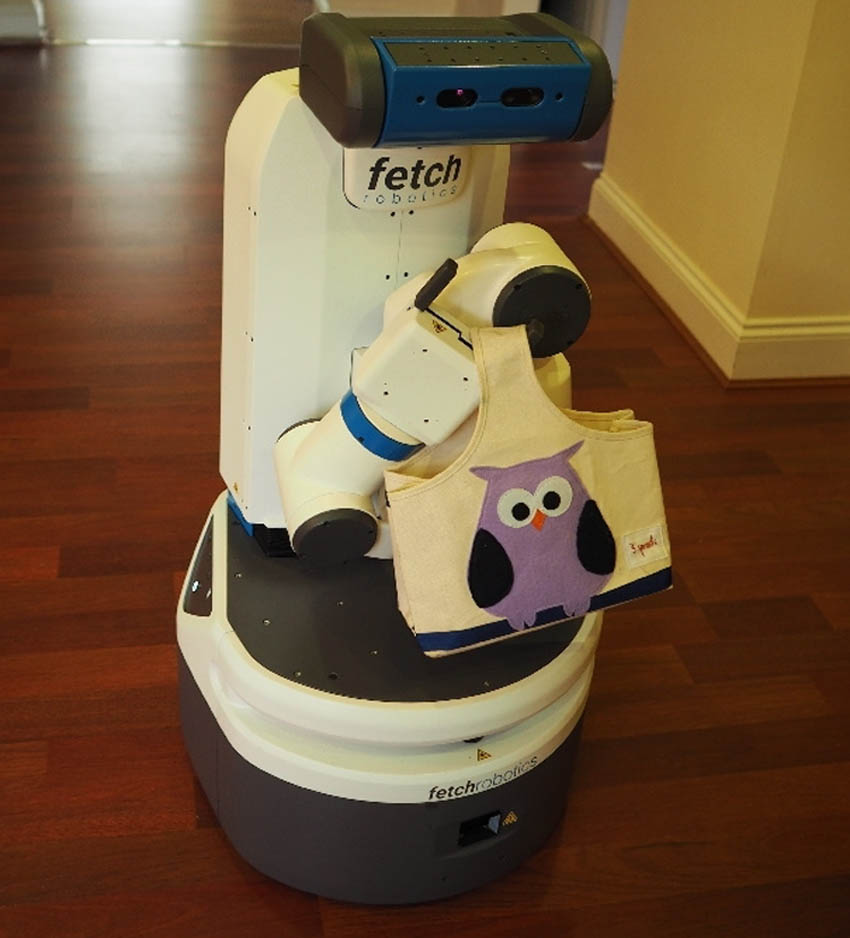
The Fetch robot at Robot House, its arm configuration, and the basket used for the experiment.
Modeling and exhibiting social signals appropriately can aid the robot in communicating its current state [19] and guide users through an interaction situation [23]. Several studies have found that the intentional use of explicit and implicit cues, such as verbal, vocal, gaze, gestures, and proximity, can influence people’s opinion of the robot [24]. Some examples of embodied cues influencing people’s opinion include using motion that communicate the robot’s collision avoidance strategy instead of its destination [25] or expressing the internal state of the robot by timing the robot’s movements [26]. Techniques from animation, such as the 12 animation principles [27], have also been used to communicate a robot’s intent to people watching or working with a robot [28].
Purely movement-based interactions can also be successfully implemented. The creators of a mechanical ottoman made it move in such a way as if to ask if the person in the room was willing or available to interact with it [22]. The study illustrated that the designers were aware that there is an explicit and an implicit dimension to the ottoman’s movement. Another study had a robot move its arm using what the researchers characterized as legible motion. The human collaborator could better infer the robot’s goal and resulted in better collaboration on a shared task [29]. Cooperation between humans and robots also improves when developers carefully consider how to use a robot’s movement for expressing its purpose, intent, state, mood, personality, attention, etc. [30].
There are also examples of what can happen when robot motion does not take into account how a robot may appear socially, even when it is not regarded as a social robot. A mismatch between the expectation and reality may, for example, lead to a loss of trust [2]. In one instance, a military robot was deactivated after it made unanticipated movements, and people distrusted it [31]. Another example is in a study where people showed tendencies toward anxiety and discomfort when they were uncertain how a robot arm would move as they worked together in proximity on a task [32]. In one study, people viewed a robot in virtual reality and on video sorting balls according to color. Participants watching the video trusted the robot when it moved fluidly, but less when it trembled doing its task. This finding was not confirmed when the robot and person cooperated on the same task [33]. This suggests a robot’s motion may be more noticeable when the person is only watching the robot instead of working directly with the robot.
In summary, all robots’ actions explicitly and implicitly communicate information even when their actions are not intended to communicate anything. Thus, it might be interesting to examine an unexpected breakdown in an experiment and investigate how people interpret and understand the robot’s unintended communication in such a situation. In our study, we apply a semiotic perspective to the perception and interpretation of robot motion as we have a special interest in the sociability of robots. We are interested in examining how a person might see the movement of a robot as a sign of “something.”
3 Studies examining breakdown situations with robots
There are several related studies that address breakdown situations in HRI. In contrast to this article, all these studies examined breakdowns that happened as a part of the study design. Accordingly, their participants may not have known about the breakdown beforehand, but the people running the study did.
In this section, we first detail how these studies identify negative effects of breakdown situations on people’s opinion of a robot, such as a loss of trust, in a controlled way. This provides a starting point to analyze the observations from our original experiment and see if it can confirm previous studies’ findings or introduce new lines of thought. This section also presents studies that develop mitigation strategies to repair negative effects of breakdowns and salvage the interaction as a basis for our later discussion and identification of themes. Finally, we look at a study where the systematic documentation of accidental breakdowns in pre-tests can help to prevent them later to get an inspiration how other researchers have learned from them.
3.1 Effects on user perception
A number of studies investigate how a planned breakdown alters people’s perception of a robot and hence how such situations influence the robot’s acceptability and usefulness. In general, it appears that different contexts lead to different implications for a robot’s breakdown or errant behavior. For example, in one study where children were to engage with a robot, a robot that displayed unexpected behavior elicited more engagement from the children than one that behaved as expected [34]. In a different study, a human and robot worked together on memory and sequence completion tasks. When the robot made mistakes, it triggered a positive attitude for the human, but lowered human performance [35]. Yet another study found that participants preferred a robot that made mistakes in social norms and made small technical errors in an interview and instruction-giving process than one that performed flawlessly, but the study found no differences in the robot’s perceived intelligence or anthropomorphism [36]. In contrast, we suspect that participants in our original experiment might have been frustrated or irritated by the breakdown instead.
Some studies have examined specifically how breakdowns affect human trust in robots. A meta-analysis of factors influencing trust in HRI found that the robot’s task performance had a large impact on people’s trust [37]. In another study, researchers looked at how willing people were to follow odd commands, such as watering a plant with orange juice, from a robot that was acting faulty [38]. Although the robot’s behavior affected participants’ opinion of the robot’s trustworthiness and the participants had different opinions about the odd requests, many of the participants honored the requests. The researchers speculated that this could be due to some participants feeling they were in an experiment and actions therefore had low stakes. Similarly, we are interested in examining how erroneous behavior that cannot easily be interpreted might have affected the users and their perception of the robot.
Another study provided different ways that a robot could handle a breakdown while playing a cooperative game with someone and looked at people’s trust in the robot afterward [39]. For some participants, the robot would freeze while speaking in mid-sentence during the game. It would then either start from the beginning or pick up from where it left off. It could then provide a justification for why it froze or offer no explanation. The robot’s freeze had a negative effect on the participants’ perceived trust of the robot, but restarting the interaction had a more negative impact on the perceived trust than if the robot continued. Robots that continued and provided a justification for freezing further reduced the negative perceived impact of trust. Similarly, in our study, we are interested in examining if a robot’s freezing and recovery behaviors might have caused negative effects on the users’ perception.
3.2 Repair and mitigation strategies
Some studies have evaluated different mitigation techniques to salvage an interaction despite the occurrence of breakdowns. One experiment investigated whether some robot action can repair the situation after a planned breakdown [40]. In the experiment, participants observed a scenario between a robot and person. The observers then rated the robot and the service it provided. The robot’s breakdown had a negative influence on how observers rated their satisfaction with a robot and the service, but different mitigation techniques (no mitigation, apologizing, or offering compensation) could change the observer’s opinion of the robot’s service or the interaction. There was also a correlation between an observer’s orientation to service (more relational versus more utilitarian) and how well the mitigation performed. As long as a mitigation was provided, observers rated the robots as more human-like regardless of the robot’s form.
A different approach is the strategy of calibrated trust where the person’s expectations are tuned to the robot’s shortcomings or potential malfunctions [2]. For example, participants in the study above by Lee et al. rated the task more difficult for the robot if the robot warned early that it might not complete the task correctly [40]. This article examines both aspects, i.e., it provides help with planning and adjusting the robot’s behaviors to the participants’ expectations, and it provides tools to design fallback strategies for unexpected cases.
3.3 Unexpected breakdowns
Most breakdowns do not happen according to plan and study data from these breakdowns are often discarded to make data analysis easier. A few researchers have argued that there is value hidden in data discarded due to robot breakdowns and other error situations [41]. For example, Barakova et al. have documented a robot’s unexpected behavior in pilot studies with children with autism and how the unexpected behavior affected the children [42]. The unexpected errors were the result of a mistake by the leader of the session, software problems, or issues with the robot. The experiences lead the researchers to document their redesign of the study and changes to the robot’s software to eliminate the issues for the final study [42]. The documented changes are useful for other researchers designing similar studies.
Our purpose here was to look at people’s opinion of the robot’s breakdown situation in an experiment that was not designed for a breakdown and where breakdowns were not present in the pilot study. We wanted to examine the participant comments and see what lessons we could learn for future experiments and robot design. We performed this examination through the lens of explicit and implicit communication.
4 Case setting: earlier experiment
The case study focuses on people’s opinion of a robot during a temporary breakdown and self-recovery of its navigation system. The data are collected from an earlier experiment that examined how people reacted to a robot that moved using two different velocity curves [1]. To introduce the case, we document the robot and setting that was used in the earlier experiment, its procedure, and the data that were collected. Although the experiment’s method was documented previously [1], we present an expanded description of the experiment here to highlight some constraints and challenges in the design.
The original intention of the experiment was to look at one animation principle, slow in and slow out, and see how it affected people’s perception of the robot. The slow in and slow out animation principle states that the speed an object moves at changes through its journey: motion is slower at the beginning and at the end [27]. Using the slow in and slow out principle should lead to a motion that appears more “natural” and less “robot-like.”
Given the constraints of designing and running the experiment, we went for a within-subject design for the experiment. This decision likely had an effect on the quantitative results (e.g., there could be a learning effect between studies [4]), it is less important for the purposes of a case study, especially given that the breakdown was unplanned and occurred throughout the whole experiment.
As we designed the experiment, we were concerned that if we simply presented the robot moving using a velocity profile using the slow in and slow out animation principle or the standard linear velocity profile and asked people their opinion, they would manufacture a response to satisfy our question, and we would not get their actual perception. We decided that participants would take part in a task that was dependent on them watching the robot’s movement and seeing the movement from different angles, but participants were not explicitly asked about the robot’s movement. The participants’ answers would focus on the way the robot performed the task and not on how the robot moved. The experiment would see if the way that the robot moved affected the participants’ opinion of the robot.
4.1 Experiment setting, questionnaire, robot, and navigation system
The procedure was approved by the University of Hertfordshire Health, Science, Engineering and Technology Ethics Committee (Protocol Number COM/SF/UH/03491) and took place at the University of Hertfordshire’s Robot House. Robot House is a place that people can visit and experience robots and sensors in a home environment instead of a typical lab environment. Since the overarching goal of the research is to have a robot be a part of a home and that the robot’s movement should appear more friendly and ultimately lead to better trust in the robot, it seemed appropriate to run the experiment in a physical area that resembled a home environment rather than a lab.
The questionnaire for the original study included the Godspeed series [43]. We also included an additional Likert item about how well the person could predict where the robot would go, and an open question: “What do you think about how the robot handled this task?” We included the prediction item as we wondered if the different velocity profiles would affect how easy the person could predict the robot’s movement. The results from the Godspeed series were reported previously [1]. The open question gathered qualitative information and is the basis of our analysis below (Section 5).
The robot we used was a Fetch Mobile Manipulator from Fetch Robotics [44] hereafter referred to as Fetch (Figure 1). We selected Fetch since it can move at a rate of 1 m/s. This speed is slower than an average person’s walking speed of 1.4 m/s [45], but accelerating up to this speed takes enough time that it is possible to create different velocity profiles.
The linear and slow in and slow out velocity profiles were based on the algorithm described in Schulz et al. [46] and adapted to a plugin for the local navigation planner in Fetch’s navigation stack, which is the navigation stack from the Robot Operating System (ROS) [47]. Fetch’s local planner uses the trajectory roll out scheme [48]. This method of integration is similar to a set up suggested by Gielniak et al. [49] for integrating stylized motion into a velocity profile for a task. The plugin included dynamic parameters for setting the velocity profile (linear or slow in and slow out). This allowed us to change the velocity profile without restarting the robot’s navigation system. The changes only affected Fetch’s linear velocity (i.e., moving forward); the angular velocity (i.e., turning in place) was unchanged from the original plugin and thus always used a linear velocity profile.
We considered ignoring the environment and simply issuing pre-recorded velocity commands to Fetch. This technique would have resulted in smoother velocity curves, but we were concerned that small inaccuracies would occur while turning, starting, and stopping would lead to large inaccuracies as Fetch moved through the house. Fetch’s navigation stack had already been extensively tested for moving the robot around and avoiding obstacles. After investigating both, we found that Fetch’s navigation stack with our developed plugin worked better than any solution we could develop from scratch in the time given for the experiment.
Fetch moved between several preassigned destinations in the house: (Positions 1, 2, and 3, respectively, on Figure 2). Each spot had two poses, one for facing the person and one for facing away from the person toward the next location. The two poses per location were done to keep the performance of Fetch’s navigation similar across conditions. Position 1 had a slightly different location for its poses to make it easier to remove and add items to the basket without the participant noticing.

Floor plan and position of people for the experiment. The robot would move between the numbered positions, starting at Position 1, using either a linear or slow in and slow out velocity profile.
4.2 Experimental procedure and data collection
Participants that had consented to being part of the experiment entered Robot House and filled out demographic information of age, gender, and if the participant had any experience with robots.
After the participant filled out this questionnaire, we went through safety information with the participant for interacting with the robot. We explained that they would be interacting with a Fetch robot during the experiment and that two of us would be constantly monitoring the robot. Fetch was brought over and controlled with the remote control during this explanation. We told the participants that we did not expect any safety issues, but advised them not to approach the robot while it was moving and that if the emergency stop was engaged, that the robot would keep its momentum and move unexpectedly. Participants were told they could end the experiment at any time if they felt unsafe (none of the participants ended their participation). As the safety information was being explained, one of the facilitators remotely controlled the robot and moved it towards the participant so the participant could see how the robot moved and see its size. Participants could ask additional questions regarding safety at this time.
Then, the scenario was explained. The participant was visiting a friend’s house to help in cleaning up the home (the facilitator that had controlled the robot was introduced as the friend). Cups had been placed on the dining table and the coffee table near some couches. These cups needed to be returned to the kitchen. The robot would aid in the cleanup by collecting cups from the participant and taking them to the kitchen. Since we did not want to draw attention to the robot’s motion, we explained we were interested in how the robot handles the hand over of objects from the participant. We instructed the participant where to stand, what to do, and what the robot would be doing (Figure 2).
The facilitators and the participant would then take their positions. Video recording of the procedure from one camera was started for participants that consented. One facilitator would stand in the kitchen (near Position 1 in Figure 2); the participant and the facilitator helping in cleaning the house would stand near the dining table and sit on the couch, respectively (near Positions 2 and 3, respectively, in Figure 2). Fetch would be sent to Position 1 in Figure 2. The remote control was placed on the table near the sofa to indicate the robot was not being teleoperated, but the remote control was in easy reach of the facilitator if something were to go wrong.
Starting at Position 1, the procedure was the following: (1) The robot moved from Position 1 to Position 2. (2) The participant took one of the cups from the dining table and put it in the robot’s basket (Figure 1). (3) The robot moved from Position 2 to Position 3. (4) The facilitator on the couch took a cup from the coffee table and put it in the robot’s basket. (5) The robot moved to Position 1. (6) The facilitator in the kitchen removed the cups and put a copy of the questionnaire in the basket. (7) The robot moved to Position 2. (8) The participant took the questionnaire from the robot and filled it out. (9) Once the questionnaire was complete, the participant put the questionnaire back in the robot’s basket. (10) The robot moved to Position 1. (11) Finally, the facilitator in the kitchen removed the questionnaire and prepared the robot for the next iteration.
This procedure was performed for four iterations: two times the movement was with a linear velocity profile, and two times the movement was with a slow in and slow out velocity profile. The profiles were counterbalanced to avoid ordering effects. The counterbalancing was achieved by taking the six possible combinations of two linear and two slow in and slow out velocity profiles, and randomly selecting an ordering for each participant.
After the final iteration, any video recording was stopped and participants went through an ending procedure. They filled out a questionnaire with open-ended questions concerning the overall interactions: “Do you have any general impressions about the robot during your interaction with it?” and “Do you have any questions you would like to ask us?”
We also informed participants that we were actually interested in the robot’s movement and not the handover. We used this opportunity to answer their questions and go into more technical details about how the robot sensed its environment and moved around the house. Participants were encouraged to ask any additional questions about the set up, the robot, and the experiment. Finally, we thanked participants for their time and, as noted in the informed consent form, gave them a
Since we were concerned how the robot’s movement affected people’s perception of the robot, we removed some confounding factors to improve the internal validity of the experiment. For example, we chose to use a basket for collecting cups and the questionnaire since Fetch’s arm movement is not deterministic and would confuse participants. In addition, Fetch only moved and did not use speech recognition or sound. A pilot study revealed that it was confusing for the person to know when it was OK to put a cup in the basket. To signal to the person that Fetch was ready to receive a cup or take and return a questionnaire, it would raise its torso 10 cm when it had arrived at the pose facing that person.
Participants were asked to stand if able while giving the cup to the robot and receiving the questionnaire (all participants were able to stand). They could sit while filling out the questionnaire. The primary reason was to allow a better view of Fetch and keep the base for participants’ perceptions similar since a standing participant is taller than the robot, which might not be true with a sitting participant. A lesser, secondary reason was to make participants feel safer as the robot approached since we reasoned that participants may feel easier to move away from a robot when they are already standing versus having to get up from a chair. For an additional level of safety, having a person in the kitchen and the couch also allowed two people to watch the robot and activate an emergency stop if Fetch was going to run into something.
Fetch was partially controlled via Wizard of Oz. In line with recommendations from Riek [50], we include the additional information about our use of Wizard of Oz. The wizard, the facilitator in the kitchen and this paper’s first author, acted as the robot’s eyes and as a conductor for the robot. The wizard was in charge of noticing when the participant or the facilitator had put the cup into the basket. Then, the wizard would signal for the robot to go to the next pose. The robot would then navigate to the next position using its navigation stack. We chose to use a Wizard of Oz component to reduce variability of time for the experiment with the robot detecting the cup or questionnaire was added to the basket. Given that Fetch traveled a fixed route and the participant’s role was rigidly defined, the Wizard of Oz component could have been eliminated given enough time. The wizard also noted down observations for each iteration.
For additional data, we collected the robot’s odometry information and the time from when a request to move was made to move to the next location until the time that the robot arrived at the location and raised its torso.
5 The case study
In this section, we document the unexpected robot’s recovery procedure and describe how we analyzed the data collected from the previous experiment for the case study in this article.
5.1 Robot’s recovery procedure
From the Godspeed questionnaire, the participants’ responses were not different enough between the linear or slow in and slow out velocity curve [1]. When we were examining the qualitative, free-text comments, we noticed how some comments expressed feelings of discomfort, curiosity, or confusion from interacting with Fetch, especially during its recovery procedure. The unplanned phenomenon became part of the interaction in a substantial amount of the trials, happening 68 times in total or around 9% of the time when the robot moved. A total of 31 of the 38 participants experienced at least one of these issues.
The recovery procedure occurred when Fetch encountered problems in calculating its path for moving across the room. The recovery procedure caused the robot to move differently than its intended behavior and was not planned for by the facilitators. As part of the navigation stack, the recovery procedure was likely implemented to get Fetch to move again without considering what an observer would see. During the pilot and testing, the breakdown situation did not occur. Once discovered, the functionality could have been disabled, but it would have increased the chance the robot did nothing, which would have caused an even larger interruption during the experiment.
Our interest is in the case of the unexpected breakdown. Furthermore, the irregularity of its occurrence makes it unfit for quantitative analysis. Instead, we analyze the comments qualitatively and cross-reference them with our notations of when Fetch had issues, and how that issue manifested in the robot’s movement and behavior.
Normally, the robot navigated competently between the positions in Figure 2. When Fetch received an instruction to proceed to the next navigation point, its head would look up and down as it calculated the path and speed to travel. The head movement would pan-tilt the depth camera inside Fetch’s head and support its navigation algorithm. When a path had been calculated, it would straighten its head and proceed on the path.
This process would normally be completed within a second or two, and Fetch would begin to move. Sometimes, however, it encountered problems in calculating its navigation path. In these situations, it would continue trying to calculate a path and the head would continue to move up and down until one of the following things happened: (1) it succeeded in calculating the path and started on the path after the delay or (2) if the navigation software had not calculated the path after 25 s, it decided that that Fetch was “stuck.”
If Fetch was stuck, the navigation software would rotate the robot

Example of the recovery procedure when Fetch traveled from Position 3 to Position 1. In (a), Fetch is at Position 3 and has received the cup. Then, it rotates to go to Position 1 (b). Once the rotation finishes, it tries to compute the route to Position 1. If this takes longer than 25 s, Fetch is considered “stuck” and rotates
5.2 Analytical procedure
To begin our analysis, we looked at the logs of the robot’s performance and noted when it paused or when it became stuck and initiated its recovery procedure. We then arranged the participants’ responses to the open questions on each iteration and their overall opinions into tables, arranged first by trial and later by participant. This resulted in tables that charted the journey of the robot, and we could easily follow the robot, the problems it had, and the responses from the participant. The answers from all the participants were manually annotated through emergent coding, first deductively and then inductively [51]. The themes presented in the results emerged from the coding during the inductive approach, which was performed by two researchers who then met to harmonize on the themes. Exactly how many iterations and re-reading of the comments were not counted: Each researcher read through as many times as they needed to make sense of the data.
5.2.1 Fetch’s performance
The study had 38 participants: 19 identified as female and 19 identified as male. The participants’ ages were from 18 to 80 years (average age: 37.39 years, median age: 34.5 years, SD: 15.74 years). A total of 22 of the 38 (around 58%) participants had previous experience with robots. Each participant had four iterations of the cup cleaning task (two times with slow in and slow out and two times with linear) for a total of 152 encounters (76 for slow in and slow out and 76 for linear). Fetch’s journey for each iteration can be divided into separate stages or legs (e.g., in one leg, Fetch traveled from Position 3 to Position 1). Each iteration had five legs. The total number of legs over all iterations is 760.
With our focus on the breakdown, we examined the videos of participants and noted Fetch’s behavior. Fetch’s behavior was divided into four classifications: (1) no problem: the robot worked as intended, (2) delay: Fetch made a longer calculation than normal, (3) stuck: Fetch was stuck and went into recovery mode, and (4) other: an event that could not be placed in the other behaviors (those three events are described below). These classifications were checked against the observation notes from the facilitator and could also be confirmed using Fetch’s odometry logs. This also enabled us to classify for participants that did not wish to be recorded on camera (one participant chose not to be recorded). The coding for the videos was obvious and the classification was in agreement.
Table 1 shows counts for events as the robot moved from position to position split by velocity curve. The three events marked as other were as follows: (1) the software crashed after the final questionnaire was filled out, (2) a near collision with the table at the sofa when the robot traveled from Position 2 to Position 3, and (3) the robot shook as it returned from Position 2 to Position 1.
Count of occurrences the robot had problems moving from position to position split by velocity curve for linear and slow in and slow out, respectively (
|
|
|
|
|
Total | |
---|---|---|---|---|---|---|
Linear | ||||||
Stuck | 1 | 0 | 5 | 0 | 2 | 8 |
Delay | 2 | 1 | 5 | 0 | 1 | 9 |
Other | 0 | 1 | 0 | 0 | 1 | 2 |
No problem | 73 | 74 | 66 | 76 | 72 | 361 |
Slow in and slow out | ||||||
Stuck | 0 | 3 | 17 | 0 | 5 | 25 |
Delay | 1 | 3 | 10 | 1 | 2 | 17 |
Other | 0 | 0 | 0 | 0 | 1 | 1 |
No problem | 75 | 70 | 49 | 75 | 68 | 337 |
The area that had the most problems was when the robot moved from the sofa area (Position 3) back to the kitchen (Position 1). The robot was stuck 17 times during the slow in and slow out curve and 5 times for the linear velocity curve. Overall, Fetch was stuck 25 times when it used the slow in and slow out curve versus the 8 times when it used the linear curve. If we look at these numbers in terms of percentages, approximately 92% of the legs had no problems. Splitting it by the linear and slow in and slow out the percentages of legs with no problems were 95% and approximately 89%, respectively.
We were unsure about why there is a difference between the linear and slow in and slow out curves. Due to implementation reasons, the navigation stack ran on a separate computer and not directly on Fetch. Both curves, however, use the same code path and the only difference was the maximum speed the plugin allowed at the start and stop (in general, the slow in and slow out curve has slower maximum speeds at the start and stop). This should not have caused a problem in picking reasonable trajectories.
5.2.2 Analysis of comments
Using the tables that charted the journey of the robot with the responses belonging to each iteration in each trial, we qualitatively analyzed each response. During the deductive stage, the focus was on whether the responses descriptively commented on what happened, or if metaphors were used to rationalize Fetch’s behavior.
The inductive stage focused on how Fetch’s sociability presented itself to the participant. Through the process of manually coding in iterations, the themes emerged. Coding the data using a semiotic lens on meaning-making made it easier to stay focused on what a participant’s response could tell us about how they made sense of the movements of the robot (both during breakdown and also when it worked according to plan).
Based on this work, we identified the categories forming the themes of our annotation scheme. The themes were as follows: (a) sentience, participants associating abilities with Fetch and guessing its intention (51 comments); (b) form, participants commenting about Fetch’s form or body parts (23 comments); and (c) competence, how Fetch performed its tasks and participants’ confusion and uncertainty with the recovery procedure (53 comments), while a few (four participants) only reported on the movement with no underlying associations that we could identify. The themes are not mutually exclusive and some comments were coded into multiple themes. Table 2 breaks down the semiotic themes and their corresponding codes. Overall, the comments and themes showed up evenly distributed among all the iterations (Table 3).
Themes and underlying codes and number of comments regarding Fetch’s movement; bold indicates the theme and the total number of all its codes
Theme | Number of comments |
---|---|
Sentience | 51 |
Mood/emotion | 6 |
Hesitate/wait | 13 |
Checking/recognizing | 15 |
Deciding/confused | 9 |
Helping out | 2 |
Intelligent/smart | 5 |
Asking for cup | 1 |
Form | 23 |
Eyes/look | 9 |
Head/nod | 11 |
Arm/extend | 3 |
Competence | 53 |
Handle/do well | 32 |
Slow | 21 |
Breakdown of comment theme versus which iteration the comment was written, or if it was written at the end of all iterations; some comments are counted in multiple themes
Theme | It. 1 | It. 2 | It. 3 | It. 4 | End | Total |
---|---|---|---|---|---|---|
Sentience | 11 | 9 | 12 | 8 | 11 | 51 |
Form | 3 | 6 | 2 | 5 | 7 | 23 |
Competence | 12 | 11 | 11 | 13 | 6 | 53 |
5.3 Results: themes based on comments
The comments from participants when Fetch performed correctly were generally positive about how Fetch handled the task. While the responses made after an iteration where Fetch did not have any navigational issues are not excluded from our analysis. Going through the tables, however, it was clear that the more interesting comments were made when Fetch did not perform as expected.
We report participants’ comments from the themes of form, competence, and sentience around the delays and recovery procedures, and another unrelated event that came up often enough that we include it as well. Since there were more problems with the slow in and slow out profile iterations, there are more comments from those iterations. We did not find a difference in the nature of the comments and therefore do not differentiate between the velocity profiles below. To avoid repetition, we do not report on all comments from the legs that had problems, but instead report a representative amount.
5.3.1 Sentience
Comments categorized into this theme included words commonly used to describe actions of living, sentient beings. They are examples of participants anthropomorphizing or sociomorphing Fetch, trying to explain or rationalize what they expected the robot would do or what they thought the robot intended to do. A few participants speculated about its mood. For example, one participant felt that “it looks oddly happy doing what its doing” (Participant 35). Another participant commented that “he [sic] looked sad on the last go” (Participant 8). We have not, however, attempted to speculate on whether the participants were purposefully attempting to anthropomorphize or sociomorph Fetch using these words.
When Fetch performed its task without delay or getting stuck, the participants tended to comment that the robot “handled the task well.” But participants came to different conclusions about what was happening when the robot would pause. Some participants thought that the robot paused because it “[...]checked surroundings very well before moving back” (Participant 2), or that it “[...]felt like it was taking a bit more time to make [a] decision” (Participant 10). A third participant (Participant 27) felt that Fetch was quick and safe, and he could predict Fetch’s movements after the second iteration. When it paused on the third iteration, however, he commented that Fetch “seemed to scan its surroundings more before moving.” This was less predictable, but he felt that Fetch “was taking more precaution so completing [the] task safer.”
Other participants interpreted Fetch’s delay as confusion, “[The delay] evoked an impression of slight confusion” (Participant 33). When one participant witnessed the recovery procedure, he noted that the robot had “more confusion than last time” (Participant 17). When there were no issues on the next iteration, he declared that the robot had become “more confident.” One participant that experienced Fetch’s delay going from Position 2 to Position 3 and rotating before going to the kitchen felt that something may have been wrong with its sensors, “[Fetch was] more unpredictable, as if it couldn’t sense as well the environment,” (Participant 21). Another participant liked the recovery procedure. She “liked when it did a little twirl, but I thought that made it seemed confused[...]” (Participant 28). Finally, one participant had concern for Fetch when it executed the recovery procedure, “[the behavior] made me want to come over and check on him [sic]” (Participant 36).
Though not related to the recovery procedure, there was some confusion on when participants should hand over the cup in the first iteration. Several participants needed a hint on the first iteration, “[...]an indicator it would stop then raise up would have been helpful,” (Participant 35). One participant (Participant 19) comment that she “wasn’t sure” when to hand over the cup when Fetch first stopped. In the fourth iteration, there was a delay in Fetch raising its body, and she “wasn’t sure when exactly he [sic] would be finished and I could hand over the cup.” In other iterations, she felt that Fetch performed the task well and “wanted to say, ‘Thank You.’” Fetch would reach a position and then rotate to face the person. Sometimes it would overshoot its stop position and need to rotate back. This also led to some confusion: “It wasn’t really clear when I was supposed to give the cup. Then it’s moving around gave me the impression it was waiting” (Participant 6).
Fetch raised itself 10 cm for each participant. There would sometimes be a delay between arriving at a position and raising. During one delay, one participant (Participant 18) thought Fetch’s delay in raising its body was due to calculating the person’s hand height, “Maybe it took a little time for it to adjust to where my hands were.”
Participants also had ideas about Fetch should move in some situations. One participant (Participant 1) noted, “The movement could be slower near obstacles. The trajectory would be more reassuring of a minimal accident possibility.” Another participant was curious about “[...]how it would react to a change in conditions (fallen cup, user movement, etc.)” (Participant 27). Another participant shared this curiosity, and she noted that Fetch “Moves quite smoothly. Avoids obstacles (perhaps there are insufficiently many obstacles to show this)” (Participant 18). A different participant (Participant 22) felt that Fetch could have moved faster and gotten closer, “[Fetch was] too slow for me; could have come a bit nearer to me to collect the cup and the questionnaire.” Finally, one participant (Participant 32) commented that Fetch’s approach could have been better since “[...]sometimes the movement adds a fear to the user (whether it will stop or not).”
5.3.2 Form
Comments categorized into this theme specifically described characteristics associated with distinct “body parts” and actions supported by them.
Fetch’s head and arm gave participants certain expectations about how they should interact with it. Several participants expected Fetch would use its arm when getting the cup or at least extending the bag (Participant 6, Participant 7, and Participant 35). As mentioned in Section 4.2, the arm was disabled for safety and consistency.
Fetch’s head and its rising and lowering left some participants thinking that Fetch was doing more. “[Fetch] keeps lowering its eyes towards my groin. Is this normal?” asked one participant (Participant 1). A different participant commented that Fetch didn’t make eye contact (Participant 29). Another participant found it strange, “It felt odd that the laser scanner (or whatever it is) never tilted upwards to ‘look at’ me” (Participant 33). Yet another participant (Participant 22) commented, “I am not sure if the upping and downing of the head piece was assessing me or even waiting for me to react.” She also complained, “The sound when Fetch was going up and down was a bit annoying.” On the other hand, one participant “liked the way the robot bobs its head; it is quite humanlike,” (Participant 28).
One participant gave Fetch more abilities than it had. Upon first interacting with Fetch, one participant commented that Fetch was “smart to sense objects around it” (Participant 24). In the second iteration, Fetch ran the recovery procedure twice. The participant maintained that Fetch “[...]handled [the] task, but [was] slow in process, although it’s smart.” Later, she commented that Fetch could “sense the obstacles in between or around it and make its way back” and felt that Fetch was “quick to respond” in the final encounter. Generally, she felt that Fetch was “friendly and smart.”
Finally, aside from moving after receiving the cup, Fetch did not react to participants’ actions. This made some participants (Participant 7 and Participant 33) question whether the robot actually looked at them, even though they used both “eyes” and “look” to describe their thoughts.
5.3.3 Competence
Comments categorized into this theme encompassed a level of confusion or uncertainty with the participants around the recovery procedure. Although some participants observed the delays and recovery procedures and questioned the robot’s intentions, other participants did not like when this happened.
One participant (Participant 3) experienced several emotions of the course of his iterations with the robot. After the first iteration, he commented that “It was a bit unpredictable at first, but I got used to its actions.” On the second iterations, after Fetch miscalculated the path from Position 2 to Position 3 and almost hit the table by the couch and executed the recovery procedure, he expressed confusion: “I struggled to understand what the robot was doing.” The third iteration went better, but he still expressed worry: “I could predict what the robot was doing, but it felt like it was going too fast. It felt rushed when putting my cup in the basket.” The fourth encounter had a recovery procedure from Position 3 to Position 1 that did not make him feel comfortable: “I felt relaxed until I saw the robot pause after picking up the second cup. I wasn’t sure why it kept looking up and down, this made me a bit uncomfortable.” He finally summed up all iterations optimistically, but not fully convinced: “I felt overall comfortable with how the robot was helping me. The robot pausing for a long time made me feel uneasy at times.”
These concerns are also echoed by another participant’s experiences (Participant 15). By the second iteration, she commented that the “robot handled [the] task well; [I] felt more comfortable with the robot so made the experience more comfortable.” This continued with the third iteration: “Robot handled task smoother I feel than previous two tasks. Robot speed also feels like it increase, but it felt smoother.” This comfort disappeared after there were multiple recovery procedures in the fourth iteration: “Robot paused and was stationary for a while. The robot then turned around in a circle unexpectedly when picking up the second cup. This made it seem as if the robot lost control.” Overall, she commented that spending time with Fetch helped with the interaction: “After 1st interaction. Robot feels more natural and more easy going.”
Another participant expressed annoyance when Fetch was delayed or executed the recovery procedure. “Weird actual interaction triggers, slow turn on a spot” commented one participant (Participant 16). This annoyance continued in the second encounter “More annoyed at the slow turn in front of me [and] with being slightly stuck in the corners.” This led to different feelings on the third encounter “[it] lingered after being handed the cup. Made me feel weird/uneasy.” The delay at the start of the fourth iteration was also classified as strange: “weird long linger before the hand off made me nearly give him [sic] the cup too early.”
Concerns of unpredictability and confusion were raised by another participant (Participant 21). Initially, there was a delay after giving Fetch the cup: “[the] reaction after handing [over] the cup was too slow, [I] didn’t know if it recognized it.” Additional delays in the second iteration did not help: “More unpredictable, as if it couldn’t sense as well the environment.” The delays in raising and lowering Fetch’s body also caused issues “The pauses before/after asking for the cup make it seem more unnatural and unpredictable.” He summed up all the iterations as needing improvement: “the movement made it seem very artificial and unpredictable.”
6 Discussion
In this section, we discuss the three themes and what might be learned. Next, we introduce the concept of movement acts for examining a robot’s motion, followed by an application of this concept to the Fetch robot in our experiment and provide other examples where it can be applied. We end with a challenge for researchers to make lemonade out of the lemon in a breakdown situation.
6.1 Examining the themes
The codes that emerged during the analysis of the responses were categorized into three themes: sentience, form, and competence. These themes emerged from the coding process and were not mutually exclusive.
Sentience had the largest variety of codes. Yet, what the codes all have in common is the clear use of either directly or metaphorically describe what the robot did or did not do. Following this line of thinking, we also noticed that it was possible to further distinguish the comments (especially) belonging in the sentience category between those that directly anthropomorphize (or sociomorph) Fetch, and those where such anthropomorphism is implicit in the language used to describe it. For instance, in many of the comments coded within the sentience theme, participants explain how it “felt like,” or “was as if,” or “seemed to me like” the robot did something that gave it a life-like character. Even though Fetch was not intended to be sociable in the experiment and thus limited in its social function, this was a recurring pattern. Perhaps it was due to the context of cooperating being of a social nature. It is also possible that the Fetch’s movements in the room gave the participants an experienced sociality (such as sociomorphing or anthropomorphizing) in their interactions with Fetch.
Form had the fewest occurrences of codes related to it, and the codes that did emerge concerned only three “body parts.” Still, there were some interesting trends that appeared. During coding, we noticed a certain overlap between the comments that described Fetch’s form using anthropomorphic or zoomorphic terminology and comments that sociomorphed Fetch. For instance, small movements of the camera were perceived as social gazes or nods of a head. On the other hand, the comments about the arm were all about the lack of its function. Fetch not extending its arm when it stopped to receive the cup from the participant might be one reason for the lack of experienced sociability toward this particular part of the interaction. Perhaps putting Fetch’s arm in a sling would have helped indicate that its arm could not be extended in the interaction. On the other hand, this change could have other unintended effects on the robot’s sociability and might look inappropriate to the participants. The study of Heider and Simmel [13] shows that the exact visual appearance of a moving object is only marginally perceived by a human observer. Instead, the quality of the movement is the predominant characteristic. The experiment does, however, show that this is an area that could be examined further.
Competence had the smallest variety in codes, of which there were only two. Yet, these two codes occurred more often than the others. A large number of responses explaining how the participants felt that the robot was slow. We understood these comments as concerning the nature of the interaction in a collaborative action, which often gave rise to frustration at the robot being slow at completing the task, even if it felt safe to collaborate with it. Another reason for this high occurrence of codes regarding Fetch’s competence could be that the participants were answering an open question specifically asking how well they thought the robot handled the task.
While this is not included in our coding one interesting observation made going through the data was an apparent distinction in the responses of participants who appeared to use words with a sentience connotation without any apparent inner strife, and those who appeared less inclined to do this, but seemed to either not find, or not to bother finding other words to describe what is happening in the interaction. Fussell et al. [52] argued that it is easy for people to anthropomorphize robots in casual descriptions of robots because they use “ordinary” words. This can be related to the work of Seibt [53] who describes that varieties of “as if” (either explicit or implicit) in descriptions of human interactions with robots masks the social asymmetry of the interaction.
In general, it is difficult to discern whether the participants perceived Fetch as actually having the social and sentient abilities their words in their answers described, or if they were applied for a lack of a better way of expressing the experience. Many comments regarding Fetch’s competence were direct answer to how well the robot handled the task, and might not be the result of any sociomorphing or anthropomorphism. On the other hand, if a participant described that they felt Fetch was “checking the room” it would imply a kind of perceived competence in the robot, as checking could be characterized as knowing what to look for and getting an overview of the situation. Furthermore, a checking function like “looking” requires intent and purpose, which are relying on sentience. Further still, it would also be a kind of comment on how the participant perceived Fetch’s form, because “checking” then also requires having visual perception. Furthermore, a comment regarding Fetch “checking” could thus belong to all three categories, “looking” in two. Several of the comments were annotated with codes belonging to two or even all three themes. One method that could be used to examine this more thoroughly is the Linguistic Category Model [54], as is done by Fussell et al. [52] to examine linguistic anthropomorphism at different abstraction levels.
6.2 Movement acts
Knepper et al.’s [20] classification of intentional and consequential sounds can also apply to robot movement. A similar categorization for movements can enable us to better understand what is explicitly communicated in Fetch’s movements and what may be implicit communicated (both intended and unintended). During normal operation Fetch’s movements were primarily functional, even though Fetch did not have any movements that were designed purposefully for social interaction and giving social cues. Because Fetch did not communicate explicitly with language or sound in the experiment, the communication was purely expressed through Fetch’s movement across the room and what was explained via the facilitators. This was due to the original experiment examining different velocity profiles. The purpose was to see if the difference in the profiles communicated different information to the participants.
For example, Fetch’s journey in each iteration was functional and intentional to collect cups and return it to the kitchen, but Fetch’s rotations were functional and consequential as the movement “calculated a path” and “performed a recovery procedure,” respectively, without communicating any intended message. Still, it does not cover how meaning arises in a semiotic, triadic relationship between signifier, signified, and interpreter. That consequential movement or non-movement is present in the world for all present to observe, which can result in unintended interpretations of what that movement or non-movement meant [12].
Before the experiment, the participants were explicitly told that Fetch would be collecting cups. They were therefore aware that Fetch moved to collect cups and knew that this would be the purpose of the robot’s approaching and stopping (having the implicit meaning of “now’s the time to give the cup”). This means that even if the participants knew what the purpose was, when and how they should hand over the cup became unclear to many participants because they were expecting a social cue and hence still waited for the robot.
That the intention behind the implementation of the rotation has no explicit communication purpose, however, does not invalidate the experiences of people who interpret robot movement with a different meaning than intended – even if they are not quite sure what to make of it. Our case study has further confirmed that functional or consequential movements still communicate “something.” But as the “message” being interpreted was not intentionally sent, what this “something” ends up meaning to an observer can be difficult to predict. As this case study has demonstrated, a robot’s movement in the breakdown situation leads to different, possibly incompatible, interpretations by the participants.
Another Fetch’s consequential movement or rather non-movement was its occasional delays, where it paused longer than usual before leaving a station. These pauses also brought forth puzzled comments from the participants about what the purpose of the delay was. During our analysis, we clearly saw that these pauses, even when the participants were not quite sure what to make of it, did not go by unnoticed.
Currently, there is no framework or concept that covers the triadic relationship of different meanings that might arise during interaction with robots and that acknowledges both movement and non-movement as social signs. Therefore, we draw inspiration from the concept of speech act [55] and introduce the concept of movement act. A movement act entails the understanding that both intentional and expressive movements and intentional and consequential movements might be interpreted by an observer as communication of inner state and intention. Just as not speaking is itself an act open to interpretation by the surroundings and its inhabitants, so will also not moving be an act (as a conscious or less conscious choice) open to interpretation. For example, a pause may only be a pause, but it may also imply a sense of insecurity or confusion.
That the situation is interpreted differently based on the robot’s movements is in line with what others have already suggested: A robot, despite its limited social capabilities, is capable of communicating implicitly and explicitly using movements only. Using the concept of movement acts, we can isolate, identify, and characterize this phenomenon. We can then take each movement act and individually examine its implicit and explicit dimension. Movement acts can make sense of what a robot’s movement communicates explicitly (or lacks to communicate). Being aware of the implicit dimension allows one to systematically look for interpretations that might happen during an interaction. The notion of movement acts facilitates a behavior design process that aims for an effective and clear communication between a robot and the people who interact with it.
6.3 Applying movement acts to robots
If we apply the movement act concept to the original experiment, it can help explain some issues or provide suggestions for a better movement design.
First, although a robot’s movement can communicate information, the original experiment did not find any significant difference in the perception of the slow in and slow out and regular velocity curves. So, was the slow in and slow out motion worth the effort? The previous article [1] outlined multiple reasons why that might have been the case. Yet given the participant’s comments in the case study, it would appear that the robot’s motion in a breakdown situation drew attention away from any other type of motion. That is, the movement acts in the rotation and delay captured more attention than the movement act in the velocity profile. Although the slow in and slow out movement act was meant to be implicit in its communication, it could have been too subtle. Perhaps a slow in and slow out velocity profile cannot be used alone and may need to be used in concert with one or more animation principles – for example, exaggeration or anticipation – to capture sufficient attention.
The movement act of Fetch tilting its head up and down as it calculated its path gave depth information to the navigation stack and provided some context to participants watching that something was happening, but the participants’ comments indicated that this movement act was ambiguous and communicated different information. The act must communicate more explicitly that Fetch needed more time. One way to do this could be additional movements such as slowing its head movement or performing a quick “double take” when the calculation started to take more time. Another possibility could be to combine the movement with other cues such as sound and light.
Likewise, Fetch’s rotation movement act focused on the functional purpose for the navigation stack (re-calibrating its obstacles and position). On the one hand, we could have put more effort to avoid the situation entirely in the original experiment. On the other hand, this movement act could be modified to communicate its purpose to observers as an explicit, communicative motion. For example, perhaps Fetch might quickly raise and lower its torso before rotating, or it could just lower its head completely in a sign of defeat before rotating. As it is unlikely to avoid all breakdown situations, we recommend paying attention to the implicit dimension of all movement acts, including functional ones, to may make it easier to communicate a robot’s current state.
This is where knowledge from other studies may be helpful. A model for mitigating breakdowns in HRI has been proposed based on a literature review [56]. The model suggested using visual indicators (LEDs, icons, emojis), secondary screens, and audio [56], but motion is not mentioned. The responses from the participants in our case study showed that motion communicates information as well. So, incorporating motion with these other modalities could strengthen communication for mitigating a breakdown. But, as Aéraïz-Bekkis et al. already reported, the discomfort that some participants expressed can be related to uncertainty about the robot’s movements and its intentions [32]. Hence, if a robot’s unexpected movement behaviors are causing discomfort (or fear), trust in the robot might be eroded, as the robot’s performance is a large factor affecting trust [37]. This is congruent with the observation by Ogreten et al. [31] that soldiers never used a specific kind of robot in the field due to this robot’s unexpected movements. Isolating the movement into movement acts can help identify where and why the uncertainty is happening and provide places where additional or different motion may communicate more explicitly and remove the uncertainty.
Returning to the humans’ expectations of a robot based on the robot’s appearance [57], designing a movement act to express the navigation issue may help calibrate people’s expectation that the robot may not be an expert navigator yet. Similarly, using movement acts to isolate the motion in a breakdown situation could lead to more legible motion for people to understand what is happening in the situation [58]. Using movement acts may also show that there is a need to add additional functionality to the robot (e.g., adding sound, lights, or extra moving parts) to aid in legibility or provide multiple modalities for communication.
There are many areas designers can turn to for inspiration to explicitly or implicitly communicate information through motion. Some sources of inspiration can be from animals or art. For example, Koay et al. [59] looked at how hearing dogs use movement to communicate with their deaf owners and transferred it to a humanoid robot. Participants were able to understand the robot’s movement as communication and act upon them to solve a problem even when they had not been told the nature of the study. The original experiment drew inspiration from animation [28], but other areas such as puppetry [60] or dance [61] also offer inspiration. All these fields have dealt with issues of designing motion that can be understood by others, provide some expression, and set expectations by the people viewing the motion.
6.4 Making unexpected breakdowns expected
There are multiple ways to reflect on the case study. The case study might be seen as a cautionary tale. Researchers can try to control as much of the variables in an experiment, but issues still can show up. In this case, the robot may have built-in behavior that will take over if things don’t work. It is good that a built-in behavior can resolve a problem, but one should consider how the people interacting with a robot will interpret the behavior. One might conclude that researchers should prioritize making robot robust, making the experiment meticulously planned, or controlling the entire experience by filming it and having participants watch it.
We would instead present this as a call to embrace the unexpected and design the breakdown situation into a study. Using the metaphor from Hoffman and Ju’s designing with movement in mind [30], we would encourage researchers to design their experiments with the possibility of “robot breakdowns in mind.” This does not absolve researchers and engineers from designing robust robots and well-designed experiments, but to accept that a breakdown may occur and have a plan to get data out of those situations. Moreover, we want to encourage authors to extensively report unexpected breakdowns to gain a deeper understanding of HRI.
Since these breakdowns may not happen for every encounter in a study, researchers will likely need to employ qualitative methods to explore the breakdown. One way of doing this could be to have a qualitative, semi-structured interview with the participants if a breakdown situation and see how they interpreted the breakdown or even if they noticed any sort of breakdown. This may mean that even if the participants’ quantitative data may not be useful due to a breakdown, they can still provide qualitative information about their experiences and interpretations of the breakdown situation.
If experimenters desire more control and consistent experience, they could intentionally insert or trigger a breakdown situation during an experiment, even if the experiment does not primarily look at breakdowns. Since the breakdown is known in these cases, experimenters could design better ways of gathering data from the participants about the breakdown and how the participants interpret it. An inspiration for this approach comes from a long-term case study where participants developed their mental models of a robot shoe rack over several encounters with the robot changing behaviors every 2 weeks (with some unintentional errors from the Wizard) [62].
For example, if we had designed our experiment from Section 4 with breakdowns in mind, we could have used the opportunity to go deeper on things participants wrote and explored their opinions. It might have been possible to examine what participants meant when they said the robot was “waiting” or was “confused”? What actions from the robot made them think this? What made them feel uncomfortable and why? Alternatively, if the person felt that everything worked fine, why do they think that? Yet another approach could have been explored in the built-in navigation recovery. We could have found a reliable way to trigger the error to make the breakdown part of the experiment.
Answers to the qualitative questions may not be directly connected to the quantitative question being investigated in a study (our case study was not linked to the earlier experiment). The data collected from the interview questions, however, can provide a better understanding in future robot design and interaction. This could lead to insight into how to make breakdown situations easier to understand, or make people feel safer and more comfortable when such a situation occurs.
Of course, quantitative scales may also be useful for getting data about breakdowns. In the original experiment, there may have been an issue that the Godspeed Series might not have been sensitive enough to capture the change in perception during the breakdown situations. A different scale, such as the robot social attributes scale (RoSAS) [63], might have picked up participants’ different perceptions of the robot that occurred during the breakdown situation.
Designing with breakdowns in mind could be formalized so that it can be part of any HRI experiment. During an experiment’s design phase, experimenters could dedicate time to imagining possible breakdowns or other things that could go wrong. Additional sources for inspiration could include breakdowns that occurred in other pilot studies or experiments. From this work, the list of breakdowns would provide a starting point to determine what breakdowns could be prevented. For the breakdowns that are not prevented – either because they are unpreventable or they can be triggered in a controlled way – the experimenters could then plan qualitative or quantitative measures to record participants’ reactions. This results in a set of breakdowns that the experimenters can prevent; a set of breakdowns that are not preventable, but expected; and a set of breakdowns the experimenters could choose to trigger. These sets will never be complete, but the steps in creating them provide preparation to handle the unexpected breakdowns not in the sets as well. Additionally, following these steps will provide a better simulation of real world conditions and could give participants a feeling that the experiment is realistic and not contrived, regardless of whether the experiment takes place in the field or in a lab.
Breakdowns happen in many situations, inside and outside of HRI. It benefits all researchers to gather data from breakdowns to help improve future experiments and to understand how HRI can help improve a breakdown situation. Being willing to collect data from random, but expected, breakdowns in an experiment are also compatible with calls for bold HRI research [64] and to try research that goes beyond experimental psychology [65]. We can expect that as robots spend more time in less well-controlled environments, it will be necessary to also understand the extreme cases when interactions do not go as planned and researchers armed with methods to examine this area will find rich data that will improve future breakdown situations and HRI.
6.5 Limitations
We mentioned in Section 4 that we chose a within-subject design. This decision could have affected the quantitative results, for example there could be a learning effect throughout iterations. Counter-balancing can help mitigate this effect, but it is difficult to say if it had an effect here. We also mentioned that a choice of within-subjects is less important for the case study as we are interested in participants’ opinions during the breakdown situation and not the answers to the Godspeed questionnaire.
Regardless, even though all participants had a different experience with the breakdown situation, there still may be some learning effect for some participants who witnessed the breakdown situation more than once. This is where using the qualitative data in the case study is useful as the goal here is not to generalize, but to examine a phenomenon and learn from it to create better future interactions.
Having the kind of data set we had, our analysis could have benefited from using a framework such as the Linguistic Category Model [54], and would have strengthened this study. It would allow us to conduct an analysis in which the descriptive action verbs used by participants to describe the robot were examined.
One could also argue that the participants from the studies about breakdown situations presented earlier also were not aware of the planned malfunctions. Those studies are looking at results that they can generalize. Our goal here was to show that even when things in an experiment are unexpected for the researchers and the participants, there are still possibilities to get data out the situation that may be useful. Here we were limited to one qualitative question that did not specifically consider the breakdown situation, but the themes from the comments led us to develop a concept for better analyzing motion and understanding how the motion communicates information to humans.
Breakdowns are often an opportunity to return to the study design. If breakdowns have a fair enough chance of happening (e.g., one could argue 9% is fairly often in our case), it might be a good idea to spend time incorporating the breakdown into the study.
7 Conclusion
In an experiment that was designed to look at how a robot moves, we ended up with an unplanned phenomenon of a robot’s recovery procedure although we had not designed the experiment to investigate this phenomenon. Given the serendipity of the situation, we used comments from participants to examine the phenomenon and found themes related to the implicit interaction of the robot’s delays and the recovery procedure.
The themes reiterate that the robot’s movement or lack thereof can be seen as a communicative act (i.e., a movement act). A movement act will be interpreted differently by people interacting with the robot. The robot’s movement act, if it creates confusion or uncertainty, can possibly lead to humans losing trust in the robot (e.g., [31,37]). But splitting a robot’s movement into movement acts lets designers focus on the implicit and explicit communication and the act and create better communication.
Robot designers should consider that a robot’s movement in a breakdown situation may cause an observer to be confused and try to interpret what it is doing. The movement act concept allows us to isolate the motion and examine the implicit and explicit information that is communicated by the motion. By focusing on what the motion communicates, it is possible to make the message clearer to participants and observers. Providing expressive signals, perhaps by using techniques from animation, may make the robot’s movement easier to understand and thereby raise the human users’ trust in the robot.
In addition, this study shows that there is additional information that can be extracted from experiments that may not have been originally under investigation. It is still important to strive for error-free operation, but there are things that may be examined even when breakdowns happen with a robot’s performance. Breakdowns may also have consequences on how well a robot is able to learn or cooperate with a participant [66]. This points to additional considerations when designing a study to better capture unplanned situations that occur and still find interesting data from a study instance that might have otherwise been ignored in the search of answering different research question.
Breakdown situations have the potential to overshadow other effects that might have come up during the experiments otherwise. One way to eliminate these situations is more extensive pre-testing. But even when they occur, better post-experiment analysis and reporting of such occurrences can lead to better HRI research. We certainly plan on using the movement act concept and to gather and report data from breakdown situations in our future experiments involving robot movement.
Acknowledgments
The authors thank Robot House at the University of Hertfordshire for allowing us to use their facilities to design and run the experiment described in this paper. Thanks also to the interesting discussions and feedback from the SCRITA workshop at RO-MAN 2019 and the reviewers and colleagues who have read revisions of the article and provided insightful feedback. Finally, thanks to Wolfgang Leister who lent us his proofreading skills on later revisions.
-
Funding information: This research was partly funded by the Research Council of Norway as part of the Multimodal Elderly Care Systems (MECS) project, under grant agreement 247697.
-
Conflict of interest: Authors state no conflict of interest.
-
Data availability statement: The datasets generated during and/or analyzed during the current study are available from the corresponding author on reasonable request.
References
[1] T. Schulz , P. Holthaus , F. Amirabdollahian , K. L. Koay , J. Torresen , and J. Herstad , “Differences of human perceptions of a robot moving using linear or slow in, slow out velocity profiles when performing a cleaning task,” in 2019 28th International Conference on Robot and Human Interactive Communication (RO-MAN), IEEE, New Dehli, India, 2019. 10.1109/RO-MAN46459.2019.8956355Search in Google Scholar
[2] J. D. Lee and K. A. See , “Trust in automation: designing for appropriate reliance,” Hum. Factors, vol. 46, no. 1, pp. 50–80, 2004. 10.1518/hfes.46.1.50_30392Search in Google Scholar PubMed
[3] S. K. Devitt , “Trustworthiness of autonomous systems,” in Foundations of Trusted Autonomy, Studies in Systems, Decision and Control, H. A. Abbass , J. Scholz , and D. J. Reid , Eds., Cham: Springer International Publishing, 2018, pp. 161–184. 10.1007/978-3-319-64816-3_9Search in Google Scholar
[4] C. L. Bethel , Z. Henkel , and K. Baugus , “Conducting studies in human–robot interaction,” in Human–Robot Interaction: Evaluation Methods and Their Standardization, Springer Series on Bio- and Neurosystems, C. Jost , B. Le Pévédic , T. Belpaeme , C. Bethel , D. Chrysostomou , N. Crook , et al., Eds., Cham: Springer International Publishing, 2020, pp. 91–124. 10.1007/978-3-030-42307-0_4Search in Google Scholar
[5] T. B. Sheridan , “Eight ultimate challenges of human–robot communication,” in International Workshop on Robot and Human Communication, IEEE, 1997, pp. 9–14. 10.1109/ROMAN.1997.646944Search in Google Scholar
[6] M. A. Goodrich and A. C. Schultz , “Human–robot interaction: a survey,” HCI, vol. 1, no. 3, pp. 203–275, 2008. 10.1561/9781601980939Search in Google Scholar
[7] S. Lackey , D. Barber , L. Reinerman , N. I. Badler , and I. Hudson , “Defining next-generation multi-modal communication in human robot interaction,” Proc. Hum. Factors Ergon. Soc. Annu. Meet., vol. 55, no. 1, pp. 461–464, 2011. 10.1037/e578902012-095Search in Google Scholar
[8] F. Hegel , S. Gieselmann , A. Peters , P. Holthaus , and B. Wrede , “Towards a typology of meaningful signals and cues in social robotics,” in 2011 20th IEEE International Symposium on Robot and Human Interactive Communication (RO-MAN), 2011, pp. 72–78 10.1109/ROMAN.2011.6005246Search in Google Scholar
[9] M. Green , “Speech acts,” in The Stanford Encyclopedia of Philosophy, E. N. Zalta , Ed., Stanford, California, USA: Metaphysics Research Lab, Stanford University, 2017.10.1093/obo/9780195396577-0300Search in Google Scholar
[10] P. Watzlawick , J. B. Bavelas , and D. D. Jackson , Pragmatics of Human Communication: A Study of Interactional Patterns, Pathologies and Paradoxes, New York, New York, USA: W. W. Norton and Company, 1967. Search in Google Scholar
[11] J. Maynard Smith and D. Harper , Animal Signals, Oxford, England: Oxford University Press, 2003. Search in Google Scholar
[12] R. Rommetveit , “On ‘Meanings’ of acts and what is meant and made known by what is said in a pluralistic social world,” in The Structure of Action, M. Brenner , Ed., Oxford: Blackwell, 1980, pp. 108–149. Search in Google Scholar
[13] F. Heider and M. Simmel , “An experimental study of apparent behavior,” Am. J. Psychol., vol. 57, no. 2, pp. 243–259, 1944. 10.2307/1416950Search in Google Scholar
[14] J. Seibt , C. Vestergaard , and M. F. Damholdt , “Sociomorphing, not anthropomorphizing: towards a typology of experienced sociality,” in Frontiers in Artificial Intelligence and Applications, M. Nørskov , J. Seibt , and O. Quick , Eds., Vienna, Austria: IOS Press, 2020. 10.3233/FAIA200900Search in Google Scholar
[15] A. Atkin , “Peirce’s theory of signs,” in The Stanford Encyclopedia of Philosophy, E. N. Zalta , Ed., Stanford, California, USA: Metaphysics Research Lab, Stanford University, 2013.Search in Google Scholar
[16] M. Q. Patton , “Qualitative research & evaluation methods: integrating theory and practice,” Thousands Oaks, California, USA: SAGE Publications, Inc., 2015, 4th edition. Search in Google Scholar
[17] J. Seibt , “Classifying forms and modes of co-working in the ontology of asymmetric social interactions (OASIS),” in Frontiers in Artificial Intelligence and Applications, M. Coeckelbergh , J. Loh , M. Funk , J. Seibt , and M. Nørskov , Eds., Vienna, Austria: IOS Press, 2018. Search in Google Scholar
[18] C. Breazeal , C. D. Kidd , A. L. Thomaz , G. Hoffman , and M. Berlin , “Effects of nonverbal communication on efficiency and robustness in human-robot teamwork,” in International Conference on Intelligent Robots and Systems, IEEE, 2005, pp. 708–713. 10.1109/IROS.2005.1545011Search in Google Scholar
[19] A. Esposito and L. C. Jain , “Modeling social signals and contexts in robotic socially believable behaving systems,” in Toward Robotic Socially Believable Behaving Systems – Volume II, A. Esposito and L. C. Jain , Eds., vol. 106, Cham: Springer International Publishing, 2016, pp. 5–11. 10.1007/978-3-319-31053-4_2Search in Google Scholar
[20] R. A. Knepper , C. I. Mavrogiannis , J. Proft , and C. Liang , “Implicit communication in a joint action,” in Proceedings of the 2017 ACM/IEEE International Conference on Human–Robot Interaction, HRI ’17, Vienna, Austria: ACM Press, 2017, pp. 283–292 10.1145/2909824.3020226Search in Google Scholar
[21] D. Moore and W. Ju , “Sound as implicit influence on human–robot interactions,” in Companion of the 2018 ACM/IEEE International Conference on Human–Robot Interaction, HRI ’18, Chicago, IL, USA: ACM Press, 2018, pp. 311–312. 10.1145/3173386.3176918Search in Google Scholar
[22] D. Sirkin , B. Mok , S. Yang , and W. Ju , “Mechanical Ottoman: how robotic furniture offers and withdraws support,” in Proceedings of the Tenth Annual ACM/IEEE International Conference on Human–Robot Interaction, HRI ’15, Portland, Oregon, USA: ACM Press, 2015, pp. 11–18. 10.1145/2696454.2696461Search in Google Scholar
[23] P. Holthaus and S. Wachsmuth , “It was a pleasure meeting you – towards a holistic model of Human–Robot encounters,” Int. J. Soc. Robot., 2021. 10.1007/s12369-021-00759-9Search in Google Scholar
[24] B. Mutlu , “Designing embodied cues for dialog with robots,” AI Magazine, vol. 32, no. 4, pp. 17–30, 2011. 10.1609/aimag.v32i4.2376Search in Google Scholar
[25] C. I. Mavrogiannis , W. B. Thomason , and R. A. Knepper , “Social momentum: a framework for legible navigation in dynamic multi-agent environments,” in Proceedings of the 2018 ACM/IEEE International Conference on Human–Robot Interaction, HRI ’18, Chicago, IL, USA: ACM Press, 2018, pp. 361–369. 10.1145/3171221.3171255Search in Google Scholar
[26] A. Zhou , D. Hadfield-Menell , A. Nagabandi , and A. D. Dragan , “Expressive robot motion timing,” in Proceedings of the 2017 ACM/IEEE International Conference on Human–Robot Interaction, HRI ’17, Vienna, Austria: ACM, 2017, pp. 22–31. 10.1145/2909824.3020221Search in Google Scholar
[27] F. Thomas and O. Johnston , The Illusion of Life: Disney Animation, New York: Hyperion, 1995, 1st hyperion edition. Search in Google Scholar
[28] T. Schulz , J. Torresen , and J. Herstad , “Animation techniques in human–robot interaction user studies: a systematic literature review,” ACM Trans. Hum.-Robot. Interact., vol. 8, no. 2, art. 12, 2019. 10.1145/3317325Search in Google Scholar
[29] A. D. Dragan , S. Bauman , J. Forlizzi , and S. S. Srinivasa , “Effects of robot motion on human–robot collaboration,” in Proceedings of the Tenth Annual ACM/IEEE International Conference on Human–Robot Interaction, HRI ’15, New York, NY, USA: ACM, 2015, pp. 51–58. 10.1145/2696454.2696473Search in Google Scholar
[30] G. Hoffman and W. Ju , “Designing robots with movement in mind,” J. Hum.-Robot Interact., vol. 3, no. 1, pp. 89–122, 2014. 10.5898/JHRI.3.1.HoffmanSearch in Google Scholar
[31] S. Ogreten , S. Lackey , and D. Nicholson , “Recommended roles for uninhabited team members within mixed-initiative combat teams,” in 2010 International Symposium on Collaborative Technologies and Systems, 2010, pp. 531–536. 10.1109/CTS.2010.5478468Search in Google Scholar
[32] D. Aéraïz-Bekkis , G. Ganesh , E. Yoshida , and N. Yamanobe , “Robot movement uncertainty determines human discomfort in co-worker scenarios,” in 2020 6th International Conference on Control, Automation and Robotics (ICCAR), 2020, pp. 59–66. 10.1109/ICCAR49639.2020.9108085Search in Google Scholar
[33] R. van den Brule , R. Dotsch , G. Bijlstra , D. Wigboldus , and P. Haselager , “Do robot performance and behavioral style affect human trust?,” Int. J. Soc. Robot., vol. 6, no. 4, pp. 519–531, 2014. 10.1007/s12369-014-0231-5Search in Google Scholar
[34] S. Lemaignan , J. Fink , F. Mondada , and P. Dillenbourg , “You’re doing it wrong! Studying unexpected behaviors in child-robot interaction,” in Social Robotics, Lecture Notes in Computer Science, A. Tapus , E. André , J.-C. Martin , F. Ferland , and M. Ammi , Eds., Cham: Springer International Publishing, 2015, pp. 390–400. 10.1007/978-3-319-25554-5_39Search in Google Scholar
[35] M. Ragni , A. Rudenko , B. Kuhnert , and K. O. Arras , “Errare humanum est: Erroneous robots in human–robot interaction,” in 2016 25th IEEE International Symposium on Robot and Human Interactive Communication (RO-MAN), 2016, pp. 501–506. 10.1109/ROMAN.2016.7745164Search in Google Scholar
[36] N. Mirnig , G. Stollnberger , M. Miksch , S. Stadler , M. Giuliani , and M. Tscheligi , “To err is robot: how humans assess and act toward an erroneous social robot,” Front. Robot. AI, vol. 4, art. 21, 2017. 10.3389/frobt.2017.00021Search in Google Scholar
[37] P. A. Hancock , D. R. Billings , K. E. Schaefer , J. Y. C. Chen , E. J. de Visser , and R. Parasuraman , “A meta-analysis of factors affecting trust in human–robot interaction,” Human Factors, vol. 53, no. 5, pp. 517–527, 2011. 10.1177/0018720811417254Search in Google Scholar PubMed
[38] M. Salem , G. Lakatos , F. Amirabdollahian , and K. Dautenhahn , “Would you trust a (faulty) robot?: effects of error, task type and personality on human–robot cooperation and trust,” in Proceedings of the Tenth Annual ACM/IEEE International Conference on Human–Robot Interaction, HRI ’15, New York, NY, USA: ACM, 2015, pp. 141–148. 10.1145/2696454.2696497Search in Google Scholar
[39] F. Correia , C. Guerra , S. Mascarenhas , F. Melo , and A. Paiva , “Exploring the impact of fault justification in human–robot trust: socially interactive agents track,” in Proceedings of the International Joint Conference on Autonomous Agents and Multiagent Systems (AAMAS), 2018, vol. 1, pp. 507–513. Search in Google Scholar
[40] M. K. Lee , S. Kielser , J. Forlizzi , S. Srinivasa , and P. Rybski , “Gracefully mitigating breakdowns in robotic services,” in Proceedings of the 5th ACM/IEEE International Conference on Human–Robot Interaction, HRI ’10, Piscataway, NJ, USA: IEEE Press, 2010, pp. 203–210. 10.1145/1734454.1734544Search in Google Scholar
[41] N. Mirnig , M. Giuliani , G. Stollnberger , S. Stadler , R. Buchner , and M. Tscheligi , “Impact of robot actions on social signals and reaction times in HRI error situations,” in Social Robotics, Lecture Notes in Computer Science, A. Tapus , E. André , J.-C. Martin , F. Ferland , and M. Ammi , Eds., Cham: Springer International Publishing, 2015, pp. 461–471. 10.1007/978-3-319-25554-5_46Search in Google Scholar
[42] E. I. Barakova , P. Bajracharya , M. Willemsen , T. Lourens , and B. Huskens , “Long-term LEGO therapy with humanoid robot for children with ASD,” Expert Sys., vol. 32, no. 6, pp. 698–709, 2015. 10.1111/exsy.12098Search in Google Scholar
[43] C. Bartneck , D. Kulić , E. Croft , and S. Zoghbi , “Measurement instruments for the anthropomorphism, animacy, likeability, perceived intelligence, and perceived safety of robots,” Int. J. Soc. Robot., vol. 1, no. 1, pp. 71–81, 2009. 10.1007/s12369-008-0001-3Search in Google Scholar
[44] M. Wise , M. Ferguson , D. King , E. Diehr , and D. Dymesich , “Fetch and freight: standard platforms for service robot applications,” in IJCAI 2016 Workshop Autonomous Mobile Service Robots, 2016. Search in Google Scholar
[45] J. Rose , D. W. Morgan , and J. G. Gamble , Energetics of walking, in Human Walking, J. Rose and J. G. Gamble , Eds., 3rd edition, Philadelphia, PA, USA: Lippincott Williams and Wilkins, 2006, pp. 77–102. Search in Google Scholar
[46] T. Schulz , J. Herstad , and J. Torresen , “Classifying human and robot movement at home and implementing robot movement using the slow in, slow out animation principle,” Int. J. Adv. Intell. Sys., vol. 11, no. 3–4, pp. 234–244, 2018. Search in Google Scholar
[47] Open Source Robotics Foundation, About ROS, 2019. Search in Google Scholar
[48] B. P. Gerkey and K. Konolige , “Planning and control in unstructured terrain,” in Workshop on Path Planning on Costmaps, Proceedings of the IEEE International Conference on Robotics and Automation (ICRA), 2008. Search in Google Scholar
[49] M. J. Gielniak , C. K. Liu , and A. Thomaz , “Stylized motion generalization through adaptation of velocity profiles,” in 2010 RO-MAN: The 19th IEEE International Symposium on Robot and Human Interactive Communication, IEEE, 2010, pp. 304–309. 10.1109/ROMAN.2010.5598733Search in Google Scholar
[50] L. D. Riek , “Wizard of Oz studies in HRI: a systematic review and new reporting guidelines,” J. Hum.-Robot. Interact., vol. 1, no. 1, pp. 119–136, 2012. 10.5898/JHRI.1.1.RiekSearch in Google Scholar
[51] M. B. Miles , A. M. Huberman , and J. Saldañs , Qualitative Data Analysis: A Methods Sourcebook, 4th edition, Los Angeles: SAGE, 2020. Search in Google Scholar
[52] S. R. Fussell , S. Kiesler , L. D. Setlock , and V. Yew , “How people anthropomorphize robots,” in: 2008 3rd ACM/IEEE International Conference on Human–Robot Interaction (HRI), 2008, pp. 145–152. 10.1145/1349822.1349842Search in Google Scholar
[53] J. Seibt , “Towards an ontology of simulated social interaction: varieties of the ‘As if’ of robots and humans,” in Sociality and Normativity for Robots, R. Hakli and J. Seibt , Eds., Cham, Germany: Springer International Publishing, 2017. 10.1007/978-3-319-53133-5_2Search in Google Scholar
[54] G. R. Semin , “The Linguistic Category Model,” in Handbook of Theories of Social Psychology: Volume 1, P. A. M. Van Lange , A. W. Kruglanski , and E. T. Higgins , Eds, Sage Publications Ltd., 2012, pp. 309–326, https://doi.org/10.4135/9781446249215.n16. 10.4135/9781446249215.n16Search in Google Scholar
[55] T. Winograd and F. Flores , Understanding Computers and Cognition: A New Foundation for Design, Norwood, New Jersey, USA: Intellect Books, 1986. Search in Google Scholar
[56] S. Honig and T. Oron-Gilad , “Understanding and resolving failures in human–robot interaction: literature review and model development,” Front. Psychol., vol. 9, art. 861, 2018. 10.3389/fpsyg.2018.00861Search in Google Scholar PubMed PubMed Central
[57] M. Kwon , M. F. Jung , and R. A. Knepper , “Human expectations of social robots,” in 2016 11th ACM/IEEE International Conference on Human–Robot Interaction (HRI), 2016, pp. 463–464. 10.1109/HRI.2016.7451807Search in Google Scholar
[58] A. D. Dragan , K. C. Lee , and S. S. Srinivasa , “Legibility and predictability of robot motion,” in Proceedings of the 8th ACM/IEEE International Conference on Human–Robot Interaction, HRI ’13, Piscataway, NJ, USA: IEEE Press, 2013, pp. 301–308. 10.1109/HRI.2013.6483603Search in Google Scholar
[59] K. L. Koay , G. Lakatos , D. S. Syrdal , M. Gácsi , B. Bereczky , K. Dautenhahn , et al., “Hey! There is someone at your door. A hearing robot using visual communication signals of hearing dogs to communicate intent,” in 2013 IEEE Symposium on Artificial Life (ALife), 2013, pp. 90–97. 10.1109/ALIFE.2013.6602436Search in Google Scholar
[60] M. Luria , G. Hoffman , B. Megidish , O. Zuckerman , and S. Park , “Designing Vyo, a robotic smart home assistant: Bridging the gap between device and social agent,” in 2016 25th IEEE International Symposium on Robot and Human Interactive Communication (RO-MAN), 2016, pp. 1019–1025. 10.1109/ROMAN.2016.7745234Search in Google Scholar
[61] A. LaViers , L. Teague , and M. Egerstedt , “Style-based robotic motion in contemporary dance performance,” in Controls and Art, Cham: Springer, 2014, pp. 205–229. 10.1007/978-3-319-03904-6_9Search in Google Scholar
[62] M. Rueben , J. Klow , M. Duer , E. Zimmerman , J. Piacentini , M. Browning , et al., “Mental models of a mobile shoe rack: exploratory findings from a long-term in-the-wild study,” ACM Trans. Hum.-Robot Interact., vol. 10, no. 2, pp. 16:1–16:36, 2021. 10.1145/3442620Search in Google Scholar
[63] C. M. Carpinella , A. B. Wyman , M. A. Perez , and S. J. Stroessner , “The robotic social attributes scale (RoSAS): development and validation,” in Proceedings of the 2017 ACM/IEEE International Conference on Human–Robot Interaction, HRI ’17 Vienna, Austria: ACM, 2017, pp. 254–262. 10.1145/2909824.3020208Search in Google Scholar
[64] R. Brooks , “A brave, creative, and happy HRI,” ACM Trans. Hum.-Robot Interact., vol. 7, no. 1, pp. 1:1–1:3, 2018. 10.1145/3209540Search in Google Scholar
[65] K. Dautenhahn , “Some brief thoughts on the past and future of human–robot interaction,” ACM Trans. Hum.-Robot Interact., vol. 7, no. 1, pp. 4:1–4:3, 2018. 10.1145/3209769Search in Google Scholar
[66] S. K. Kim , E. A. Kirchner , L. Schloßmüller , and F. Kirchner , “Errors in human–robot interactions and their effects on robot learning,” Front. Robot. AI, vol. 7, art. 558531, 2020. 10.3389/frobt.2020.558531Search in Google Scholar PubMed PubMed Central
© 2021 Trenton Schulz et al., published by De Gruyter
This work is licensed under the Creative Commons Attribution 4.0 International License.